Attention-Based Ensemble Pooling for Time Series Forecasting
CoRR(2023)
摘要
A common technique to reduce model bias in time-series forecasting is to use an ensemble of predictive models and pool their output into an ensemble forecast. In cases where each predictive model has different biases, however, it is not always clear exactly how each model forecast should be weighed during this pooling. We propose a method for pooling that performs a weighted average over candidate model forecasts, where the weights are learned by an attention-based ensemble pooling model. We test this method on two time-series forecasting problems: multi-step forecasting of the dynamics of the non-stationary Lorenz `63 equation, and one-step forecasting of the weekly incident deaths due to COVID-19. We find that while our model achieves excellent valid times when forecasting the non-stationary Lorenz `63 equation, it does not consistently perform better than the existing ensemble pooling when forecasting COVID-19 weekly incident deaths.
更多查看译文
关键词
ensemble pooling,forecasting,time series,attention-based
AI 理解论文
溯源树
样例
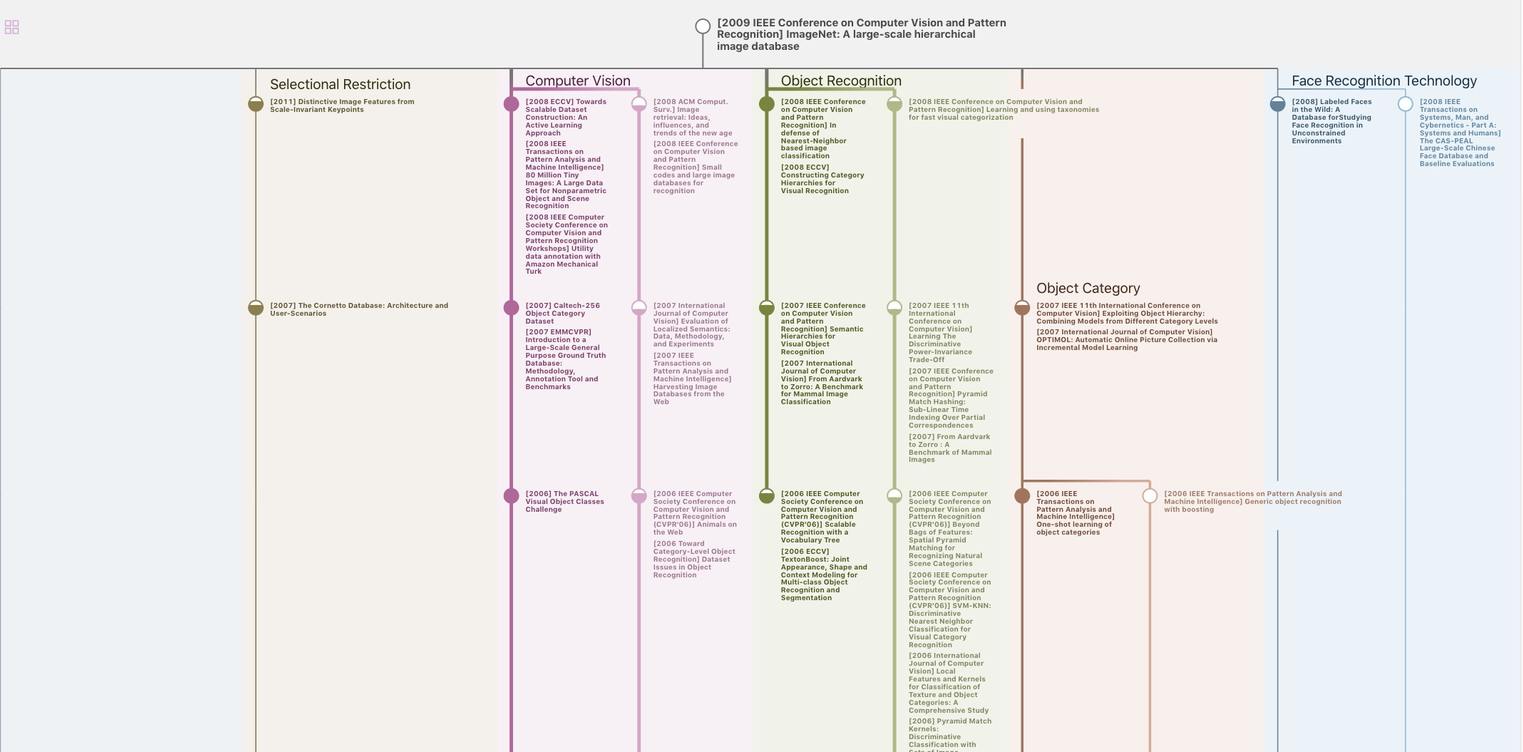
生成溯源树,研究论文发展脉络
Chat Paper
正在生成论文摘要