Considering the dual endogenous-exogenous uncertainty integrated energy multiple load short-term forecast
Energy(2023)
摘要
More accurate multivariate load forecasting is conducive to integrated energy system planning optimization and economic operation. In this paper, based on the consideration of exogenous factors such as weather, endogenous factors are added into the prediction, and a short-term prediction model for integrated energy multivariate load based on endo-exogenous uncertainty is established using fine-tuned transfer learning. The LSTM pre-training model is first initially established using genetic algorithm and exogenous factors such as weather. Then the exogenous uncertainty of the pre-training model and the knowledge of endogenous uncertainty in the target model are combined using fine-tuned transfer learning to continuously optimize the basic parameters of the prediction model to obtain the final multivariate load prediction model. The performance of the four models is comprehensively evaluated after the historical load validation of the integrated energy system in Beijing, China. Compared with the traditional LSTM multivariate load forecasting model, the MAPE of the proposed model in this paper is reduced by 21.03 %, 13.00 %, and 24.39 % for electricity, heat, and cooling load forecasting, respectively.
更多查看译文
关键词
Integrated energy system,Multiple load forecasting,Endogenous-exogenous uncertainty,Long- and short-term memory networks,Transfer learning
AI 理解论文
溯源树
样例
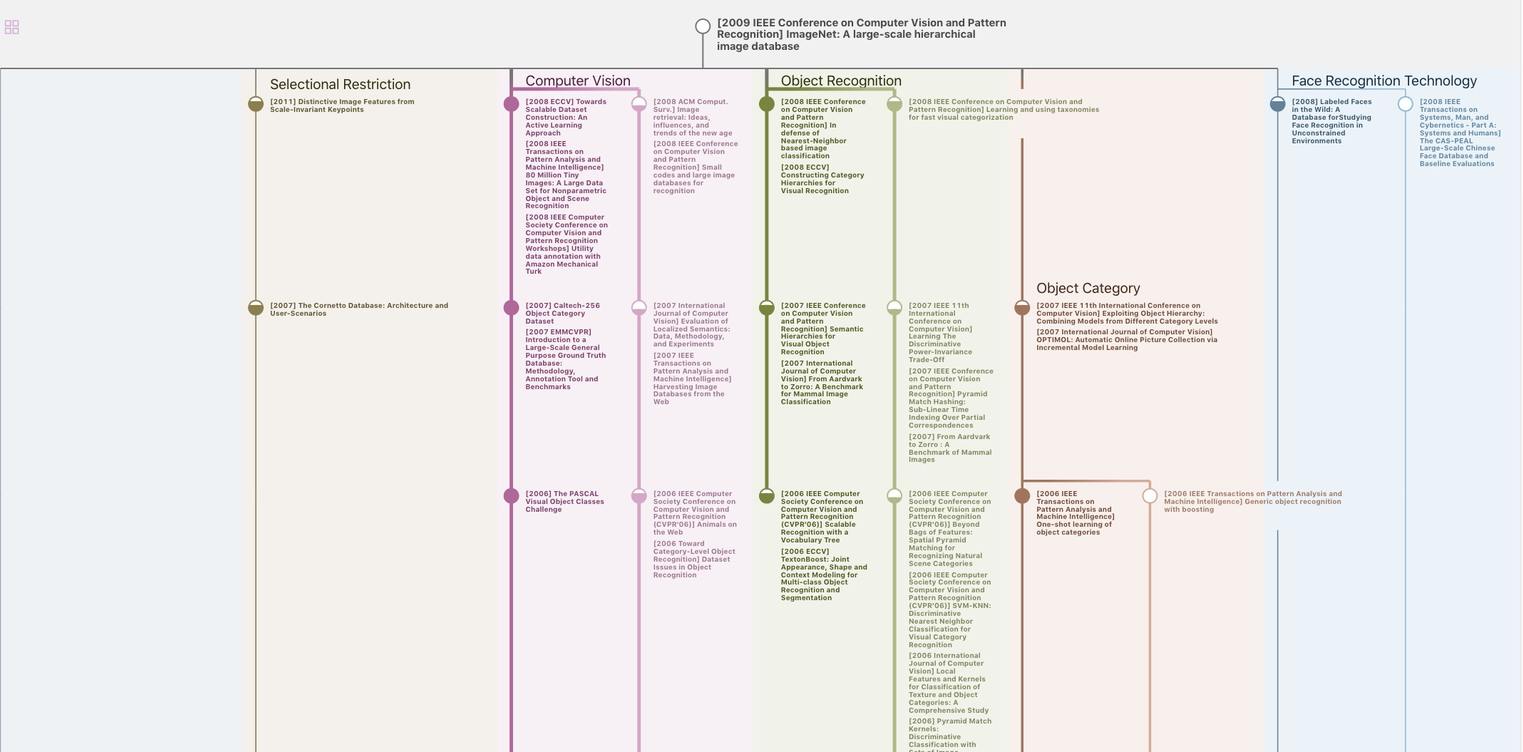
生成溯源树,研究论文发展脉络
Chat Paper
正在生成论文摘要