Hybrid scheme of DFT and machine learning to accelerate the design of graphyne nanoribbons as electrocatalysts for the ORR and HER
FUEL(2024)
摘要
Recently, graphyne and its family members (GYFs) have emerged as promising carbon-based metal-free catalysts (CMFCs) for the oxygen reduction reaction (ORR) and hydrogen evolution reaction (HER). Herein, we performed density functional theory simulations to explore the ORR and HER performances of beta-graphyne/graphdiyne nanoribbons (beta GyNRs/beta GDyNRs), which have received scant attention. Our results reveal that beta GyNRs/beta GDyNRs are excellent bifunctional CMFCs after functionalization by manipulating the edge shape or N-doping. Moreover, we verified the role of the binding strength of the H atom (Delta EH*) as a universal descriptor for predicting the bifunctional activities for the ORR and HER on GYFs. We also proposed a machine learning model based on an extreme gradient boosting algorithm for predicting the bifunctional activities of GYFs. Feature importance analysis indicated that the atomic charge of the catalytic site (Q) played a determining role in the ORR activity of the GYFs. This study not only identifies promising GYFs, but also accelerates the search for highly active GYFs for the ORR and HER.
更多查看译文
关键词
Graphyne,Density functional theory,Machine learning,ORR,HER
AI 理解论文
溯源树
样例
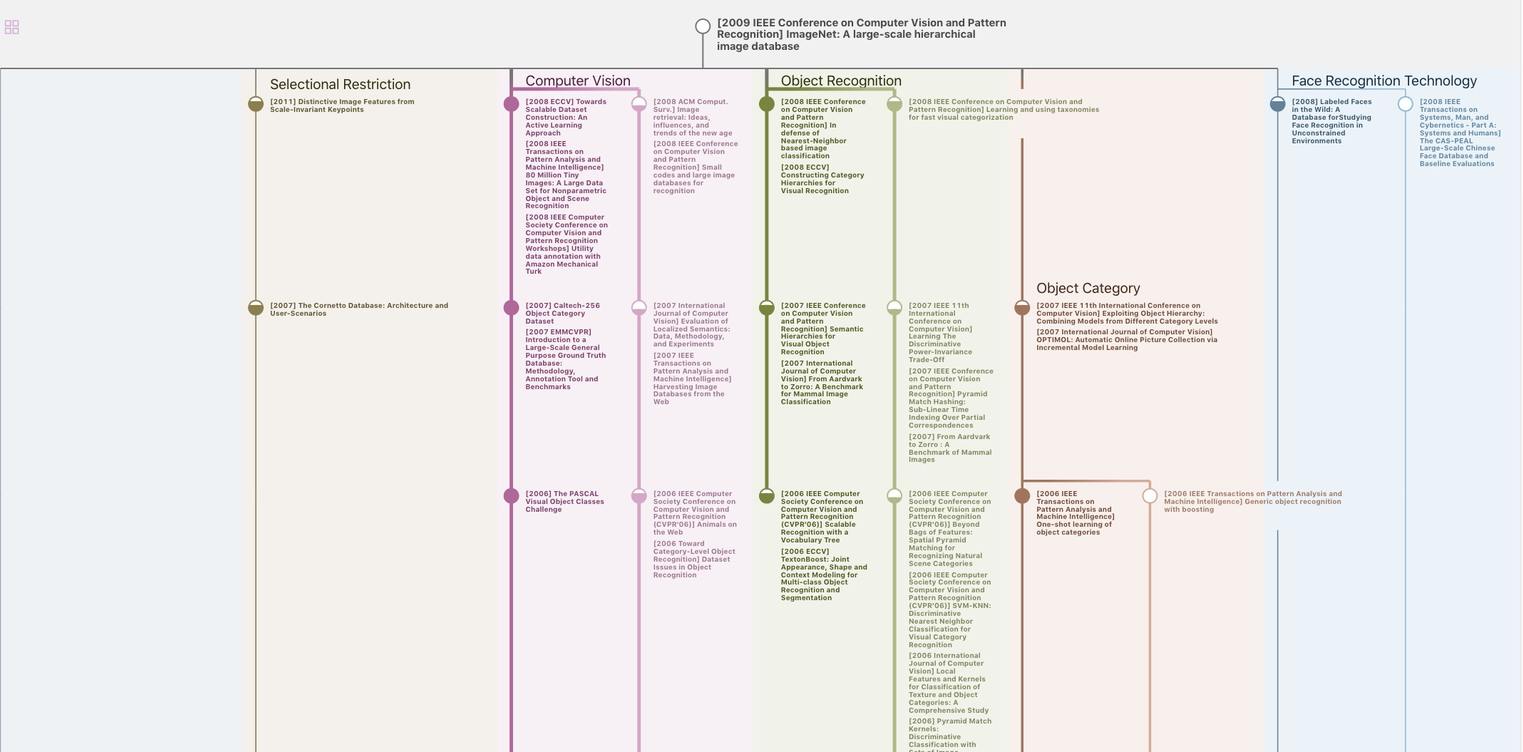
生成溯源树,研究论文发展脉络
Chat Paper
正在生成论文摘要