Unsupervised Domain Adaptation of Universal Source Separation Based on Neural Full-Rank Spatial Covariance Analysis
2023 IEEE 33rd International Workshop on Machine Learning for Signal Processing (MLSP)(2023)
摘要
This paper presents an unsupervised domain adaptation method of universal source separation (USS) using a neural blind source separation (BSS) technique. USS is a task of separating environmental sound mixtures and has been investigated by training a deep neural network in a supervised manner. This approach, however, suffers from the domain mismatch problem between training and test data. In this paper, we propose an unsupervised domain adaptation method based on neural full-rank spatial covariance analysis. In the (pre-)training phase, a neural separation model is trained to perform multichannel Wiener filtering in a supervised manner. In the test phase, on the other hand, the network is fine-tuned to fit the test mixtures in an unsupervised manner by maximizing a log-marginal likelihood of a nonlinear (neural) BSS model. Experimental results with simulated mixture signals show that our method successfully improves the separation performance for test sets without any additional supervision.
更多查看译文
关键词
Neural source separation,domain adaptation,blind source separation,multichannel signal processing
AI 理解论文
溯源树
样例
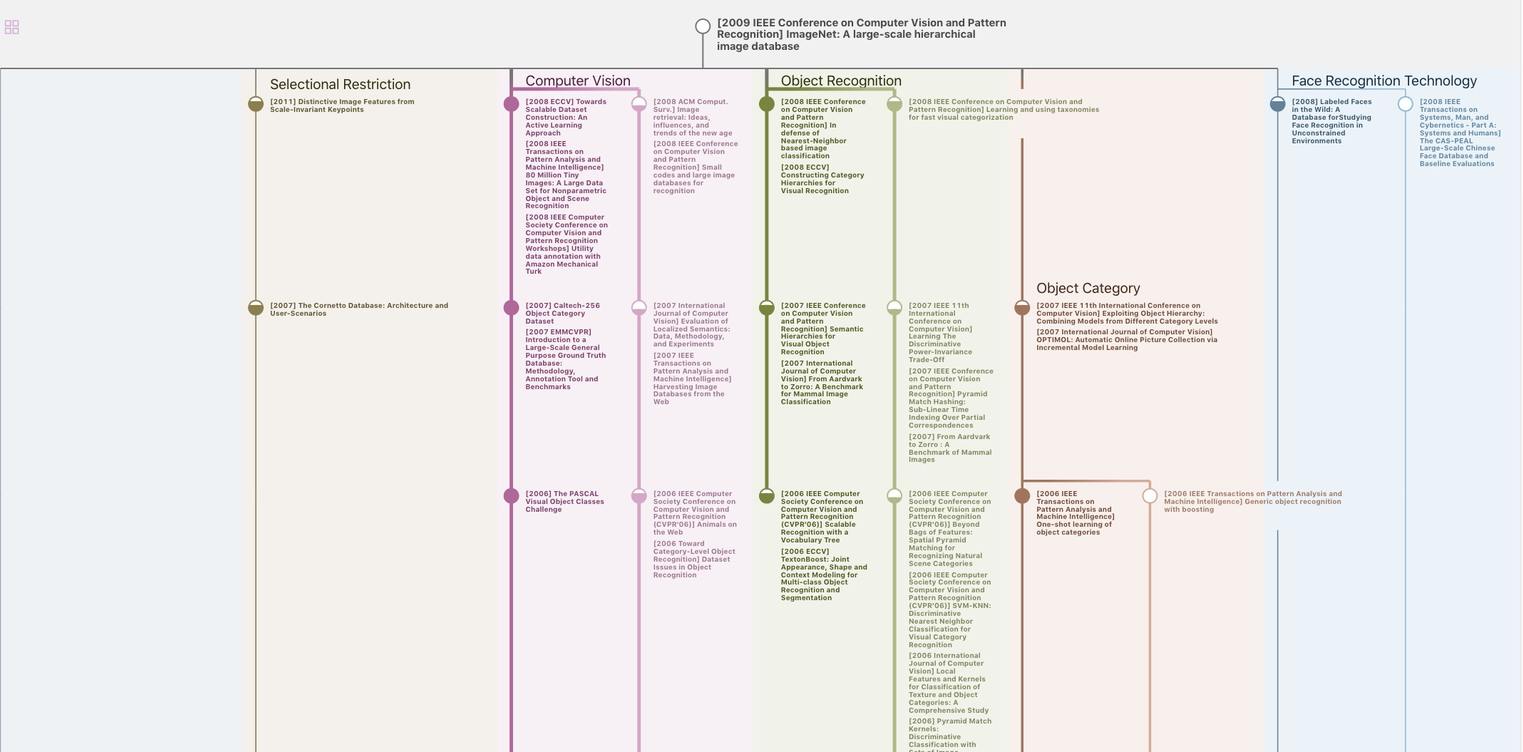
生成溯源树,研究论文发展脉络
Chat Paper
正在生成论文摘要