Distributed Clustering for Cooperative Multi-Task Learning Networks
IEEE Transactions on Network Science and Engineering(2023)
摘要
Distributed learning enables collaborative training of machine learning models across multiple agents by exchanging model parameters without sharing local data. Each agent generates data from distinct but related distributions, and multi-task learning can be effectively used to model related tasks. This article focuses on clustered multi-task learning, where agents are partitioned into clusters with distinct objectives, and agents in the same cluster share the same objective. The structure of such clusters is unknown apriori. Cooperation with the agents in the same cluster is beneficial and improves the overall learning performance. However, indiscriminate cooperation of agents with different objectives leads to undesired outcomes. Accurately capturing the clustering structure benefits the cooperation and offers many practical benefits; for instance, it helps advertising companies better target their ads. This article proposes an adaptive clustering method that allows distributed agents to learn the most appropriate neighbors to collaborate with and form clusters. We prove the convergence of every agent towards its objective and analyze the network learning performance using the proposed clustering method. Further, we present a method of computing combination weights that approximately optimizes the network's learning performance to determine how one should aggregate the neighbors' model parameters after the clustering step. The theoretical analysis is well-validated by the evaluation results using target localization and digits classification, showing that the proposed clustering method outperforms existing distributed clustering methods as well as the case where agents do not cooperate.
更多查看译文
关键词
clustering,networks,learning,multi-task
AI 理解论文
溯源树
样例
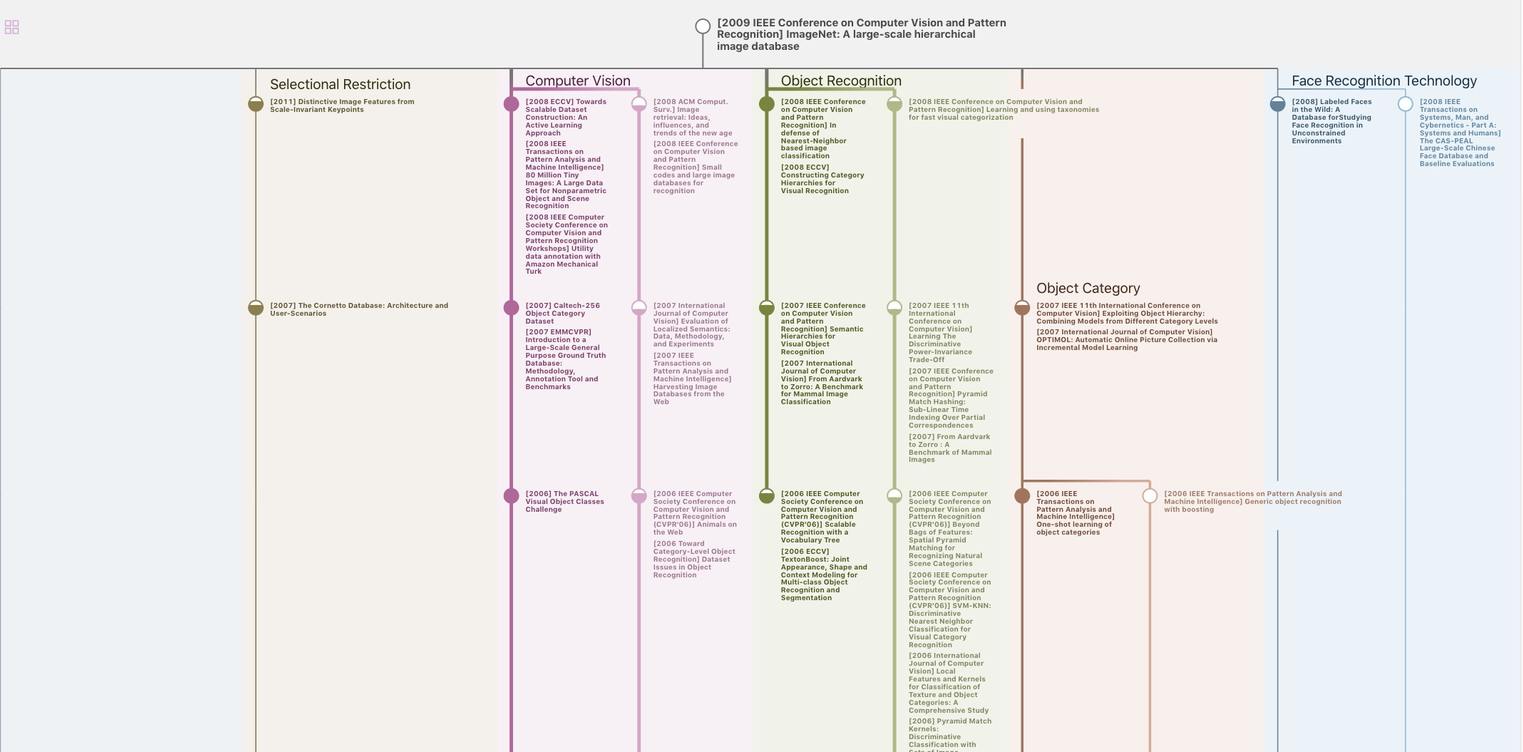
生成溯源树,研究论文发展脉络
Chat Paper
正在生成论文摘要