A dual-branch siamese spatial-spectral transformer attention network for Hyperspectral Image Change Detection
EXPERT SYSTEMS WITH APPLICATIONS(2024)
摘要
The convolutional neural networks have recently gained widespread attention in Hyperspectral Image Change Detection (HSI-CD) due to their outstanding feature extraction ability. However, limited by the inherent network backbones, the convolutional neural networks (CNNs) fail to mine the sequence attributes and model the intricate relationships of spectral signatures. In contrast, transformers are proficient at learning sequence information owing to the powerful self-attention mechanisms. The two backbone structures exhibit complementary spatial and spectral feature extraction strengths, respectively. Inspired by this, we propose a dual-branch siamese spatial-spectral transformer attention network (DBS3TAN) for HSI-CD. The main idea is to fully exploit the advantages of CNNs and transformers for spatial and spectral feature extraction. More importantly, we devise the two key modules, i.e., the spatial attention module and the spatial-spectral transformer module. The former utilizes depthwise separable convolutions and attention mechanisms to emphasize the features of dual-temporal HSIs from the spatial perspective. The latter focuses on the sequence attributes of spectral signatures and mines the spatial characteristics from adjacent pixels. We employ the weighted contrastive loss function to separate the changed and unchanged pixels more reliably and set the random weight factors to balance the contributions of the two branches. Finally, the threshold values judgment is used to obtain the ultimate detection maps. We conduct extensive experiments to evaluate the DBS3TAN on three HSI datasets, demonstrating its superior performances than compared methods qualitatively and quantitatively. The source code will be available at https://github.com/zhangyiyan001/DBS3TAN.
更多查看译文
关键词
Hyperspectral change detection,Dual-branch siamese network,Spatial-spectral transformer,Attention module
AI 理解论文
溯源树
样例
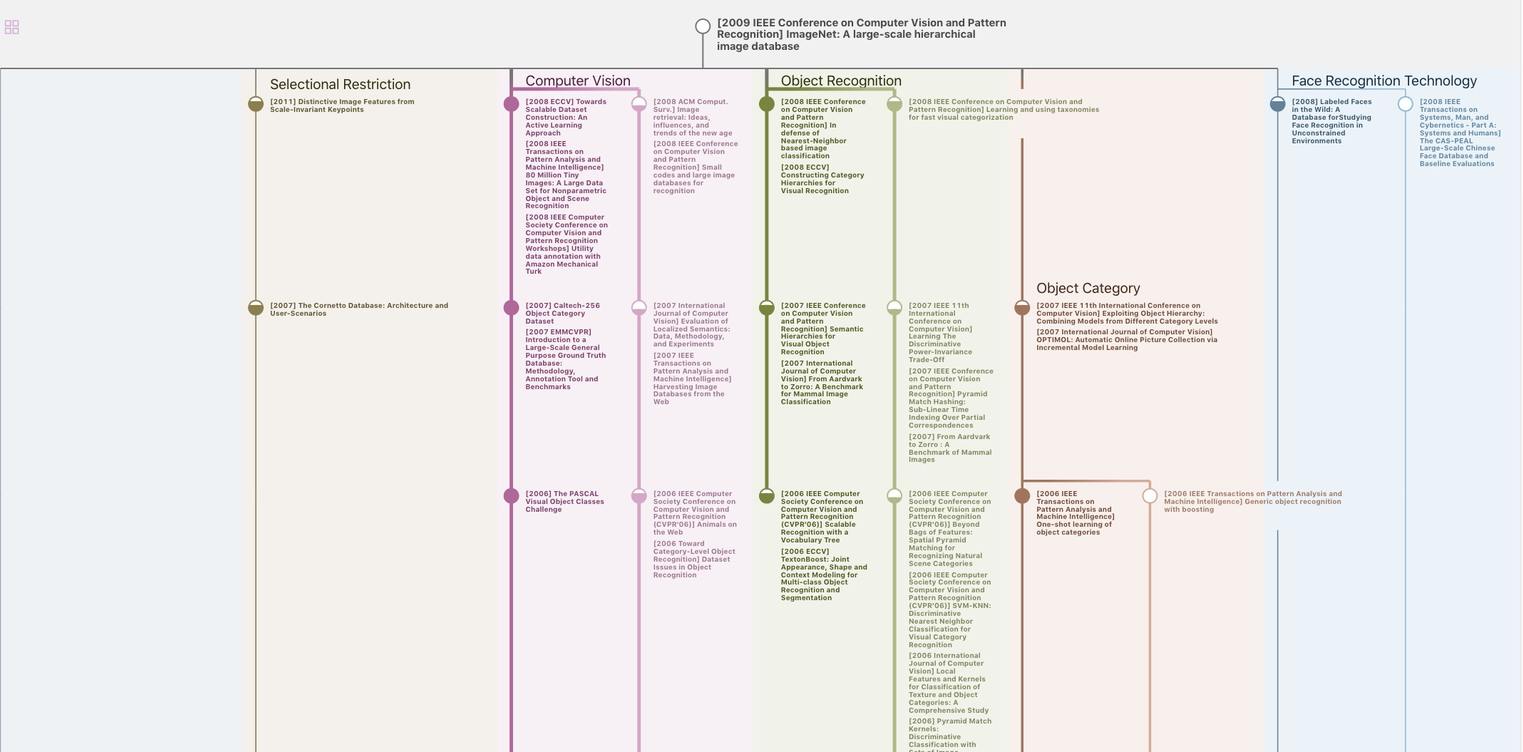
生成溯源树,研究论文发展脉络
Chat Paper
正在生成论文摘要