Semi-supervised generative adversarial networks with spatial coevolution for enhanced image generation and classification
APPLIED SOFT COMPUTING(2023)
摘要
Labeling images for classification can be expensive. Semi-Supervised Learning (SSL) Generative Adversarial Network (GAN) methods train good classifiers with a few labeled images. However, authors generally do not train SSL-GAN generators to produce new high-quality images, but as a component to train the classifier. In this article, we use a coevolutionary algorithm (CoEA) with SSL-GANs to train both the classifier and the image generative model using a few labeled images. A CoEA introduces diversity into the GAN training and mitigates training pathologies. We use a two-dimensional grid of GANs to inject diversity via distributed training that exchanges GAN components between neighboring cells based on performance and population-based hyperparameter tuning. In addition, we identify simple and efficient SSL-GAN architectures. We demonstrate the utility on three separate benchmark datasets, achieving good classification accuracy and high image quality generation while using fewer labeled data exemplars. The image quality and classification accuracy are also competitive with State-of-the-Art methods.
更多查看译文
关键词
Generative adversarial network,Semi supervised learning,Coevolution,Spatial distribution
AI 理解论文
溯源树
样例
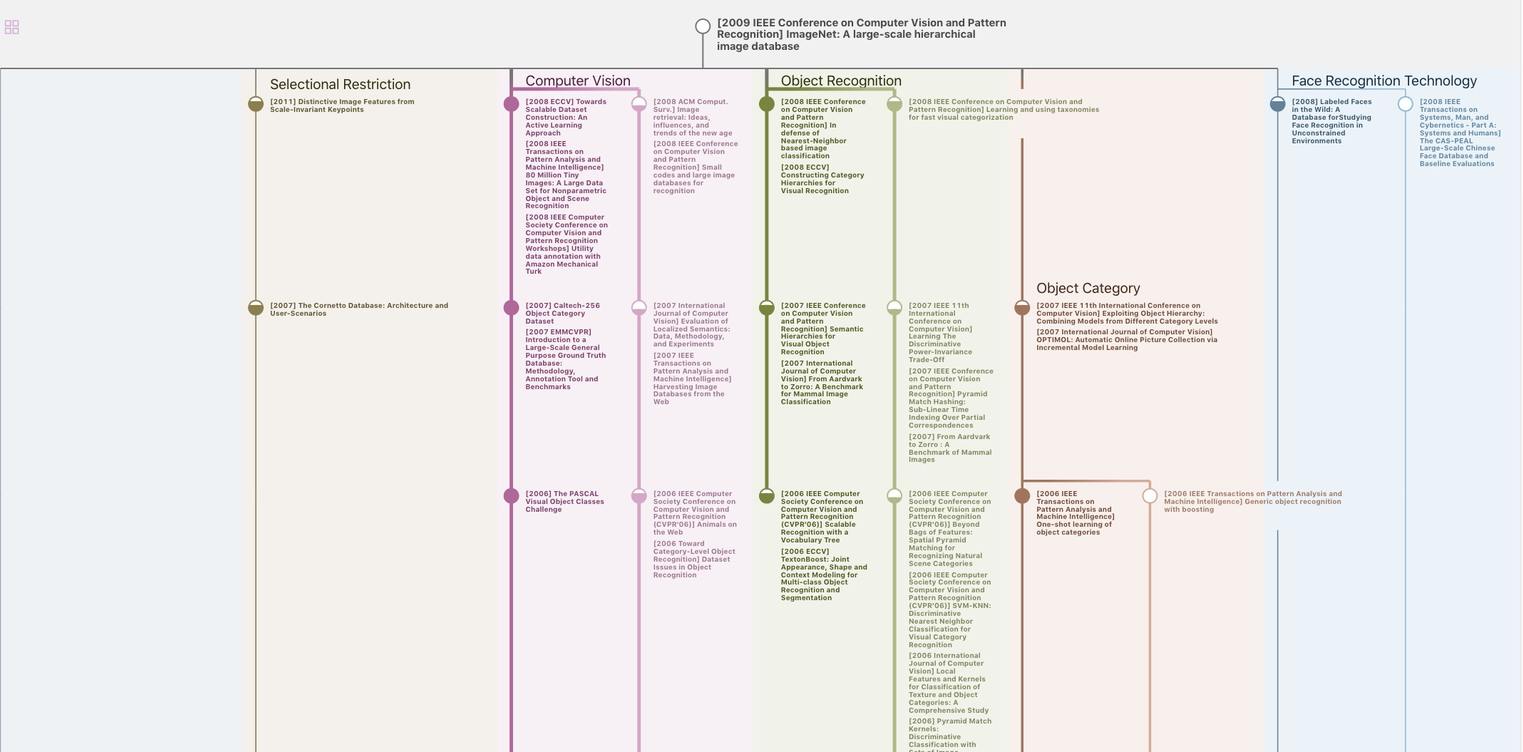
生成溯源树,研究论文发展脉络
Chat Paper
正在生成论文摘要