Score Matching-based Pseudolikelihood Estimation of Neural Marked Spatio-Temporal Point Process with Uncertainty Quantification
CoRR(2023)
摘要
Spatio-temporal point processes (STPPs) are potent mathematical tools for modeling and predicting events with both temporal and spatial features. Despite their versatility, most existing methods for learning STPPs either assume a restricted form of the spatio-temporal distribution, or suffer from inaccurate approximations of the intractable integral in the likelihood training objective. These issues typically arise from the normalization term of the probability density function. Moreover, current techniques fail to provide uncertainty quantification for model predictions, such as confidence intervals for the predicted event's arrival time and confidence regions for the event's location, which is crucial given the considerable randomness of the data. To tackle these challenges, we introduce SMASH: a Score MAtching-based pSeudolikeliHood estimator for learning marked STPPs with uncertainty quantification. Specifically, our framework adopts a normalization-free objective by estimating the pseudolikelihood of marked STPPs through score-matching and offers uncertainty quantification for the predicted event time, location and mark by computing confidence regions over the generated samples. The superior performance of our proposed framework is demonstrated through extensive experiments in both event prediction and uncertainty quantification.
更多查看译文
关键词
pseudolikelihood estimation,neural marked
AI 理解论文
溯源树
样例
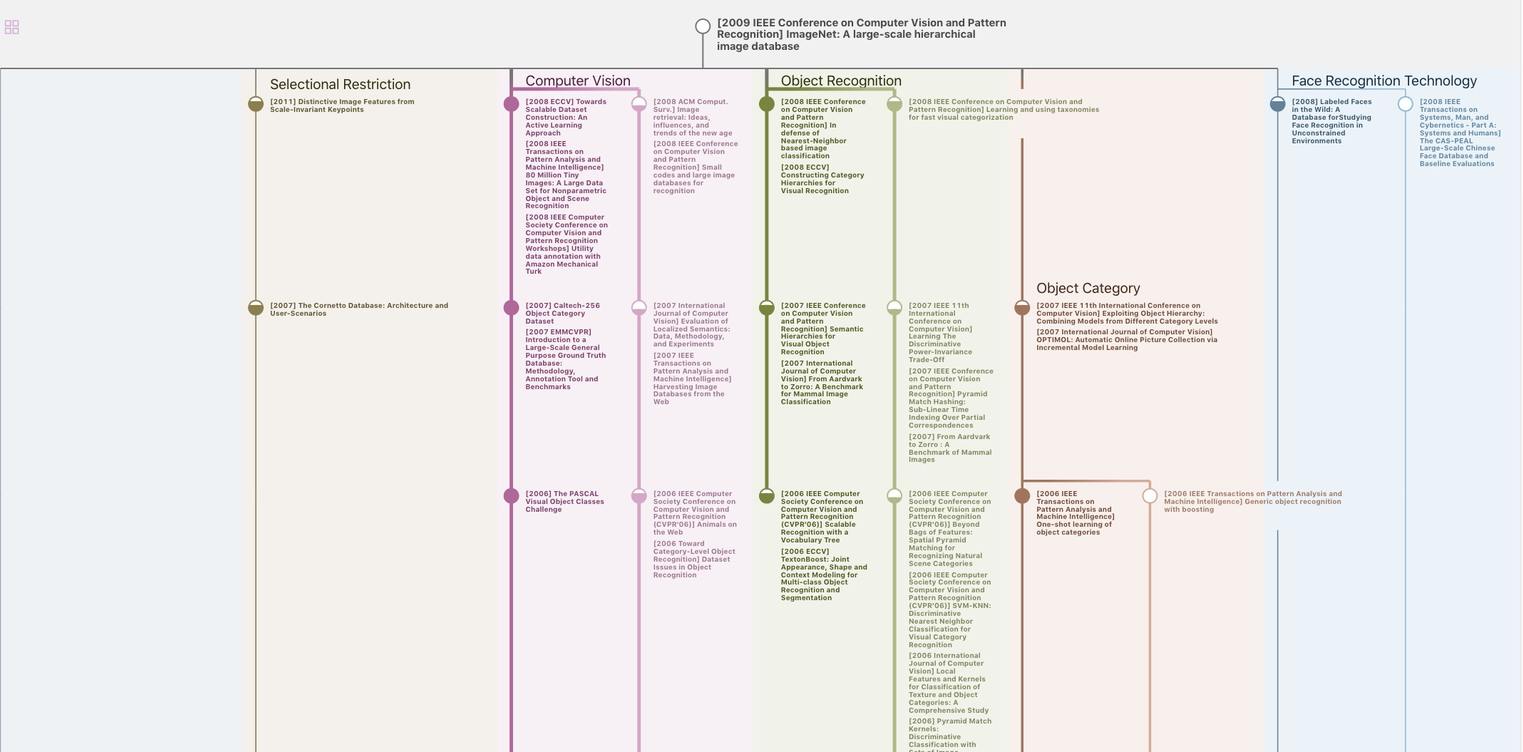
生成溯源树,研究论文发展脉络
Chat Paper
正在生成论文摘要