A heterogeneous ensemble architecture coupling model selection sorting and residual error iterative correction for crude oil price forecasting
APPLIED SOFT COMPUTING(2023)
摘要
Although crude oil price forecasts can provide policymakers, investors, consumers, and producers with scientific and reliable guidance, the nonlinearity and complexity of crude oil markets still pose serious challenges to this work. To this end, we propose a crude oil price ensemble prediction model that combines model selection sorting, multi-objective heterogeneous ensemble learning, and residual error iterative correction. First, a selection strategy based on the weighted information criterion is used to screen benchmark models in the model pool. Subsequently, candidate models are heterogeneously integrated using the multi-objective multi-verse optimization algorithm. VIseKriterijumska Optimizacija I Kompromisno Resenje, Technique for Order Preference by Similarity to Ideal Solution, and Grey Relational Analysis are utilized to select the optimal non-dominated solution of the Pareto surface. Finally, correction technology is designed to adaptively correct the residual error. Optimum error shrinkage and the number of corrections ensure maximum predictable component development. West Texas Intermediate and Europe Brent spot price data are utilized for experimental verification. Results show that the model can accurately describe crude oil price fluctuations, and has excellent robustness and stability. The forecasting method can be used as a reliable and promising energy economic decision algorithm.
更多查看译文
关键词
Crude oil price forecasting,Model selection sorting,Heterogeneous ensemble learning,Multi -objective optimization,Residual error iterative correction
AI 理解论文
溯源树
样例
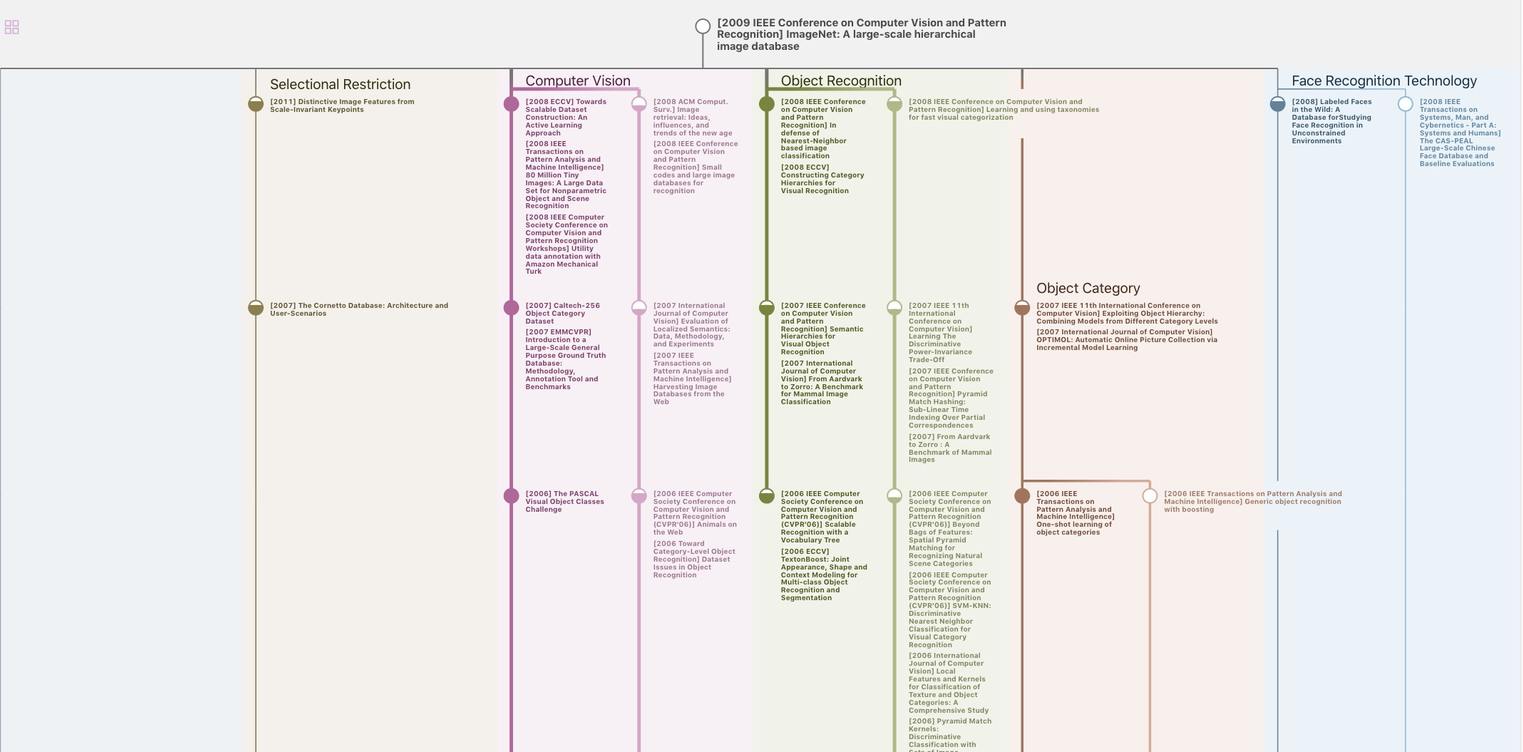
生成溯源树,研究论文发展脉络
Chat Paper
正在生成论文摘要