Improving the accuracy of AOD by using multi-sensors data over the Red Sea and the Persian Gulf
ATMOSPHERIC POLLUTION RESEARCH(2024)
Abstract
Aerosol Optical Depth (AOD) retrieved using Cloud-Aerosol LIDAR with Orthogonal Polarization (CALIOP) and Moderate Resolution Imaging Spectroradiometer (MODIS) plays a crucial role in aerosol sensing. However, the performance of these products varies across different aerosol concentrations. This research assessed the performance of CALIOP and MODIS AOD products over a three-year period (January 2017 to June 2019) under various aerosol concentrations in the Red Sea and the Persian Gulf. The products were compared with ship-based AERONET (Aerosol Robotic Network) measurements conducted in the study area. The findings reveal that MODIS AOD products exhibit greater reliability during clear days, whereas CALIOP AOD products are more accurate in regions characterized by high aerosol concentrations. However, CALIOP's limited product resolution prevents it from providing adequate spatial-temporal coverage under heavy aerosol concentrations. To enhance the accuracy of MODIS AOD, this paper proposes a methodology based on various machine learning (ML) al-gorithms, including Random Forest (RF), Multi-layer Perceptron (MLP), Extreme Gradient Boosting (XGBoost), Support Vector Machine (SVR), and Bayesian network. The study explores the performance of MODIS and CALIOP AOD products during moderate and pollution days in the Persian Gulf and Red Sea regions.The ML models are ranked based on their accuracy, with the order being XGBoost > MLP > SVRrbf > RF > Bayesian > SVRlinear for the annual and two semi-annual networks. In other words, the Bayesian method demonstrates higher efficiency (R-2 = 0.97, 0.91, and 0.90) in handling seasonal subsets. The proposed model's outcomes are validated using ship-based AERONET AOD data. By comparing the estimated AOD values with the ship-based AERONET AOD values and analyzing the overall correlation results, it is evident that machine learning techniques effectively provide accurate AOD estimates for moderate and pollution days.
MoreTranslated text
Key words
AOD,CALIOP,MODIS,Heavy aerosol concentrations,Machine learning algorithms
AI Read Science
Must-Reading Tree
Example
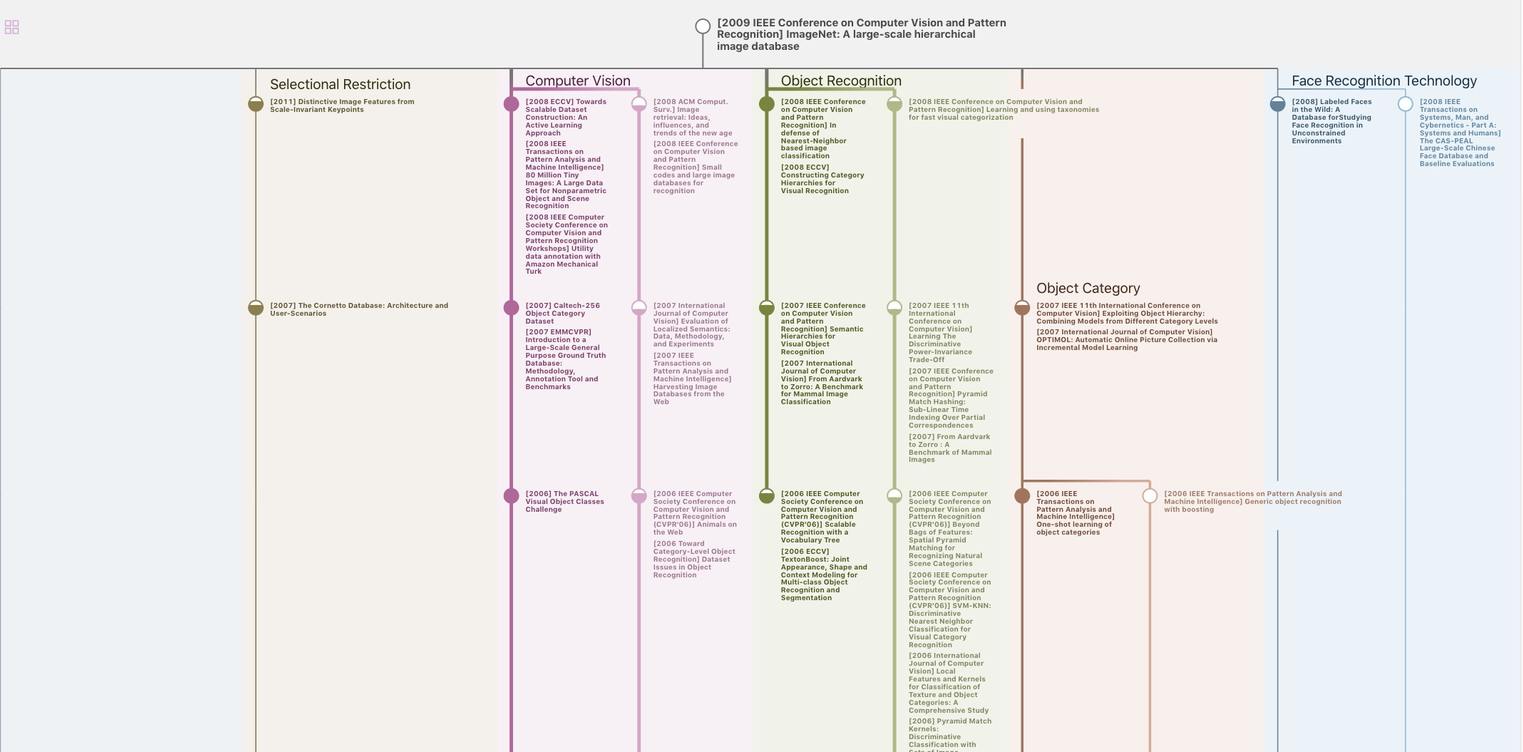
Generate MRT to find the research sequence of this paper
Chat Paper
Summary is being generated by the instructions you defined