EVS2vec: A Low-dimensional Embedding Method for Encrypted Video Stream Analysis
2023 20TH ANNUAL IEEE INTERNATIONAL CONFERENCE ON SENSING, COMMUNICATION, AND NETWORKING, SECON(2023)
摘要
The rise in video streaming has led to an increase in network traffic, with encrypted video streams playing a significant role in illegal video detection. However, there are challenges in performing content analysis of encrypted video streams, including label limitations and complex calculations. In this paper, we proposed a low-dimensional embedding method based on Byte Rate Sequences (BRS), named EVS2vec (Encrypted Video Stream to Vector), to solve these problems effectively. It can represent the content of encrypted video streams with low-dimensional vectors by mapping the indefinite-length sequence into a low-dimensional Euclidean space. EVS2vec can thereby be applied for not only supervised analysis but also unsupervised analysis. Furthermore, using BRS can also save the time overhead on fine-grained network traffic parsing. In order to ensure the content-related distinguishability of the embedding result, inspired by contrastive learning, we designed a network structure based on Recurrent Neural Network (RNN) with self-attention mechanism in EVS2vec and trained it using siamese network. The experiments on a public dataset show that EVS2vec saves storage overhead while containing enough video content information. EVS2vec can achieve a high accuracy of similarity threshold, reaching 96.71%. An 8-dimensional fingerprint for each video is constructed. Moreover, classification and clustering analysis can also be performed with acceptable results.
更多查看译文
关键词
Encrypted Video Stream,Embedding,BRS,Side-channel Analysis,DASH
AI 理解论文
溯源树
样例
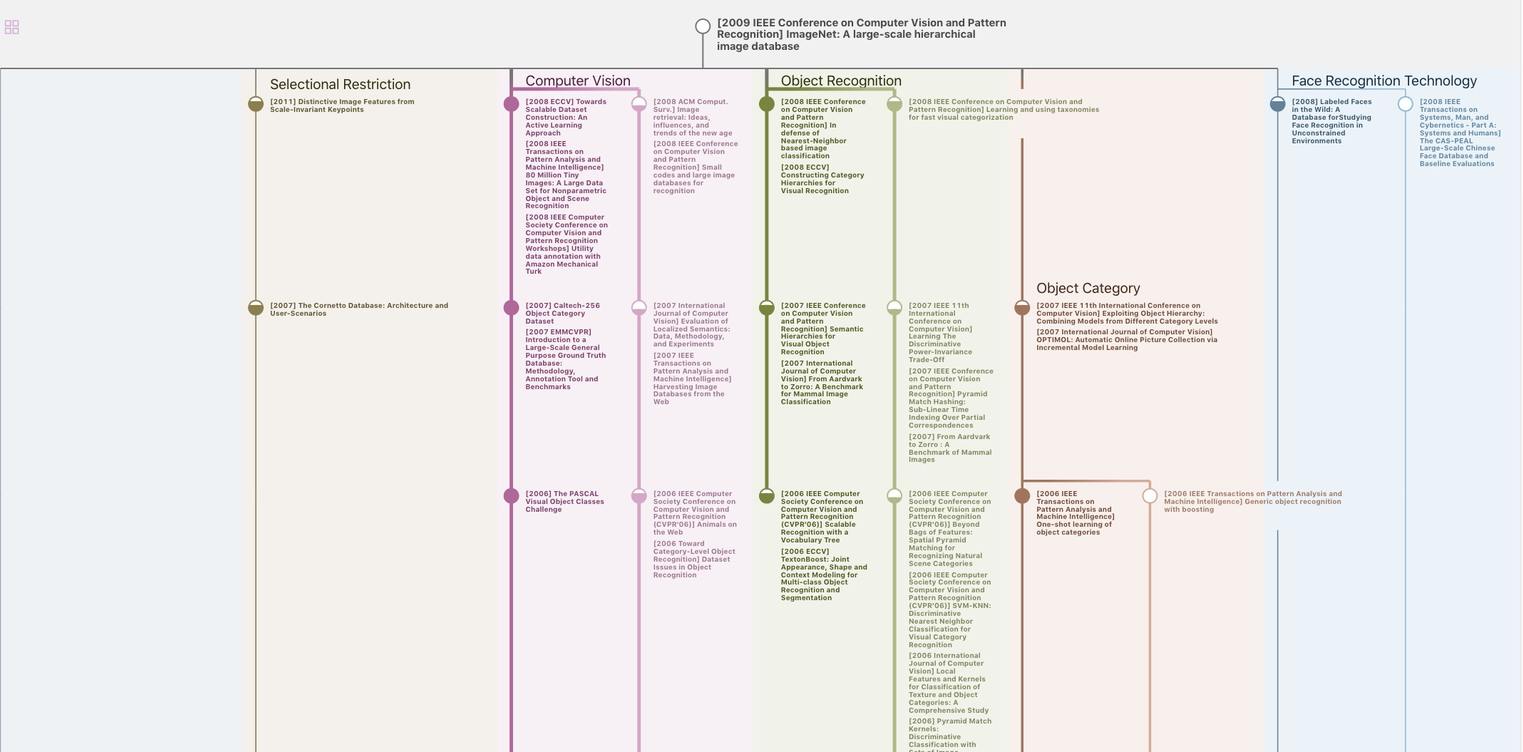
生成溯源树,研究论文发展脉络
Chat Paper
正在生成论文摘要