Winter wheat yield prediction using integrated Landsat 8 and Sentinel-2 vegetation index time-series data and machine learning algorithms
Computers and Electronics in Agriculture(2023)
摘要
Timely and accurate forecasting of winter wheat yield is important to crop management, food security, and sustainable agricultural development. Unfortunately, the process of predicting winter wheat yield using satellite time series data often fails to capture complete and critical information about the crop growth process, which can restrict the accuracy of crop yield predictions. To overcome this challenge, it is necessary to increase the frequency of monitoring crop growth dynamics, identify suitable vegetation index (VI), and determine the optimal prediction model for time-series remote sensing data. In this study, we propose proposes a novel method for predicting winter wheat yield using integrated Landsat 8 (L8) and Sentinel-2 (S2) vegetation index time-series data and machine learning algorithms. Firstly, the integrated L8 and S2 dataset was obtained through the steps of cloud masking, re-sampling, re-projection, BRDF correction, and band adjustment. Then, the optimal VI was determined based on the association between the growth characteristics of winter wheat and the time-series characteristic curves of each VI. Subsequently, we employed Bayesian optimized CatBoost (BO-CatBoost) regression model to predict winter wheat yield, and compared this method with three other data-driven methods, including least absolute shrinkage and selection operator (LASSO), support vector regression (SVM), and random forest (RF). Our results showed that the winter wheat yield prediction accuracies reached the best performance using integrated Landsat 8 and Sentinel-2 WDRVI (Wide Dynamic Range Vegetation Index) time-series data and BO-CatBoost model. The R2 values were 0.70, 0.63, and 0.68, and RMSE values were 0.62, 0.73, and 0.62 t/ha for the years 2019 to 2021, respectively. In addition, acceptable accuracy was obtained for yield prediction in 2021 based on the model trained with historical data from 2019 and 2020. Moreover, this study demonstrated the result that winter wheat yield could be predicted about 40 days earlier using the proposed method. Finally, results showed that the harmonized data improved the yield estimation accuracies by a factor of 1.52, 1.29, and 1.13 compared with single S2 dataset for years 2019-2021. Various experiments demonstrated that the proposed method could effectively estimate and predict winter wheat yield data with good accuracy and robustness. This study provides technical support for improving the accuracy of winter wheat yield prediction and has the potential to be extended to yield estimation for other crops.
更多查看译文
关键词
Integrated data,Yield prediction,Time series,Wide Dynamic Range Vegetation Index,CatBoost model
AI 理解论文
溯源树
样例
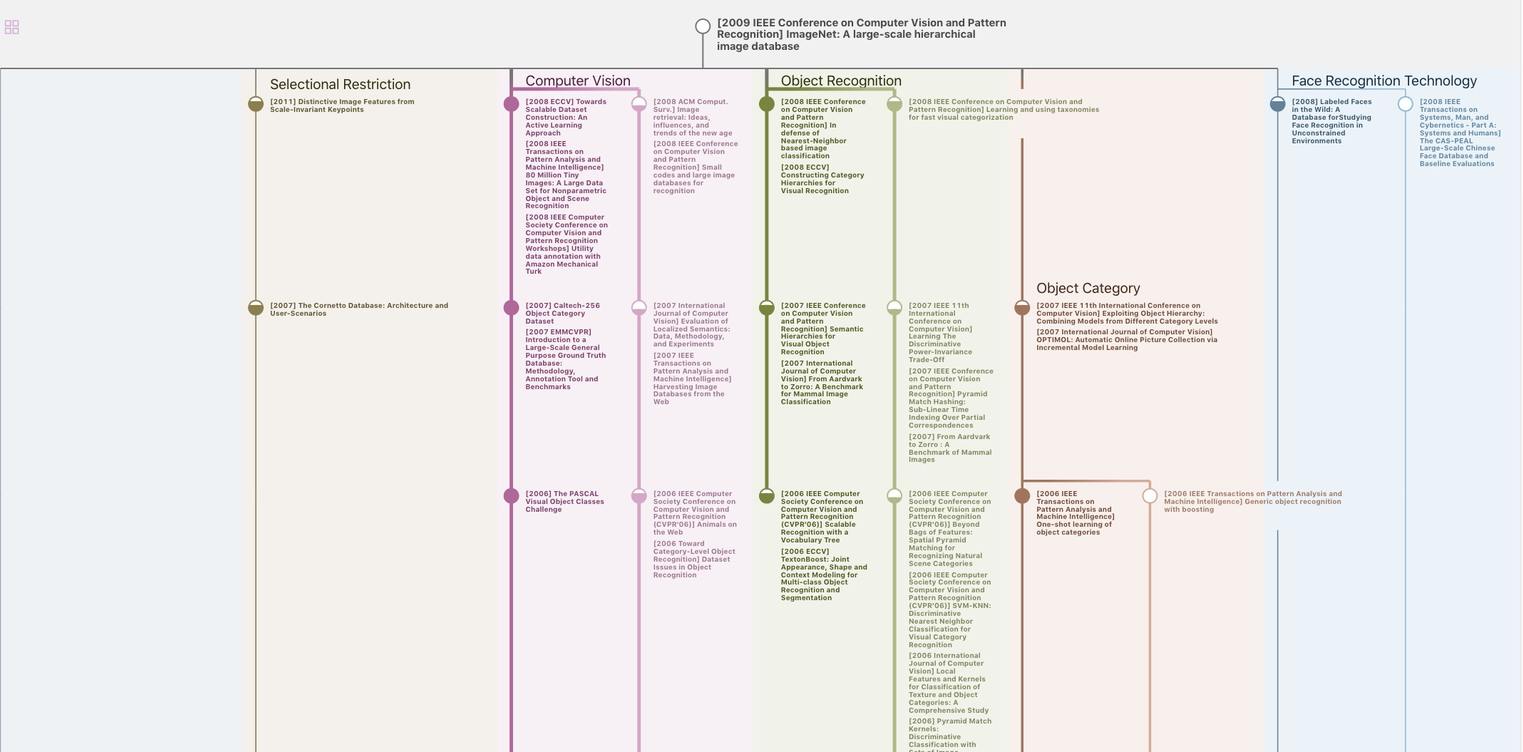
生成溯源树,研究论文发展脉络
Chat Paper
正在生成论文摘要