Taking complementary advantages: Improving exploration via double self-imitation learning in procedurally-generated environments
EXPERT SYSTEMS WITH APPLICATIONS(2024)
摘要
Efficient exploration is the core issue of deep reinforcement learning. Although state-of-the-art exploration methods have achieved much progress in many tasks, they usually underperform in procedurally-generated environments, indicating the low capability of generalization of the agent. To address the problem, a self-imitation exploration approach for procedurally-generated environments, referred to as Double Self-Imitation Learning (DSIL), is proposed. DSIL screens out good history experiences of exploration by utilizing an episode scoring rule that considers local scores, global scores and external rewards. Then DSIL employs a cooperation strategy to reproduce the agent's past good exploration behaviors by combining generative adversarial imitation learning (GAIL) and behavioral cloning (BC). Specifically, DSIL is composed of a reinforcement learning module and a discriminator. The discriminator generates intrinsic rewards by judging the similarity of the current state-action pairs to the past good exploration experiences. The policy of agent is optimized alternately by the BC task and the reinforcement learning algorithm in the GAIL task; meanwhile, the reinforcement learning module and the discriminator are updated alternately in the GAIL task. Experiments on several procedurally-generated environments demonstrated that the proposed DSIL significantly outperformed existing exploration approaches in sample efficiency and performance, that is, DSIL makes the agent have stronger generalization.
更多查看译文
关键词
Reinforcement learning,Exploration,Generalization,Intrinsic reward,Self-imitation
AI 理解论文
溯源树
样例
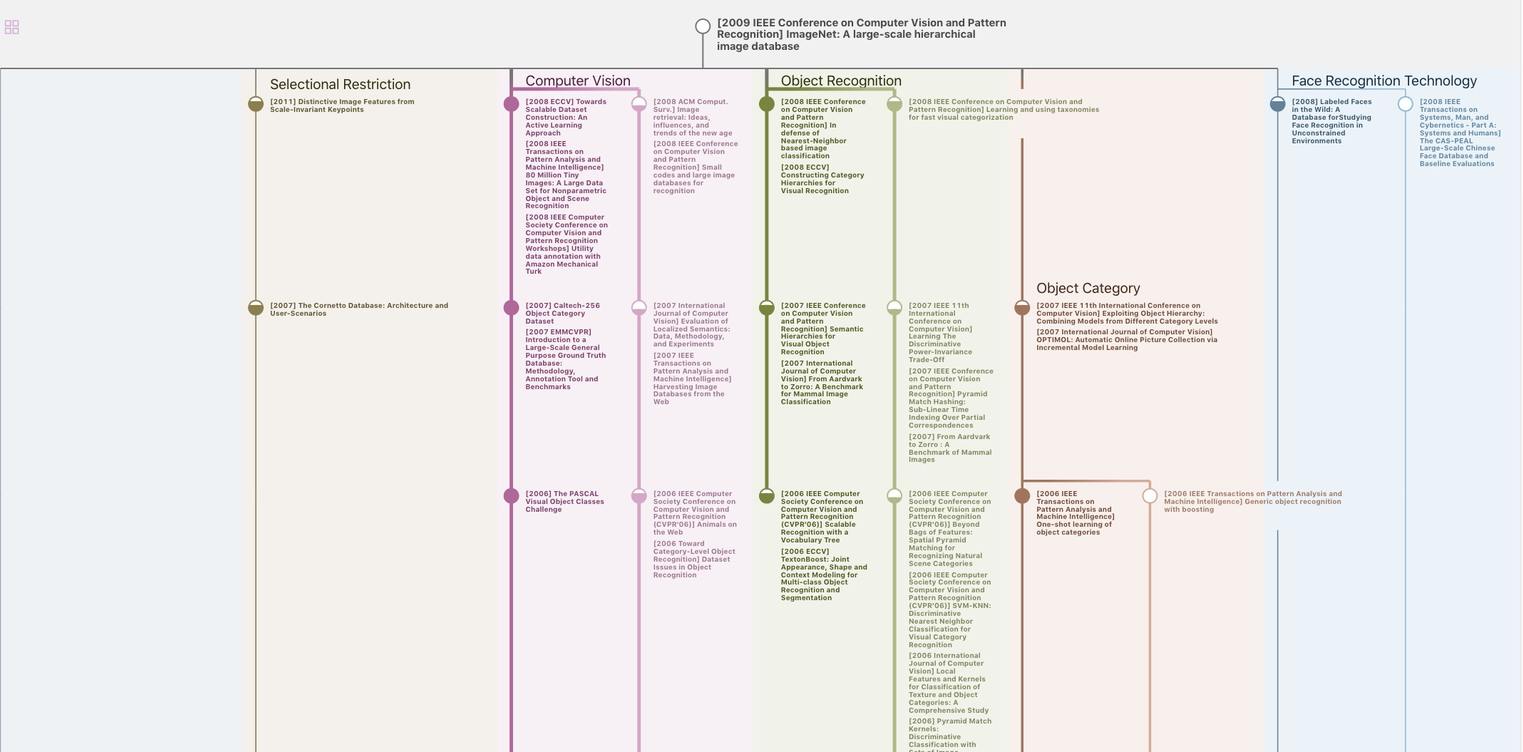
生成溯源树,研究论文发展脉络
Chat Paper
正在生成论文摘要