Investigation on the combined model of sensor drift compensation and open-set gas recognition based on electronic nose datasets
Chemometrics and Intelligent Laboratory Systems(2023)
摘要
Electronic nose (EN) is widely used to identify and detect gases. However, ENs are inevitably subject to sensor drift and the intrusion of unknown gases during prolonged exposure to the environment. A combined model of sensor drift compensation and open-set gas recognition, class anchoring and contrasting semantic alignment (CACSA) was proposed and tested on two commonly used EN drift datasets. Firstly, the model learns an embedding subspace that is discriminative, where mapped domains are semantically aligned and maximally separated. In addition, the method requires a very small number of labeled target training samples, even if one per class can be effective. Then, our model learns a fixed anchor for each known class. Known classes form tight clusters around the centers of class dependencies anchored in the feature space. CACSA can be degenerated into a closed-set drift compensation model without sacrificing accuracy and not require the additional target domain sample labeling and repeated training for open-set recognition. According to the experimental result, our model showed better drift compensation performance, and achieved the highest open-set recognition accuracy.
更多查看译文
关键词
gas recognition,sensor drift compensation,open-set
AI 理解论文
溯源树
样例
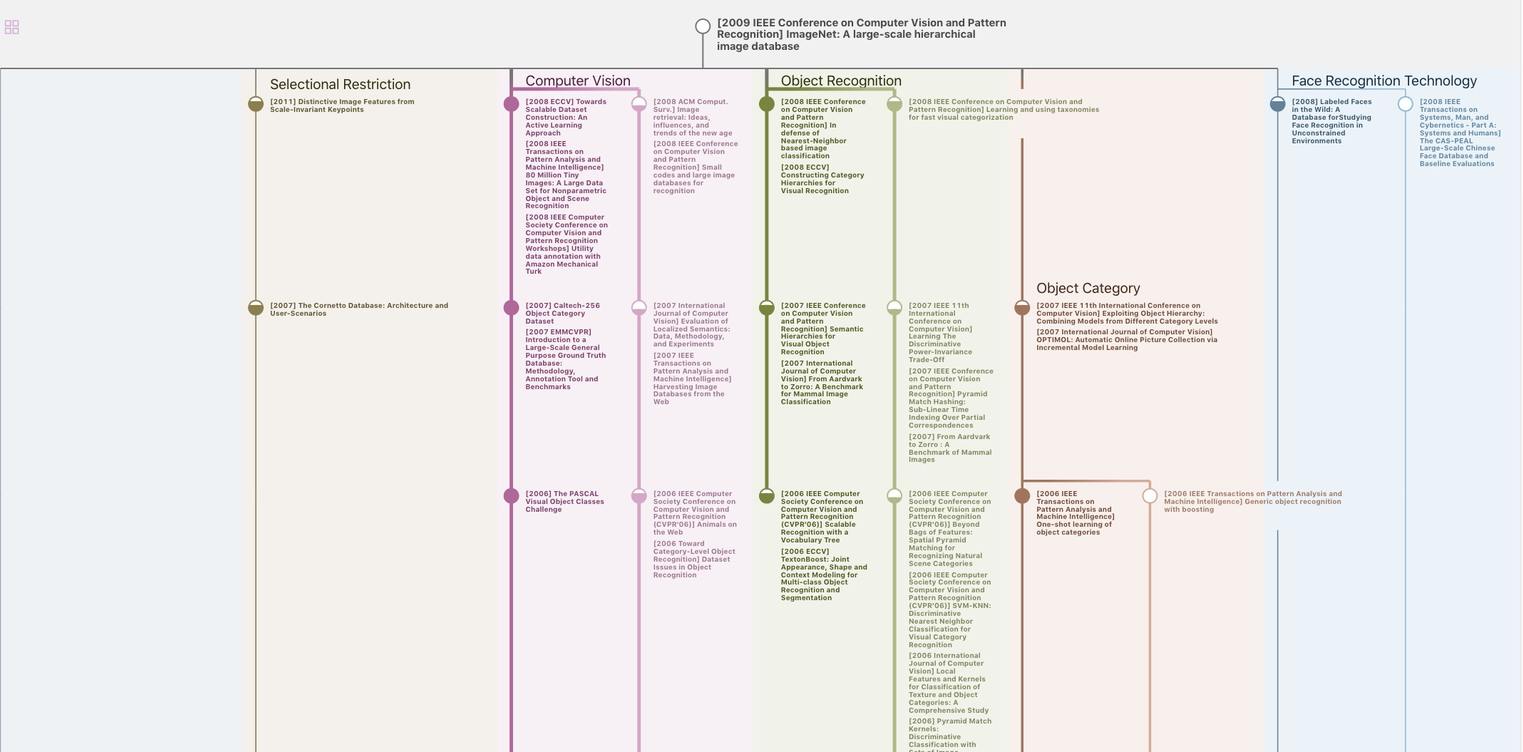
生成溯源树,研究论文发展脉络
Chat Paper
正在生成论文摘要