Visual interpretation of deep deterministic policy gradient models for energy consumption prediction
JOURNAL OF BUILDING ENGINEERING(2023)
摘要
Accurate prediction of energy consumption is pivotal to achieving sustainable building energy objectives, and Deep Reinforcement Learning (DRL) has demonstrated efficacy in this regard. Nevertheless, efficient model training within DRL remains challenging for practitioners due to the need for expertise in Reinforcement Learning (RL) and parameter tuning. Moreover, the invisible mechanism of DRL models raises doubts among users, impeding subsequent tasks. To address these challenges, a visual analytics system named DDPGVis is proposed in this work, which focuses on exploring the experience data generated by Deep Deterministic Policy Gradient (DDPG) models used for energy consumption prediction. Specifically, temporal aggregation of steps is employed to heighten the efficiency of subsequent analysis. Feature importance analysis and dimensionality reduction of state data are utilized to help users understand the high-dimensional environment space. Simultaneously, experience data is subjected to spatio-temporal modeling, yielding dynamic network diagrams, which are utilized to analyze the experience correlations. Except for showcasing the statistics and results from the analysis of state and experience data, DDPGVis also provides a recommendation view for assisting users in parameter tuning. In corporation with three non-reinforcement learning experts, case studies demonstrate that DDPGVis can help users understand the model training process, diagnose model anomalies, and optimize the model efficiency. Compared to the parameters initially set by the same expert for energy consumption prediction, DDPGVis can recommend a better configuration that contributes to a reduction of MAE, MAPE, and RMSE by 41%, 55.62%, and 28.03%, respectively, and an increase of R2 by 7.42%.
更多查看译文
关键词
Deep reinforcement learning,Deep deterministic policy gradient,Spatio-temporal modelling,Energy consumption prediction,Visual analysis
AI 理解论文
溯源树
样例
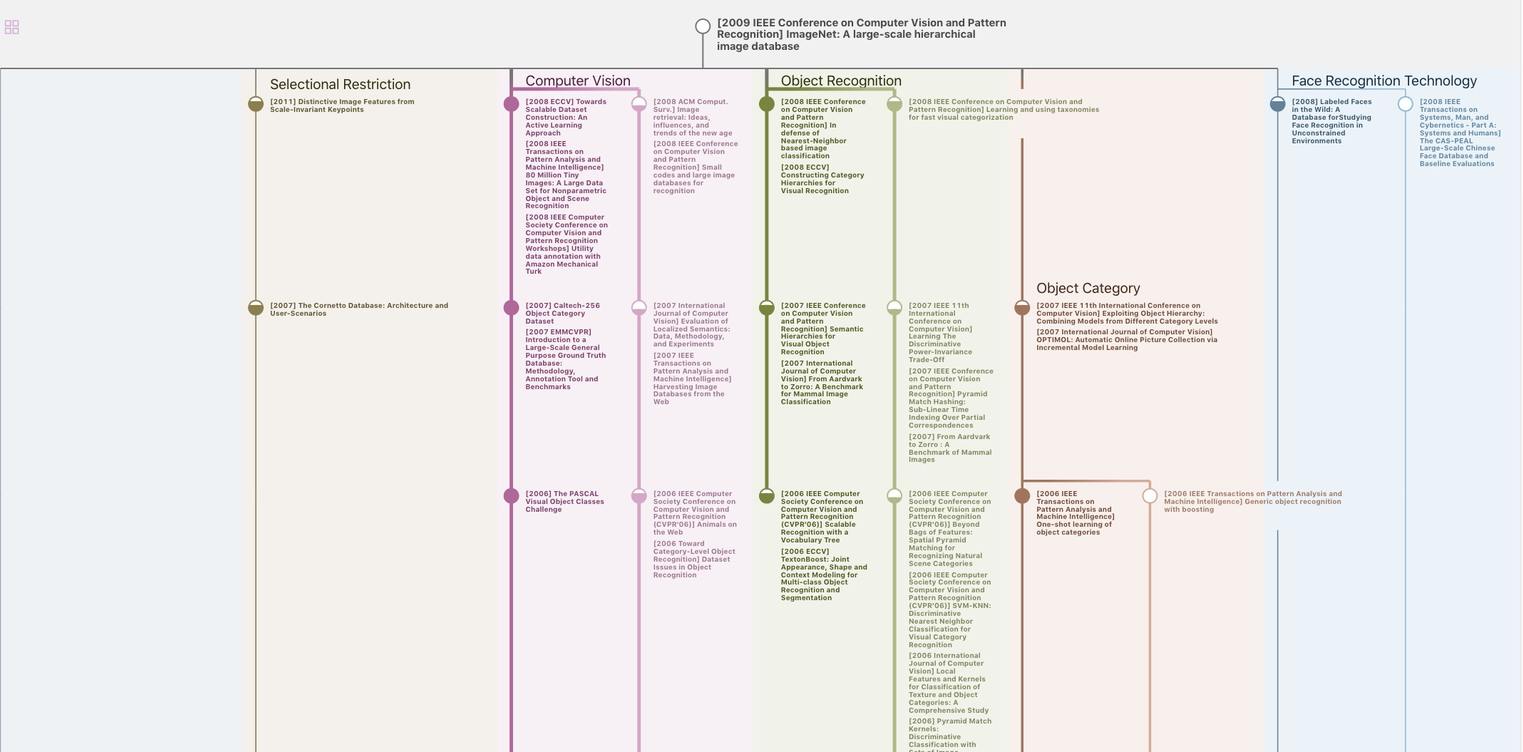
生成溯源树,研究论文发展脉络
Chat Paper
正在生成论文摘要