PulseID: Multi-scale photoplethysmographic identification using a deep convolutional neural network
BIOMEDICAL SIGNAL PROCESSING AND CONTROL(2024)
摘要
Photoplethysmography (PPG) signals reflect the cardiovascular health of the human body and contain discriminate information that can be utilized as biometric traits in the personal human identification field. Recent approaches achieve it by hand-crafted features and using shallow networks to extract features, which might not achieve comparable results on large-scale datasets. In this paper, we first propose PPG augmentation to generate multi-scale PPG signals to avoid overfitting. Subsequently, a deep end-to-end model, namely PulseID, is proposed to extract multi-scale and unique features from PPG signals. Finally, a curriculum learning guided training strategy is proposed to provide elaborate supervisions for PulseID. As key modules in curriculum learning, contrastive learning is adopted to enhance the discriminate distribution in feature space. Comprehensive experiments are demonstrated on 4 databases which are ZJPhys, Capno, Bidmc, and Biosec2 respectively to show our superior performance on both intra-and cross-dataset testings. Specifically, on intra-dataset testing, the proposed PulseID achieves superior performance on the largest database compared with state-of-the-art methods which are 94.3% and 2.9% in terms of Average Accuracy and Equal Error Rate. On cross-dataset testing, PulseID also achieves comparable performance on unknown individual recognition regardless of various sampling rates, races, and body conditions. In conclusion, without heavy pre-processing, the proposed PulseID can be easily used in known-and unknown-individual recognition with superior performance.
更多查看译文
关键词
Photoplethysmography,Person identification,Convolutional neural network,Curriculum learning,Contrastive learning
AI 理解论文
溯源树
样例
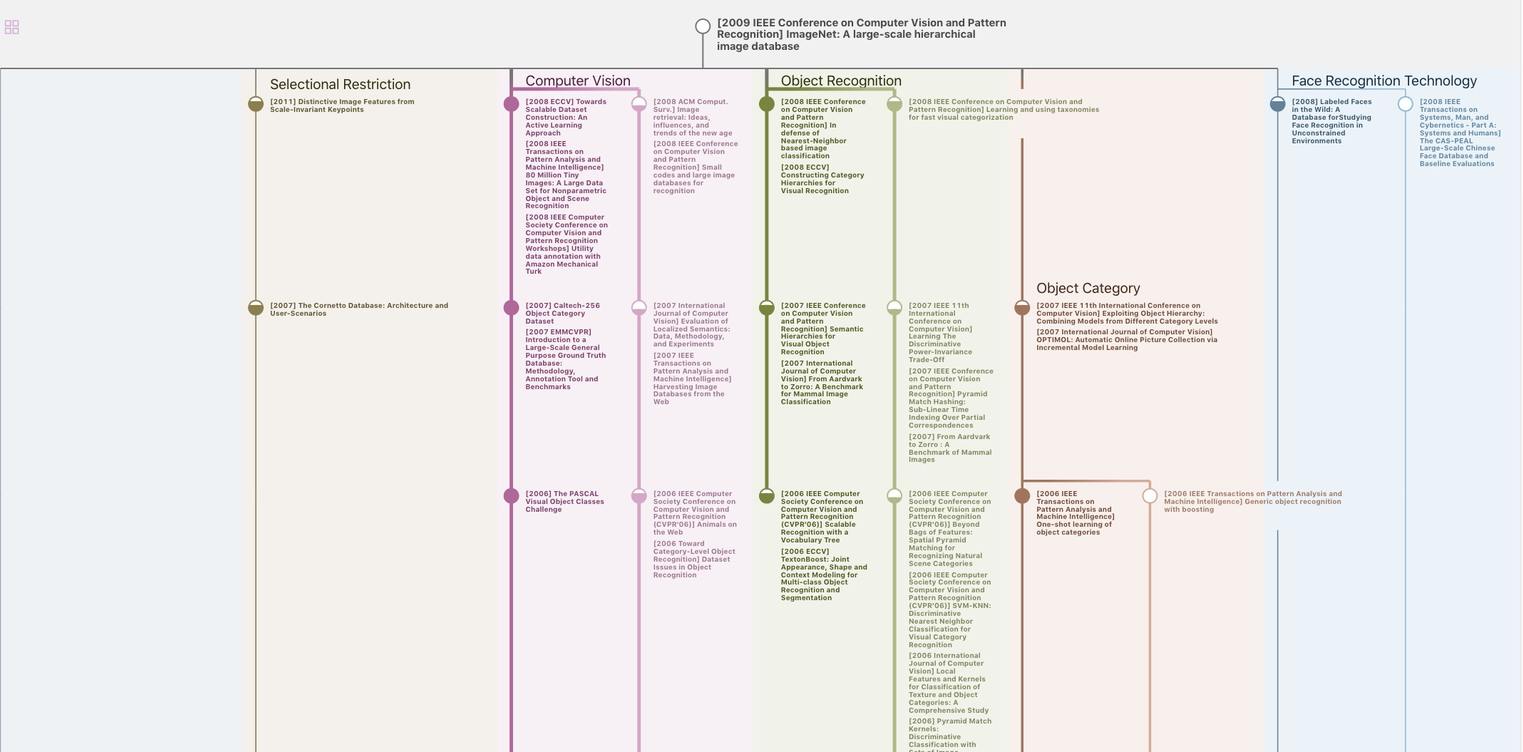
生成溯源树,研究论文发展脉络
Chat Paper
正在生成论文摘要