Contextual Bandits for Evaluating and Improving Inventory Control Policies
CoRR(2023)
摘要
Solutions to address the periodic review inventory control problem with nonstationary random demand, lost sales, and stochastic vendor lead times typically involve making strong assumptions on the dynamics for either approximation or simulation, and applying methods such as optimization, dynamic programming, or reinforcement learning. Therefore, it is important to analyze and evaluate any inventory control policy, in particular to see if there is room for improvement. We introduce the concept of an equilibrium policy, a desirable property of a policy that intuitively means that, in hindsight, changing only a small fraction of actions does not result in materially more reward. We provide a light-weight contextual bandit-based algorithm to evaluate and occasionally tweak policies, and show that this method achieves favorable guarantees, both theoretically and in empirical studies.
更多查看译文
关键词
contextual bandits,inventory control
AI 理解论文
溯源树
样例
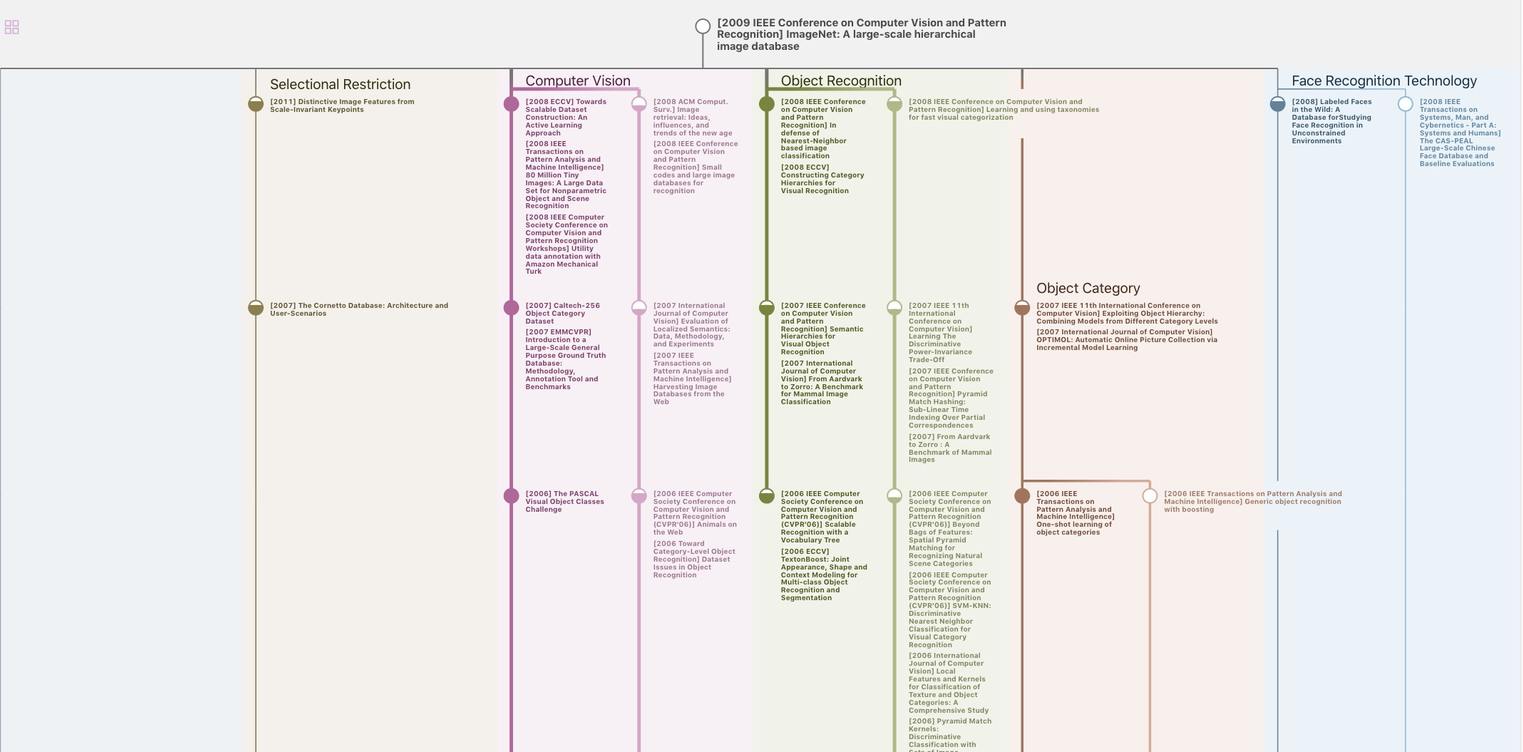
生成溯源树,研究论文发展脉络
Chat Paper
正在生成论文摘要