Semi-supervised deep density clustering
Applied Soft Computing(2023)
摘要
Deep clustering generally obtains promising performance by learning deep feature representations. However, there are two limitations: (1) end-to-end deep density clustering needs to be explored; (2) prior information is ignored to guide the learning process. To overcome these limitations, we propose a novel semi-supervised deep density clustering (SDDC). Specifically, a convolutional autoencoder is applied to learn embedded features, and semi-supervised density peaks clustering is designed to identify stable cluster centers. Meanwhile, prior information is introduced to instruct the preferable clustering process. By integrating prior information, a joint clustering loss is directly built on embedded features to perform feature representation and cluster assignment simultaneously. Extensive experiments validate the power of SDDC for initializing and the effectiveness on clustering tasks.
更多查看译文
关键词
density,clustering,deep,semi-supervised
AI 理解论文
溯源树
样例
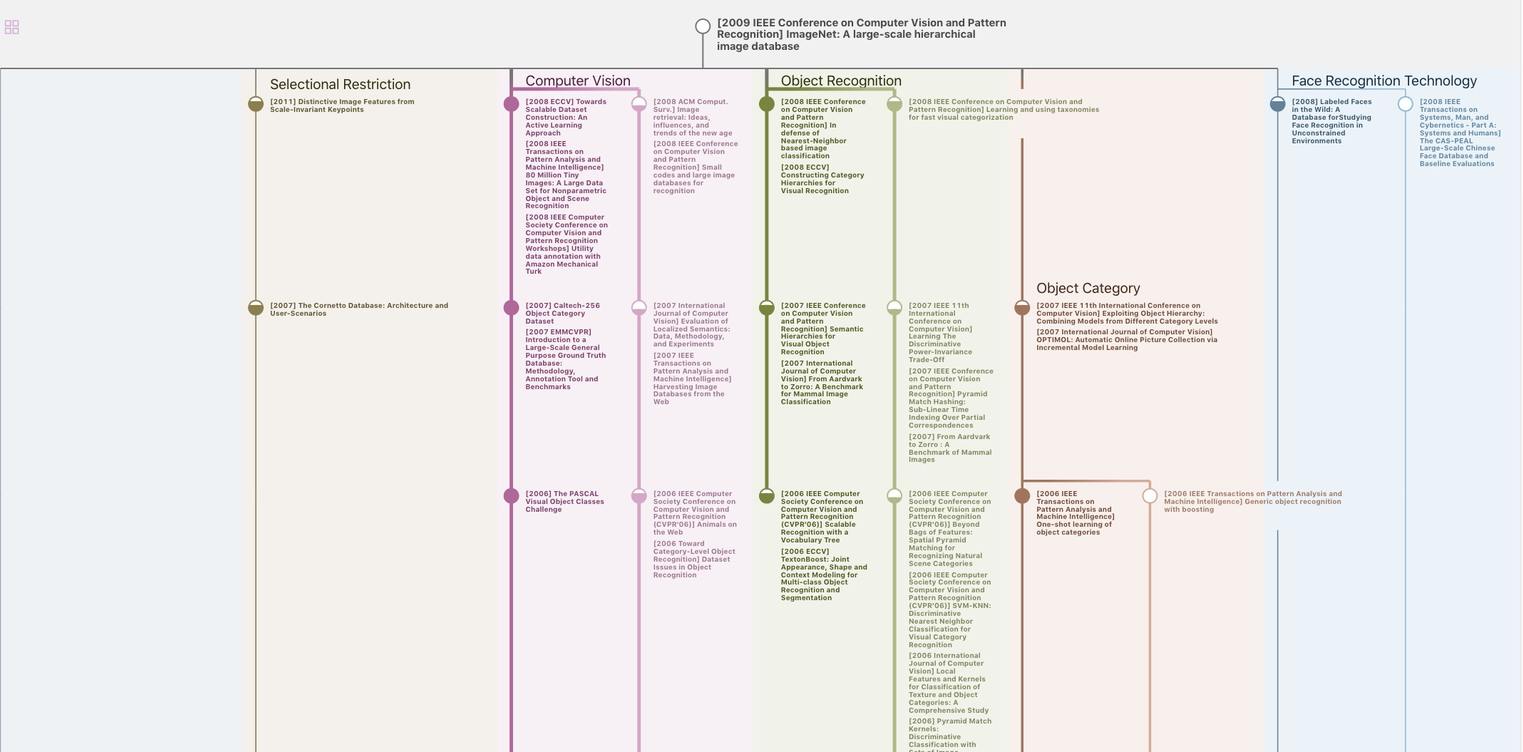
生成溯源树,研究论文发展脉络
Chat Paper
正在生成论文摘要