A comparative study of variational autoencoders, normalizing flows, and score-based diffusion models for electrical impedance tomography
JOURNAL OF INVERSE AND ILL-POSED PROBLEMS(2024)
摘要
Electrical Impedance Tomography (EIT) is a widely employed imaging technique in industrial inspection, geophysical prospecting, and medical imaging. However, the inherent nonlinearity and ill-posedness of EIT image reconstruction present challenges for classical regularization techniques, such as the critical selection of regularization terms and the lack of prior knowledge. Deep generative models (DGMs) have been shown to play a crucial role in learning implicit regularizers and prior knowledge. This study aims to investigate the potential of three DGMs - variational autoencoder networks, normalizing flow, and score-based diffusion model - to learn implicit regularizers in learning-based EIT imaging. We first introduce background information on EIT imaging and its inverse problem formulation. Next, we propose three algorithms for performing EIT inverse problems based on corresponding DGMs. Finally, we present numerical and visual experiments, which reveal that (1) no single method consistently outperforms the others across all settings, and (2) when reconstructing an object with two anomalies using a well-trained model based on a training dataset containing four anomalies, the conditional normalizing flow (CNF) model exhibits the best generalization in low-level noise, while the conditional score-based diffusion model (CSD*) demonstrates the best generalization in high-level noise settings. We hope our preliminary efforts will encourage other researchers to assess their DGMs in EIT and other nonlinear inverse problems.
更多查看译文
关键词
Electrical impedance tomography,inverse problems,deep generative model,deep learning
AI 理解论文
溯源树
样例
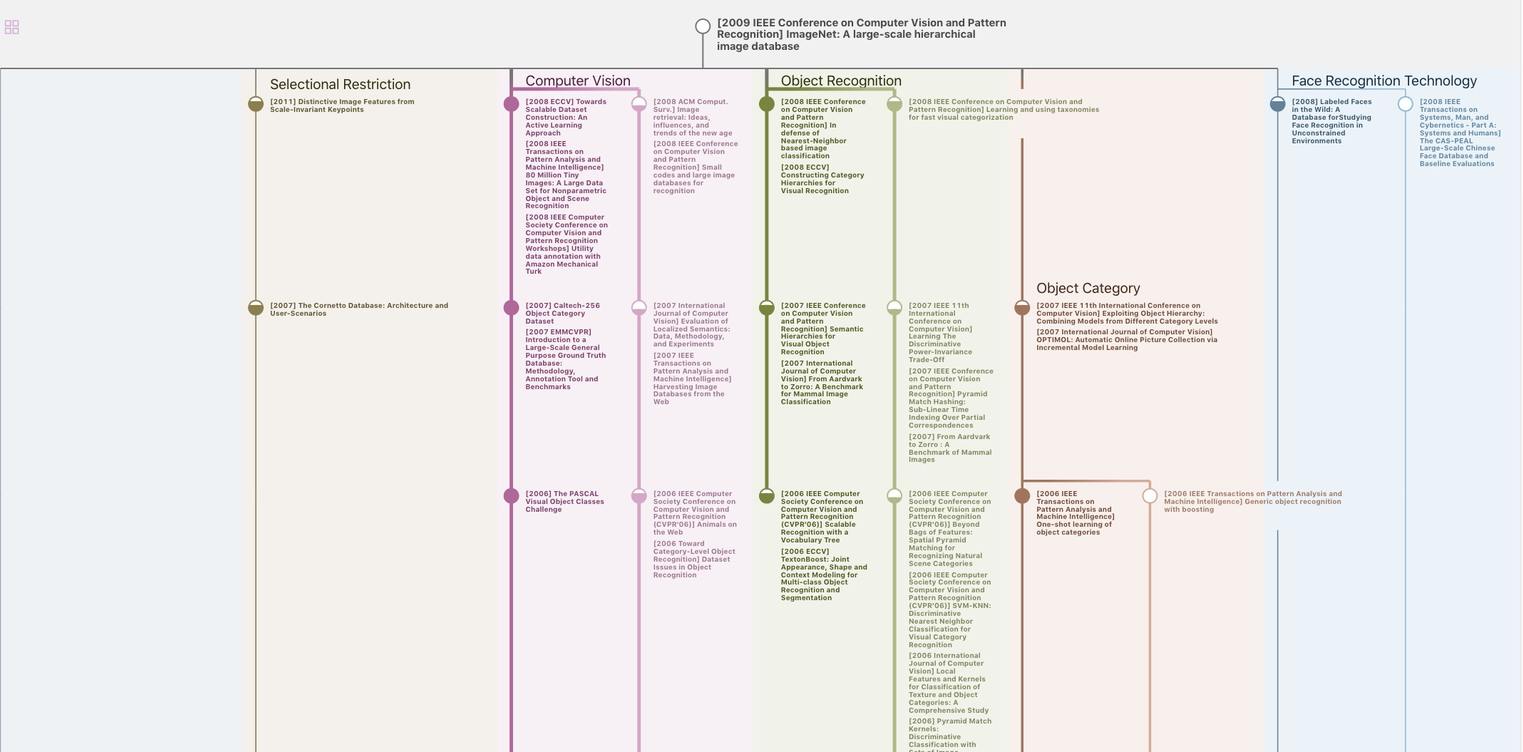
生成溯源树,研究论文发展脉络
Chat Paper
正在生成论文摘要