Quantum convolutional neural network based on variational quantum circuits
OPTICS COMMUNICATIONS(2024)
摘要
Machine learning algorithms are becoming increasingly resource-intensive. In contrast to classical computing, quantum computing holds the potential with exponential advantages and promises to overcome the bottleneck in computing performance. Taking inspiration from the convolution and pooling operations of convolutional neural networks, a quantum convolutional neural network (QCNN) is designed based on pure variational quantum circuits. By combining the advantages of angle encoding and amplitude encoding, a tree-structured hybrid amplitude encoding method has been developed. This method provides flexibility and stability in adjusting the width and the depth of the quantum circuit required for data encoding. Experiments conducted on the MNIST dataset and the Fashion-MNIST dataset demonstrate that the QCNN achieves faster convergence speed and higher image classification accuracy than the classical convolutional neural networks. Specifically, the QCNN reaches convergence after just 50 iterations, whereas the classical counterpart requires more than 200 iterations to converge. Furthermore, when performing the PCA dimensionality reduction to 8, the QCNN with Circuit 6 achieves an accuracy of 98.54% on the MNIST dataset, while the classical counterpart achieves only 86.32%.
更多查看译文
关键词
Quantum convolutional neural network,Pure variational quantum circuits,Tree-structured hybrid amplitude encoding,Image classification,Quantum machine learning
AI 理解论文
溯源树
样例
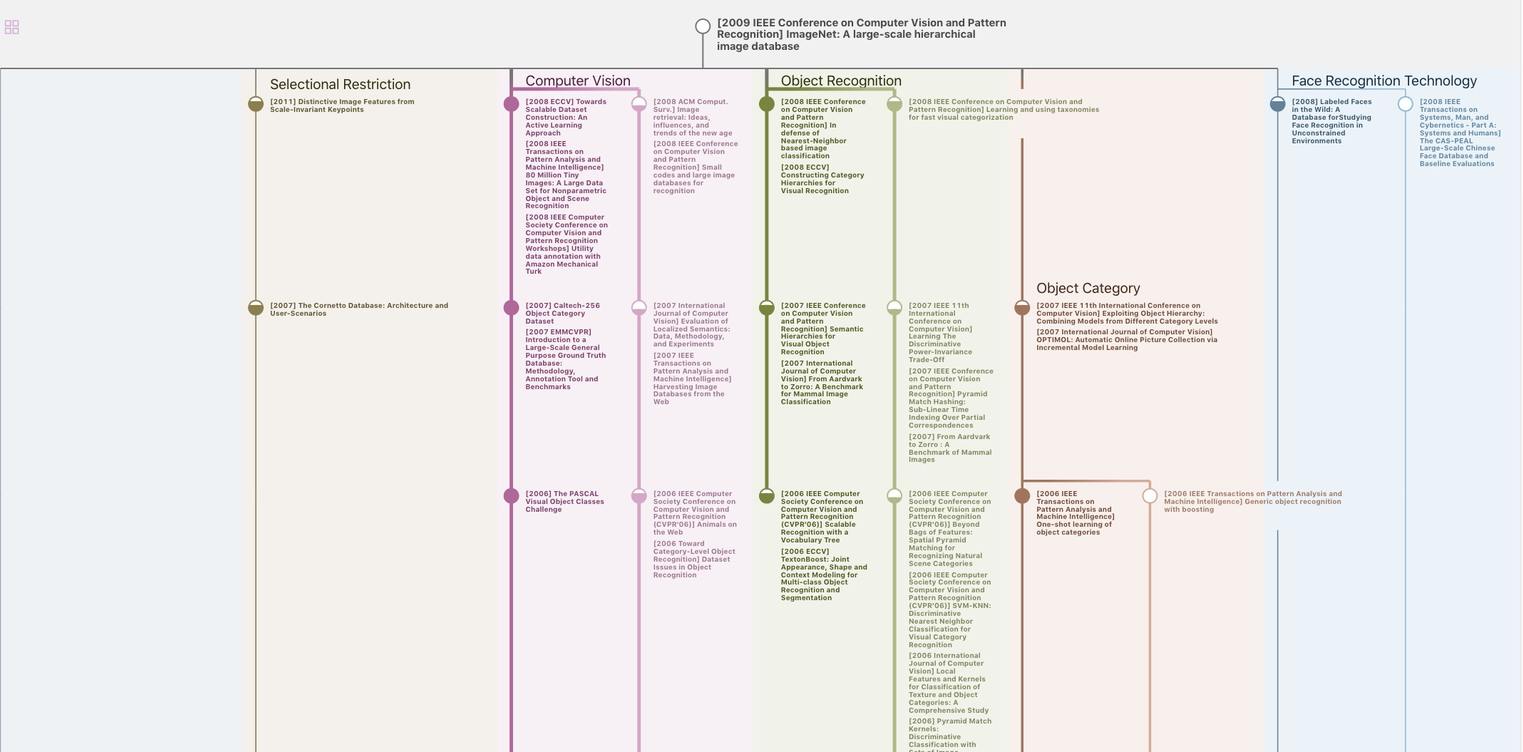
生成溯源树,研究论文发展脉络
Chat Paper
正在生成论文摘要