A fractional-order visual neural network for collision sensing in noisy and dynamic scenes
Applied Soft Computing(2023)
摘要
Autonomously sensing collision threats in intelligent mobile machines is a significant challenge. Inspired by the perfect collision sensing system of locusts, various neural networks based on lobular giant motion detectors (LGMD) have been proposed. These networks use integer-order differential operators to simulate the simple and efficient visual neural system of locusts. However, some biological studies have shown that the neurodynamics of neuronal response should be fractional-order, and fractional-order differential operators can accurately describe the biological logic brain neural response process. In view of this, firstly, this paper uses a fractional-order differential operator to simulate the membrane potential response of neurons, and a new fractional-order LGMD collision sensing visual neural network is proposed. This network is designed to accurately detect looming objects and generate reliable response spikes. Then, through experimental analysis, a range [0.36, 0.4] of order in the fractional-order system is given, which is considered to be a reasonable interval for modeling the behavior of biological nervous systems. This maybe contribute to the study of the locust's fractional-order biological nervous system. Finally, the network was systematically tested in noisy and complex dynamic environments. The experimental results demonstrated that the fractional-order LGMD visual neural network generated responses with high adaptability and reliability, showcasing significantly improved collision detection performance.
更多查看译文
关键词
Visual neural network,LGMD neuron structure,Fractional-order differential operator,Collision sensing,Complex dynamic scenes
AI 理解论文
溯源树
样例
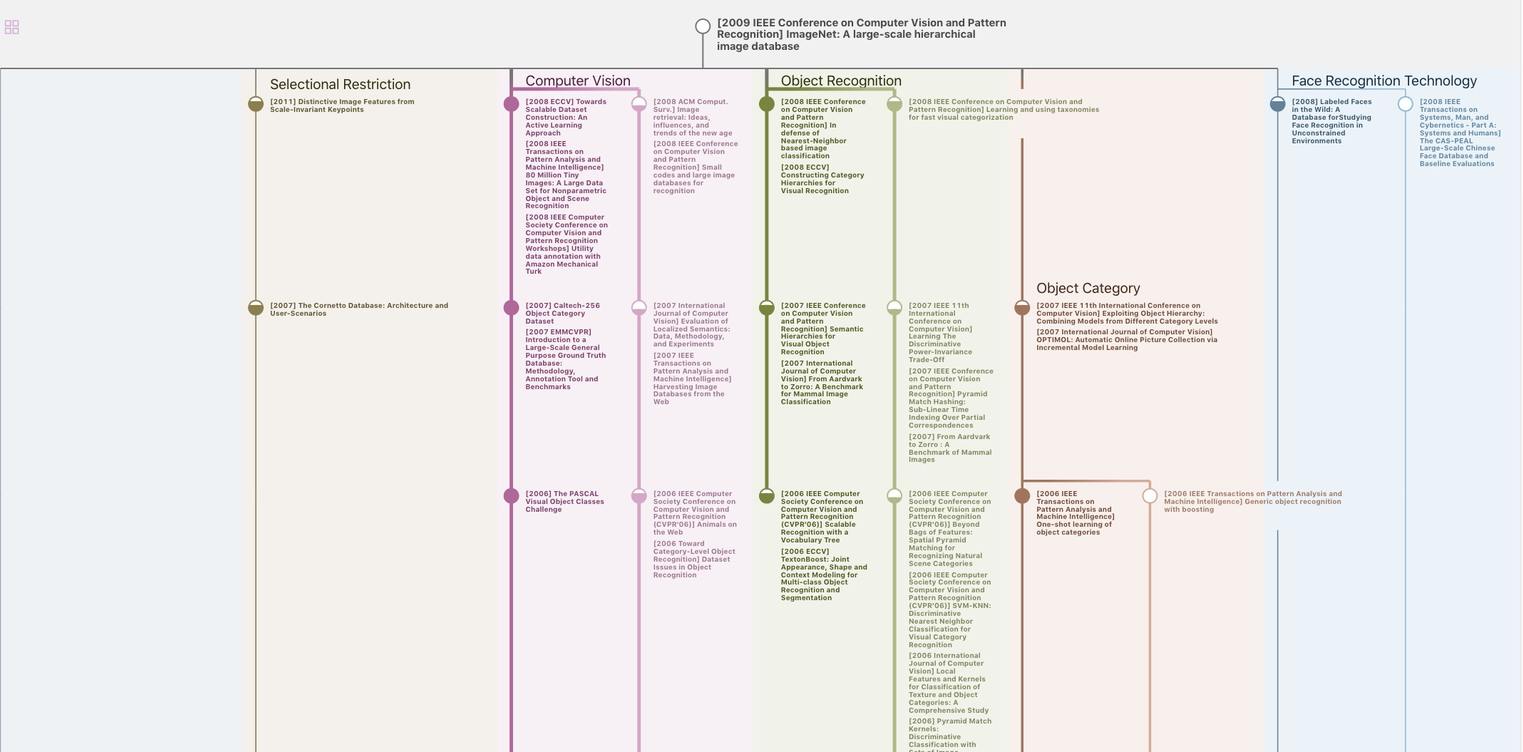
生成溯源树,研究论文发展脉络
Chat Paper
正在生成论文摘要