An adaptive multi-fidelity optimization framework based on co-Kriging surrogate models and stochastic sampling with application to coastal aquifer management
ADVANCES IN WATER RESOURCES(2023)
摘要
Surrogate modelling has been used successfully to alleviate the computational burden that results from highfidelity numerical models of seawater intrusion in simulation-optimization routines. Nevertheless, little attention has been given to multi-fidelity modelling methods to address cases where only limited runs with computationally expensive seawater intrusion models are considered affordable imposing a limiting factor for singlefidelity surrogate-based optimization as well. In this work, a new adaptive multi-fidelity optimization framework is proposed based on co-Kriging surrogate models considering two model fidelities of seawater intrusion. The methodology is tailored to the needs of solving pumping optimization problems with computationally expensive constraint functions and utilizes only small high-fidelity training datasets. Results from both hypothetical and real-world optimization problems demonstrate the efficiency and practicality of the proposed framework to provide a steep improvement of the objective function while it outperforms a comprehensive single-fidelity surrogate-based optimization method. The method can also be used to locate optimal solutions in the region of the global optimum when larger high-fidelity training datasets are available.
更多查看译文
关键词
Pumping optimization,Surrogate modelling,Multi-fidelity,Coastal aquifers,Co-Kriging
AI 理解论文
溯源树
样例
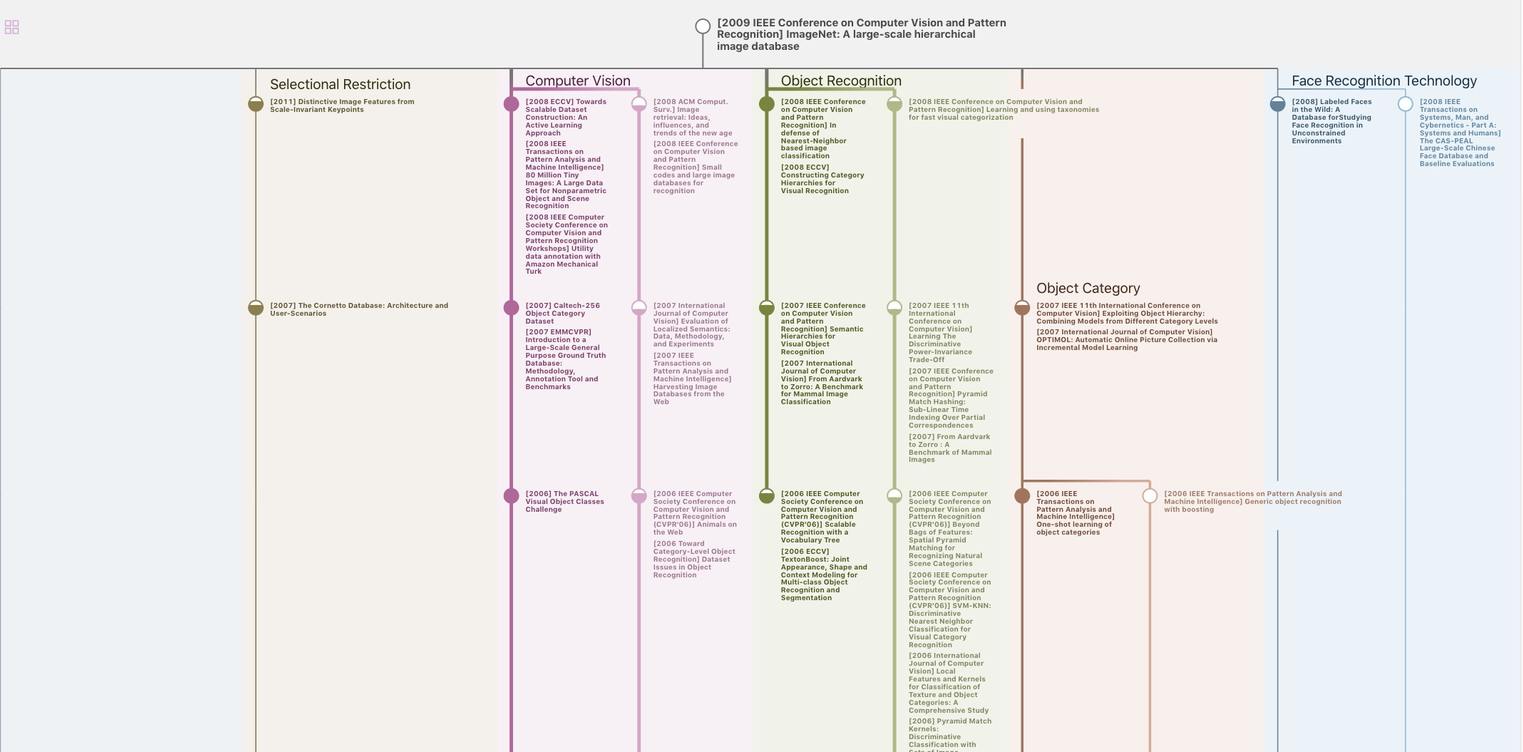
生成溯源树,研究论文发展脉络
Chat Paper
正在生成论文摘要