Metrically Scaled Monocular Depth Estimation through Sparse Priors for Underwater Robots
ICRA 2024(2024)
摘要
In this work, we address the problem of real-time dense depth estimation from monocular images for mobile underwater vehicles. We formulate a deep learning model that fuses sparse depth measurements from triangulated features to improve the depth predictions and solve the problem of scale ambiguity. To allow prior inputs of arbitrary sparsity, we apply a dense parameterization method. Our model extends recent state-of-the-art approaches to monocular image based depth estimation, using an efficient encoder-decoder backbone and modern lightweight transformer optimization stage to encode global context. The network is trained in a supervised fashion on the forward-looking underwater dataset, FLSea. Evaluation results on this dataset demonstrate significant improvement in depth prediction accuracy by the fusion of the sparse feature priors. In addition, without any retraining, our method achieves similar depth prediction accuracy on a downward looking dataset we collected with a diver operated camera rig, conducting a survey of a coral reef. The method achieves real-time performance, running at 24 FPS on a NVIDIA Jetson Xavier NX, 160 FPS on a NVIDIA RTX 2080 GPU and 7 FPS on a single Intel i9-9900K CPU core, making it suitable for direct deployment on embedded GPU systems. The implementation of this work is made publicly available at https://github.com/ebnerluca/uw_depth.
更多查看译文
关键词
Marine Robotics,Computer Vision for Automation,Deep Learning for Visual Perception
AI 理解论文
溯源树
样例
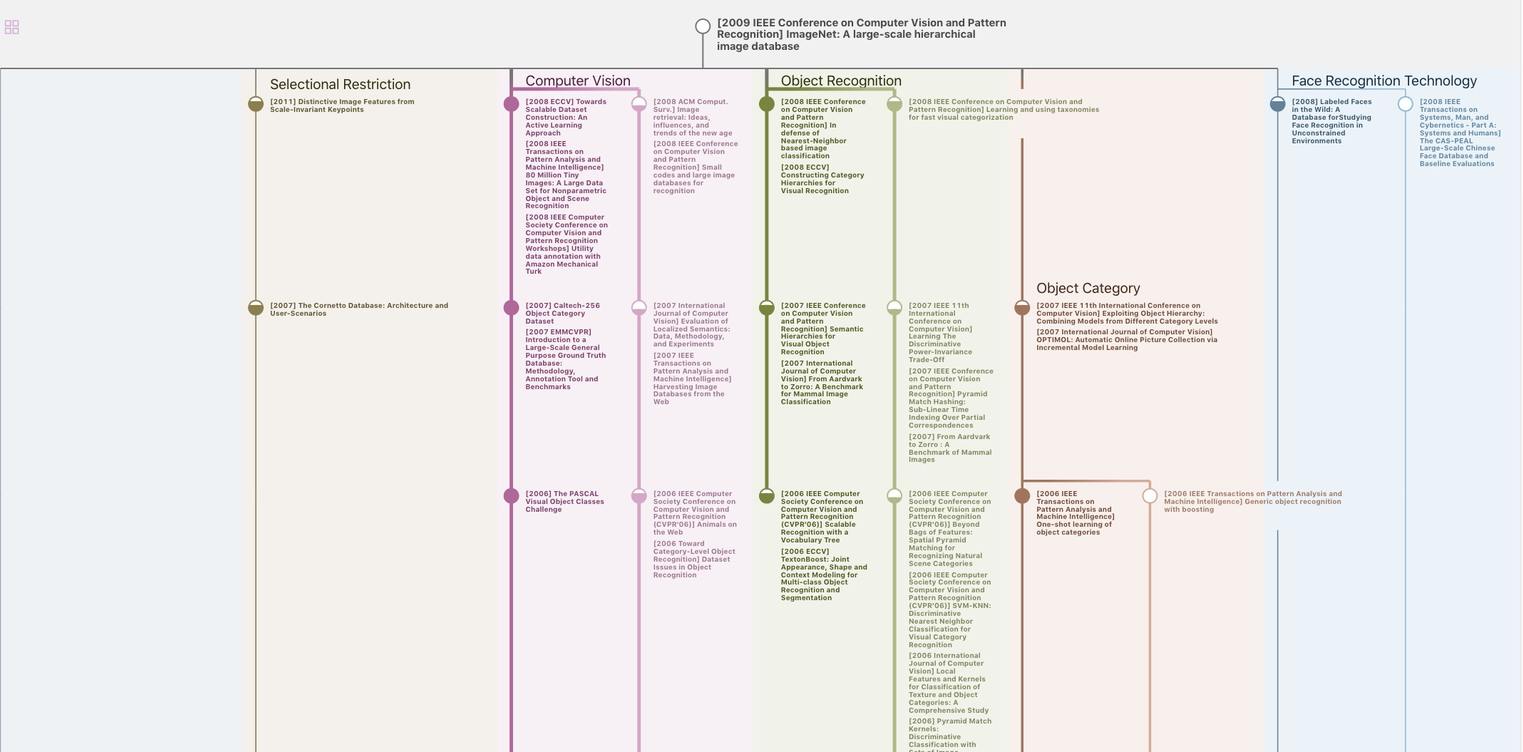
生成溯源树,研究论文发展脉络
Chat Paper
正在生成论文摘要