Dynamic Relational Graph Convolutional Network for Metro Passenger Flow Forecasting
Oper. Res. Forum(2023)
摘要
Due to the complex network structure and time-varying spatial relationships inherent in metro station systems, coupled with the influence of historical time series, station-level passenger flow forecasting tasks are challenging to solve by traditional prediction models. Therefore, we propose a dynamic relational graph convolutional network that includes a spatial-temporal framework to forecast passenger flow. Regarding spatial convolution, apart from physical and similarity graphs, we introduce accessibility graphs, which utilize the origin–destination (OD) matrix and the OD path consumption time, into graph convolutional networks. Then, dynamic graph convolution by the long short-term memory (LSTM) model is implemented with similarity graphs and accessibility graphs. Within the temporal convolution, the temporal convolutional network (TCN) module is implemented to convolve historical passenger flow to acquire passenger flow in the near future. Moreover, an automatic fare collection (AFC) dataset of the Chongqing metro system is adopted to validate the effectiveness of our proposed model with the baselines. The experimental results confirm that our model outperforms the baselines and show the effectiveness of the dynamic multirelationship and the TCN module.
更多查看译文
关键词
Metro system,Passenger flow forecasting,Dynamic graph convolution,Accessibility graphs,Temporal convolutional networks
AI 理解论文
溯源树
样例
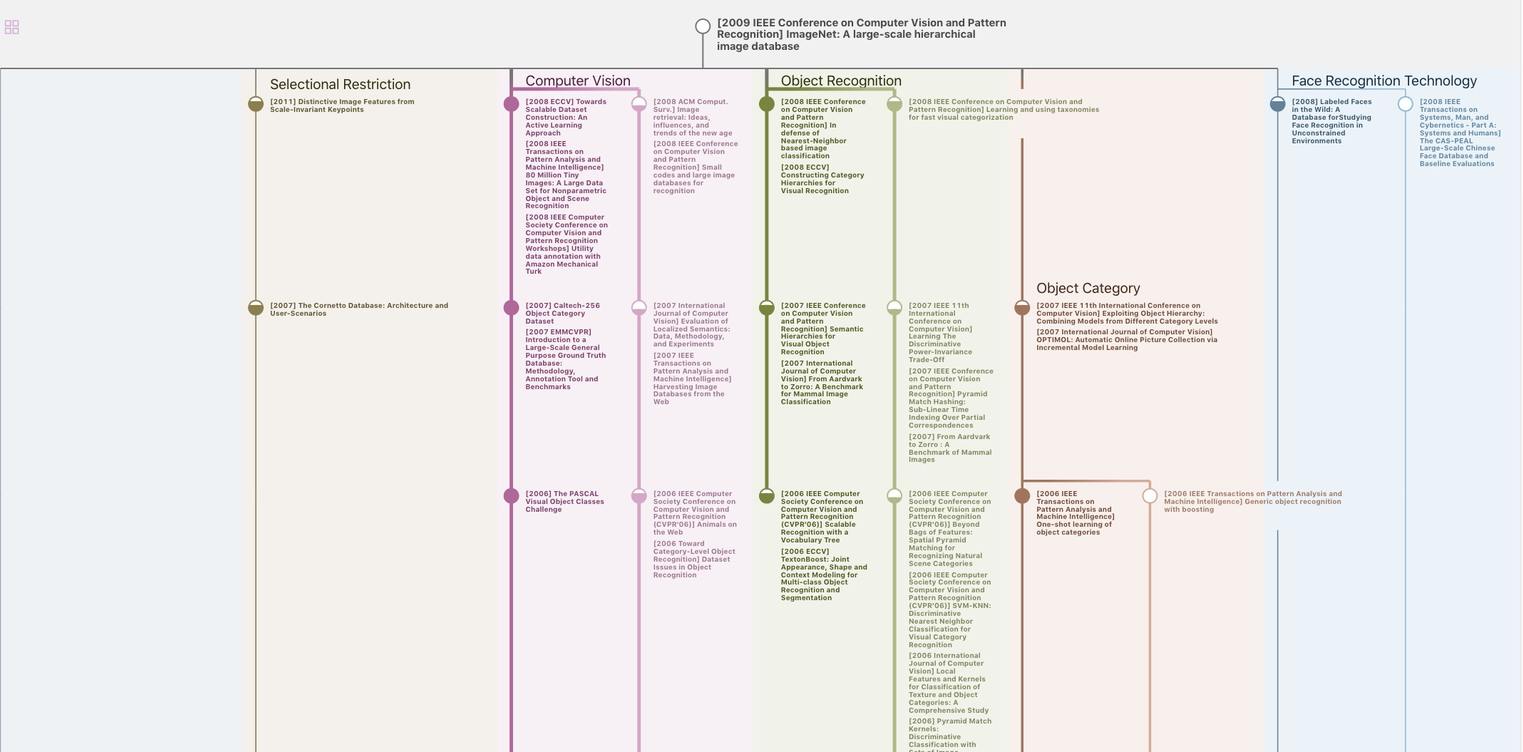
生成溯源树,研究论文发展脉络
Chat Paper
正在生成论文摘要