An empirical evaluation of Q-learning in autonomous mobile robots in static and dynamic environments using simulation
Decision Analytics Journal(2023)
摘要
Path planning plays a crucial role in the navigation of mobile robots. Among the various path planning techniques, Q-learning (QL) has gained popularity as a reinforcement learning approach that exhibits the ability to learn without significant prior knowledge of the environment. However, despite the introduction of enhanced versions of Q-learning, specifically distance metric and moving target Q-learning (DMMTQL) and distortion and optimization Q-learning (DOQL), the validation of these algorithms in real-world scenarios is incomplete. In this study, we conduct real-world experiments to assess the performance of DMMTQL and DOQL in two distinct environments: one featuring static and another with dynamic obstacles. Our investigation involves comparing the real-world results obtained from DMMTQL and DOQL with those obtained from QL and contrasting them with simulation results. The findings from our real-world experiments demonstrate that both DMMTQL and DOQL outperform QL in terms of path planning effectiveness. Both improved QL algorithms are able to analyse and decide the optimal path with free collision for the mobile robots. When comparing the improvements achieved by DMMTQL and DOQL with simulation results, we observe similar outcomes in most aspects, with the exception of the time taken and distance travelled metrics.
更多查看译文
关键词
Autonomous mobile robot,Real world experiment,Static environment,Dynamic environment,Path planning,Q-learning
AI 理解论文
溯源树
样例
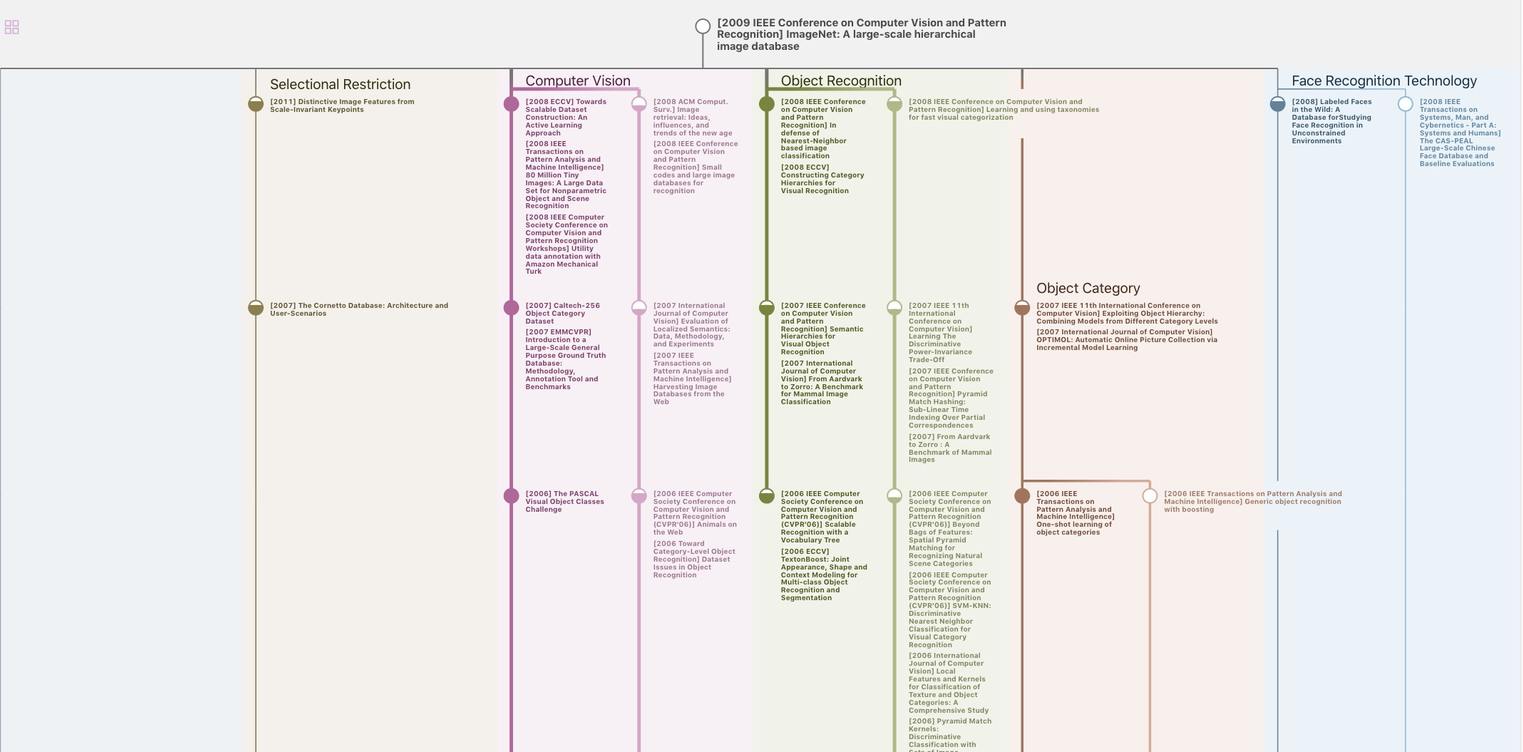
生成溯源树,研究论文发展脉络
Chat Paper
正在生成论文摘要