Learning-based Techniques for Transmitter Localization: A Case Study on Model Robustness
2023 20TH ANNUAL IEEE INTERNATIONAL CONFERENCE ON SENSING, COMMUNICATION, AND NETWORKING, SECON(2023)
摘要
Transmitter localization remains a challenging problem in large-scale outdoor environments, especially when transmitters and receivers are allowed to be mobile. We consider localization in the context of a Radio Dynamic Zone (RDZ), a proposed experimental platform where researchers can deploy experimental devices, waveforms, or wireless networks. Wireless users outside an RDZ must be protected from harmful interference coming from sources inside the RDZ. In this setting, localizing transmitters that are causing interference is critical. One notable obstacle for developing data-driven methods for localization is the lack of large-scale training datasets. As our first contribution, we present a new dataset for localization, captured at 462.7 MHz in a 4 sq. km outdoor area with 29 different receivers and over 4,500 unique transmitter locations. Receivers are both mobile and stationary, and heterogeneous in terms of hardware, placement, and gain settings. Next, we propose a new machine learning-based localization method that can handle inputs from uncalibrated, heterogeneous receivers. Finally, we leverage our new dataset to study the robustness of our technique and others against "out of distribution" (OOD) inputs that are common in most real life applications. We show that our technique, CUTL (Calibrated U-Net Transmitter Localization), is 49% more accurate on in-distribution data, and more robust than previous methods on OOD data.
更多查看译文
关键词
transmitter localization,model robustness,RF spectrum sensing
AI 理解论文
溯源树
样例
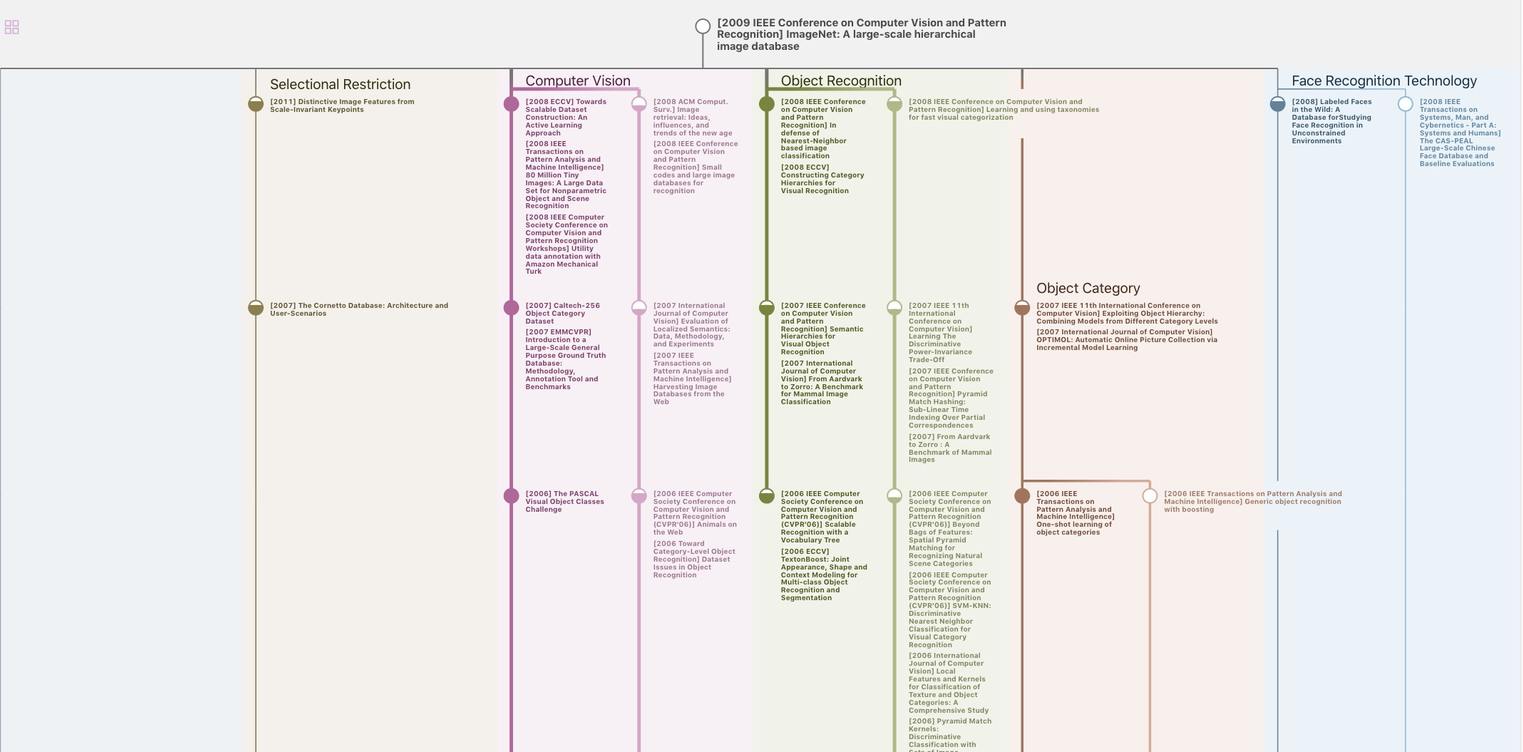
生成溯源树,研究论文发展脉络
Chat Paper
正在生成论文摘要