GraphMal: A Network Malicious Traffic Identification Method Based on Graph Neural Network
2023 International Conference on Networking and Network Applications (NaNA)(2023)
摘要
Detecting malicious attacks in normal network traffic is critical to network security. Traditional traffic identification methods often struggle to capture the complex communication patterns in network traffic, hindering their effectiveness. We introduce GraphMal, a framework utilizing Graph Neural Networks (GNNs) for the identification of malicious traffic. This approach effectively exploits network topology and traffic characteristics to improve performance. We first apply Pearson's correlation coefficient and random forest approaches to effectively reduce the dimensionality of the dataset. Then, the E-GraphSAGE algorithm is used to extract salient features from the traffic topology graph and construct a robust graph classifier. Additionally, the focal loss function is used to solve the data imbalance problem. Experiments conducted on the UNSW-NB15 dataset demonstrate GraphMal's remarkable performance in both binary and multi-class classification tasks, while improving the learning efficiency of GNNs.
更多查看译文
关键词
Network Security,Traffic Identification,Graph Neural Networks,Data Imbalance
AI 理解论文
溯源树
样例
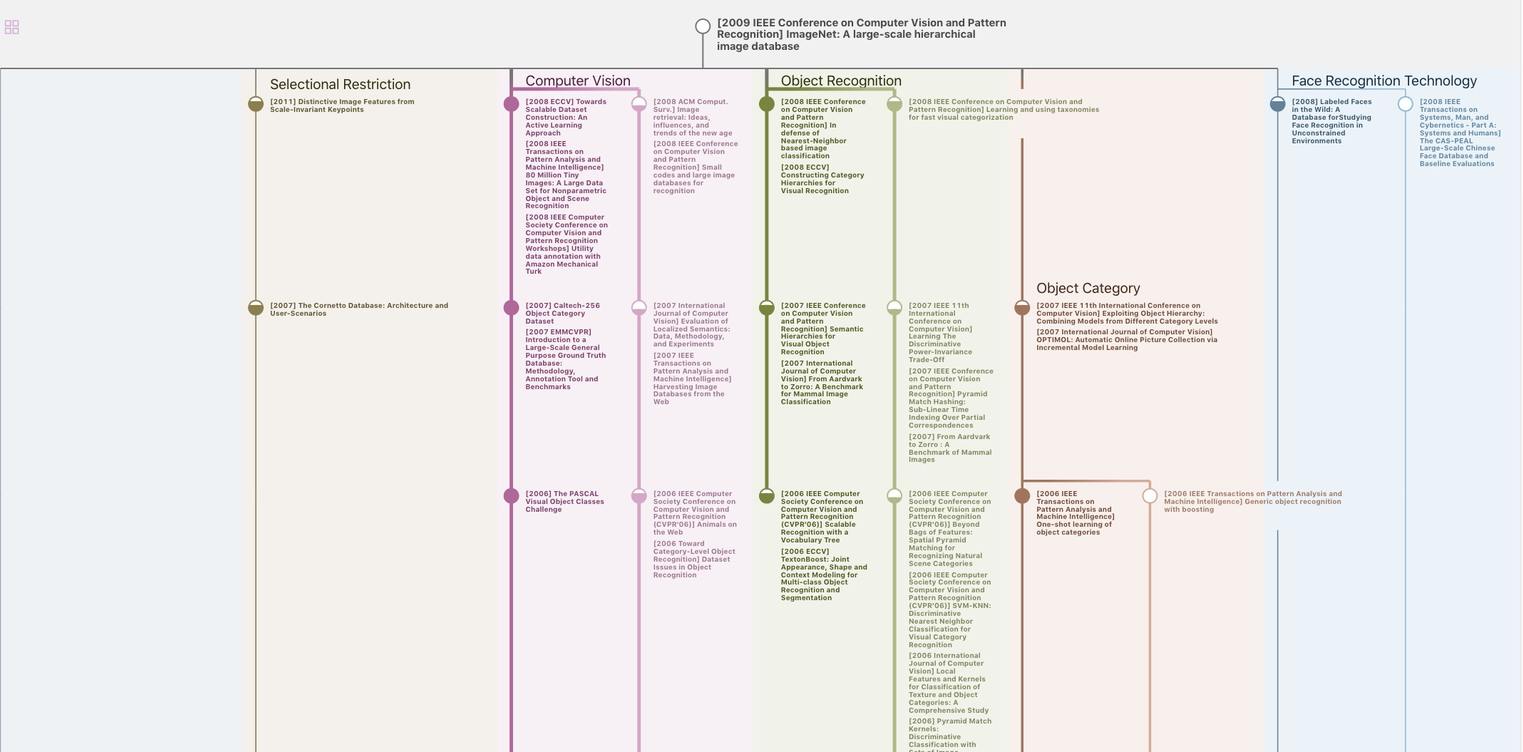
生成溯源树,研究论文发展脉络
Chat Paper
正在生成论文摘要