Data-driven distributionally robust support vector machine method for multiple criteria sorting problem with uncertainty
APPLIED SOFT COMPUTING(2023)
摘要
The multiple criteria sorting decision is a critical area of research in decision science. However, accurately capturing the decision maker's cognition for each criterion using precise values poses significant challenges. To address this issue, our study investigates an uncertain multiple criteria sorting problem with indeterminate values for each criterion, which closely aligns with real-life decision-making processes. We adopt stochastic programming (SP) and distributionally robust optimization (DRO) to deal with the associated uncertainties. We formulate the preference learning process as a binary classification problem, where a piecewise-linear function is employed to measure the preference. Specifically, we develop a distributionally robust support vector machine model that incorporates the Wasserstein metric. By leveraging duality theory, the resulting DRO-based model can be transformed into a tractable convex problem. Then, we introduce an incremental projected subgradient algorithm to solve the tractable model, which can effectively utilize the separable structure. Finally, the efficiency of the proposed method is demonstrated through numerical experiments. The comparative evolution of the marginal value functions exhibits a similar tendency, highlighting the advantage and robustness of our approach.
更多查看译文
关键词
Multiple criteria sorting,Distributionally robust optimization,Wasserstein metric,Support vector machine,Preference disaggregation approach
AI 理解论文
溯源树
样例
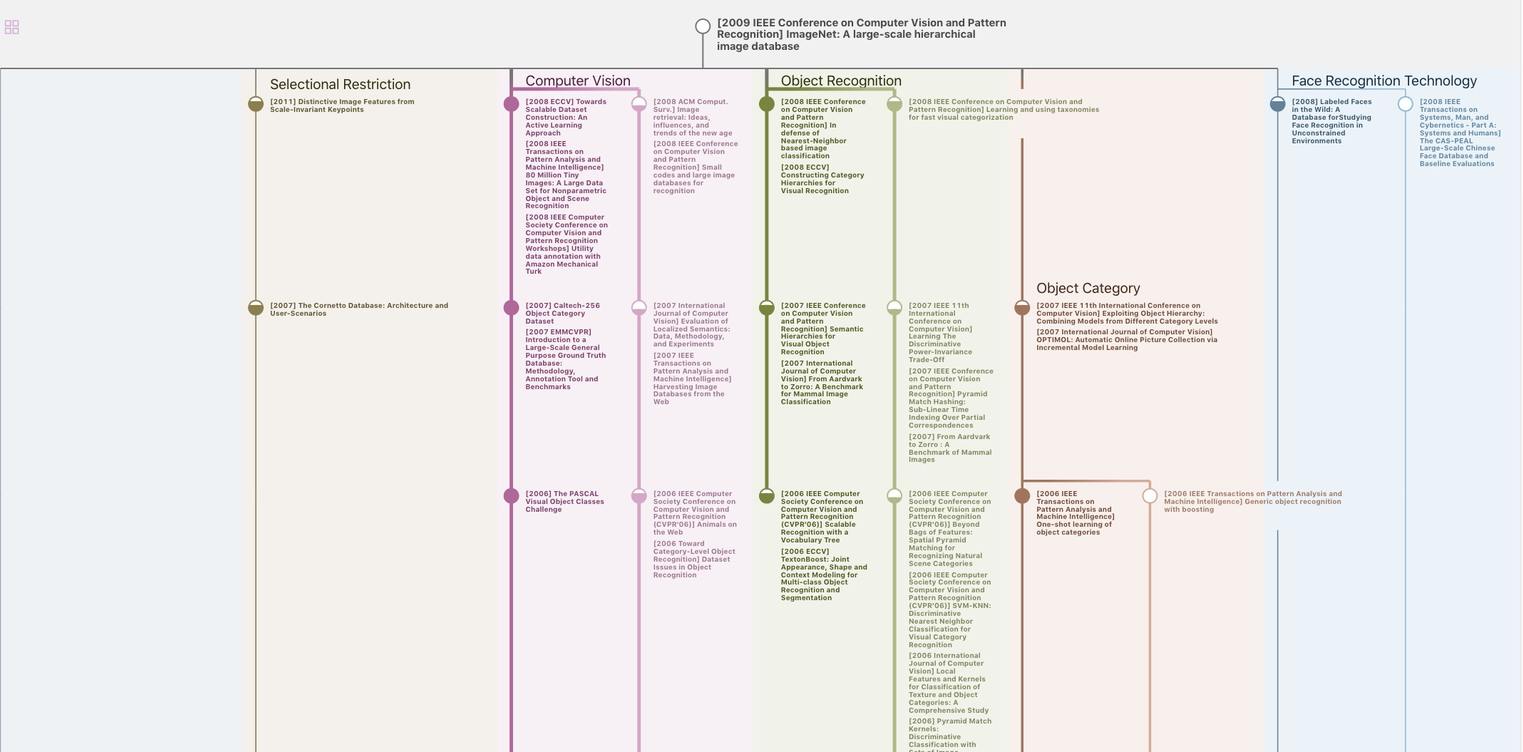
生成溯源树,研究论文发展脉络
Chat Paper
正在生成论文摘要