Person re-identification method with Mahalanobis TRM triplet on multi-branch network
APPLIED INTELLIGENCE(2023)
摘要
There are certain challenges in person re-identification tasks owing to changes in illumination, color, scale, viewpoint, and occlusion. Data augmentation and multi-branch network have been proven to be effective methods for improving the performance of person re-identification. However, current methods focus on general data augmentation and feature extraction and neglect valuable feature representation and scene characteristics. In addition, the measurements do not pertain to scenes with changing viewpoints that lack spatial information. Therefore, in this paper, we propose a person re-identification method with Mahalanobis TRM Triplet in multi-branch network to solve the above problems. First, by constructing a multi-branch network, local features and global features can be extracted and learned and more efficient fine-grained features can be extracted. Second, in order to improve the robustness of the model under a wide range of conditions, we also construct an embedded joint data augmentation module to refresh the input dataset that can handle diverse situations in which a novel random mosaic erasing method is proposed. Finally, a Mahalanobis-based Triplet loss with a Transformation Relation Matrix is proposed to encode the changing factors into the learning process and enhance the model robustness. We evaluate our methods with current mainstream benchmarks Market1501, DukeMTMCreID, and MSMT17 and achieve significant performance in terms of mAP and CMC.
更多查看译文
关键词
Person re-identification,Multi-branch network,Metric learning,Joint data augmentation
AI 理解论文
溯源树
样例
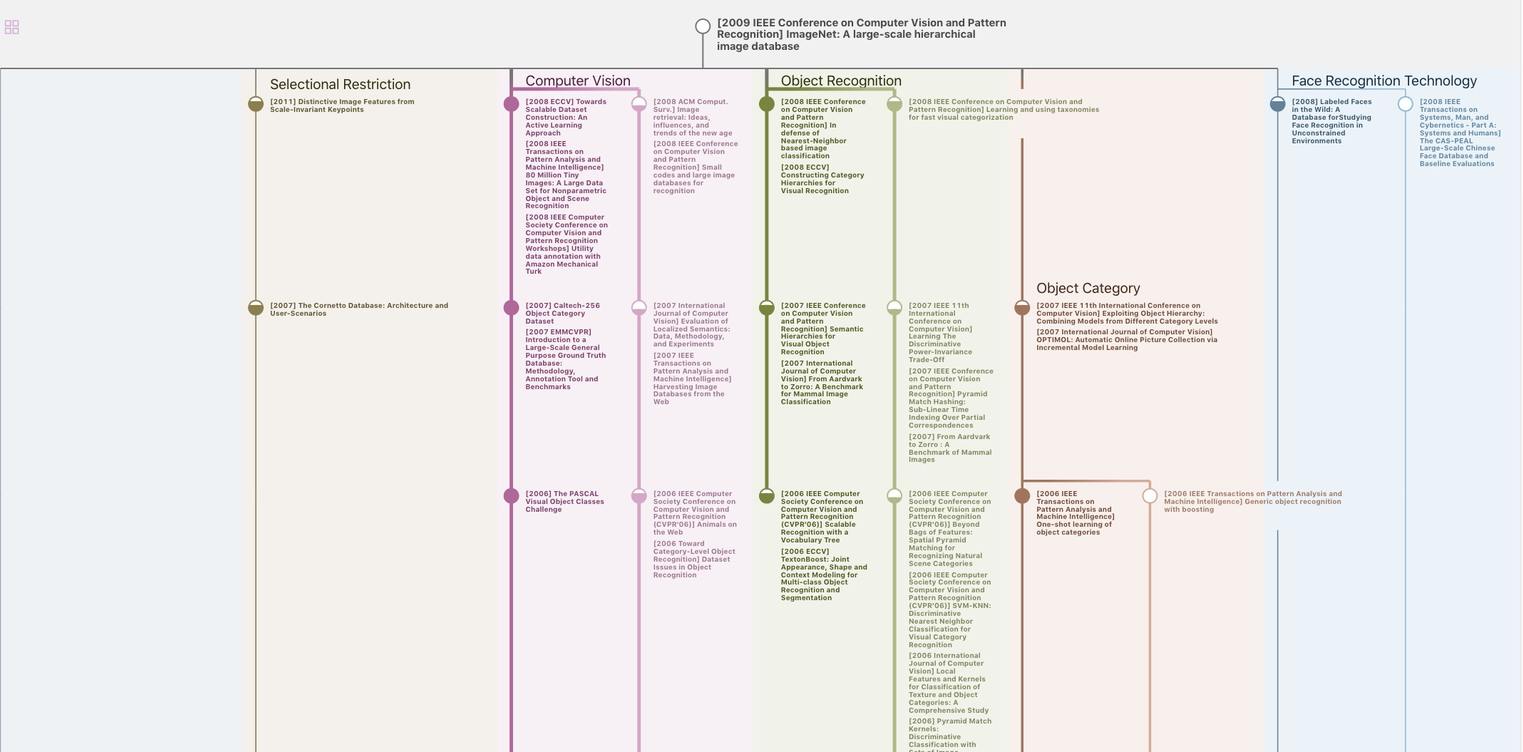
生成溯源树,研究论文发展脉络
Chat Paper
正在生成论文摘要