Let the Pretrained Language Models "Imagine" for Short Texts Topic Modeling
CoRR(2023)
摘要
Topic models are one of the compelling methods for discovering latent semantics in a document collection. However, it assumes that a document has sufficient co-occurrence information to be effective. However, in short texts, co-occurrence information is minimal, which results in feature sparsity in document representation. Therefore, existing topic models (probabilistic or neural) mostly fail to mine patterns from them to generate coherent topics. In this paper, we take a new approach to short-text topic modeling to address the data-sparsity issue by extending short text into longer sequences using existing pre-trained language models (PLMs). Besides, we provide a simple solution extending a neural topic model to reduce the effect of noisy out-of-topics text generation from PLMs. We observe that our model can substantially improve the performance of short-text topic modeling. Extensive experiments on multiple real-world datasets under extreme data sparsity scenarios show that our models can generate high-quality topics outperforming state-of-the-art models.
更多查看译文
关键词
pretrained language models,language models,short texts topic,imagine
AI 理解论文
溯源树
样例
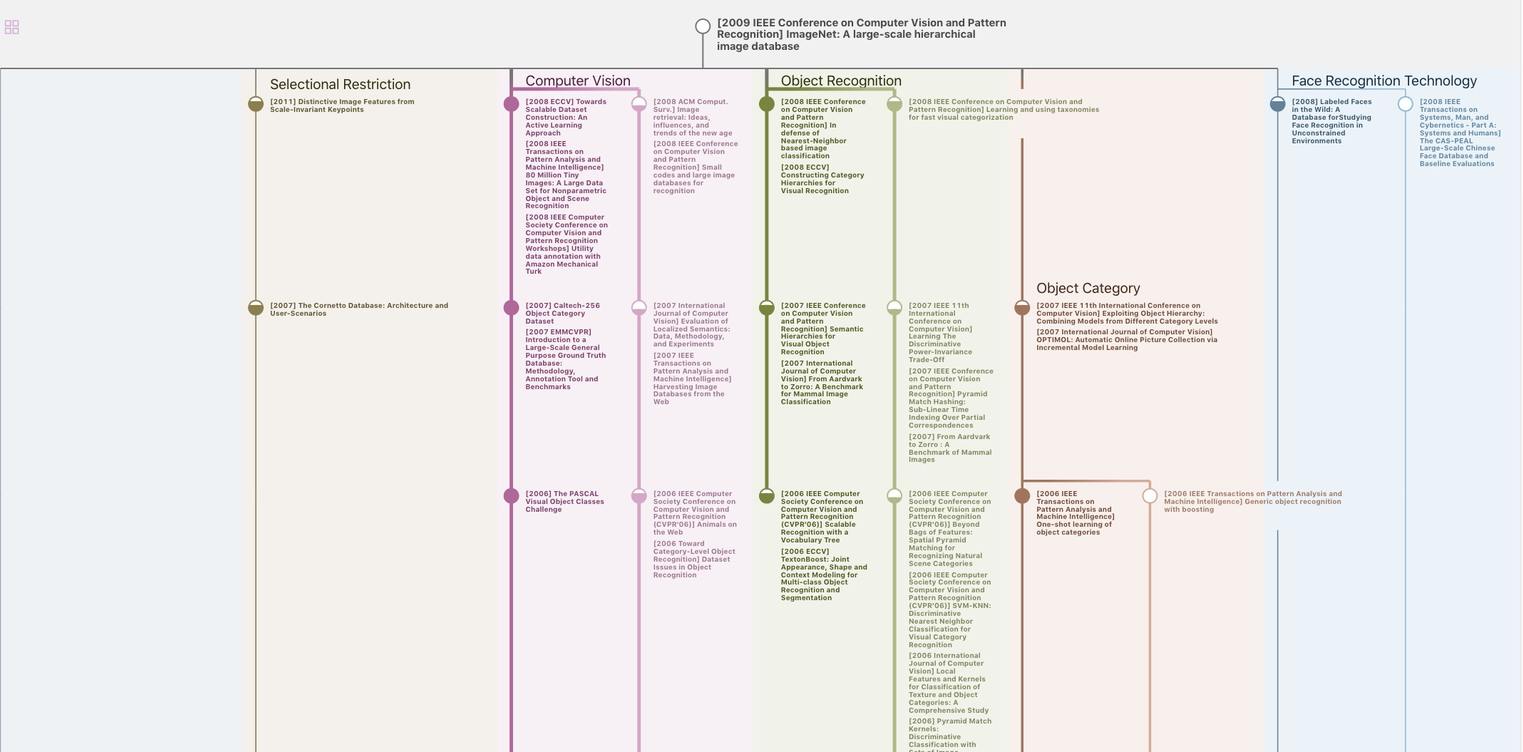
生成溯源树,研究论文发展脉络
Chat Paper
正在生成论文摘要