Federated Representation Learning Through Clustering
2023 IEEE 33rd International Workshop on Machine Learning for Signal Processing (MLSP)(2023)
摘要
Federated self-supervised learning (FedSSL) methods have proven to be very useful in learning unlabeled data that is distributed to multiple clients, possibly heterogeneously. However, there is still a lot of room for improvement for FedSSL methods, especially for the case of highly heterogeneous data and a large number of classes. In this paper, we introduce a new way of thinking to approach the FedSSL problems. Specifically, we propose optimizing the representations through the more difficult task of clustering. The resulting federated representation learning through clustering (FedRLC) scheme utilizes i) a crossed KL divergence loss with a data selection strategy during local training and ii) a dynamic upload on local cluster centers during communication updates. Experimental results show that FedRLC achieves state-of-the-art results on widely used benchmarks even with highly heterogeneous settings and datasets with a large number of classes such as CIFAR-100.
更多查看译文
关键词
Federated learning,self-supervised representation learning,clustering,KL divergence
AI 理解论文
溯源树
样例
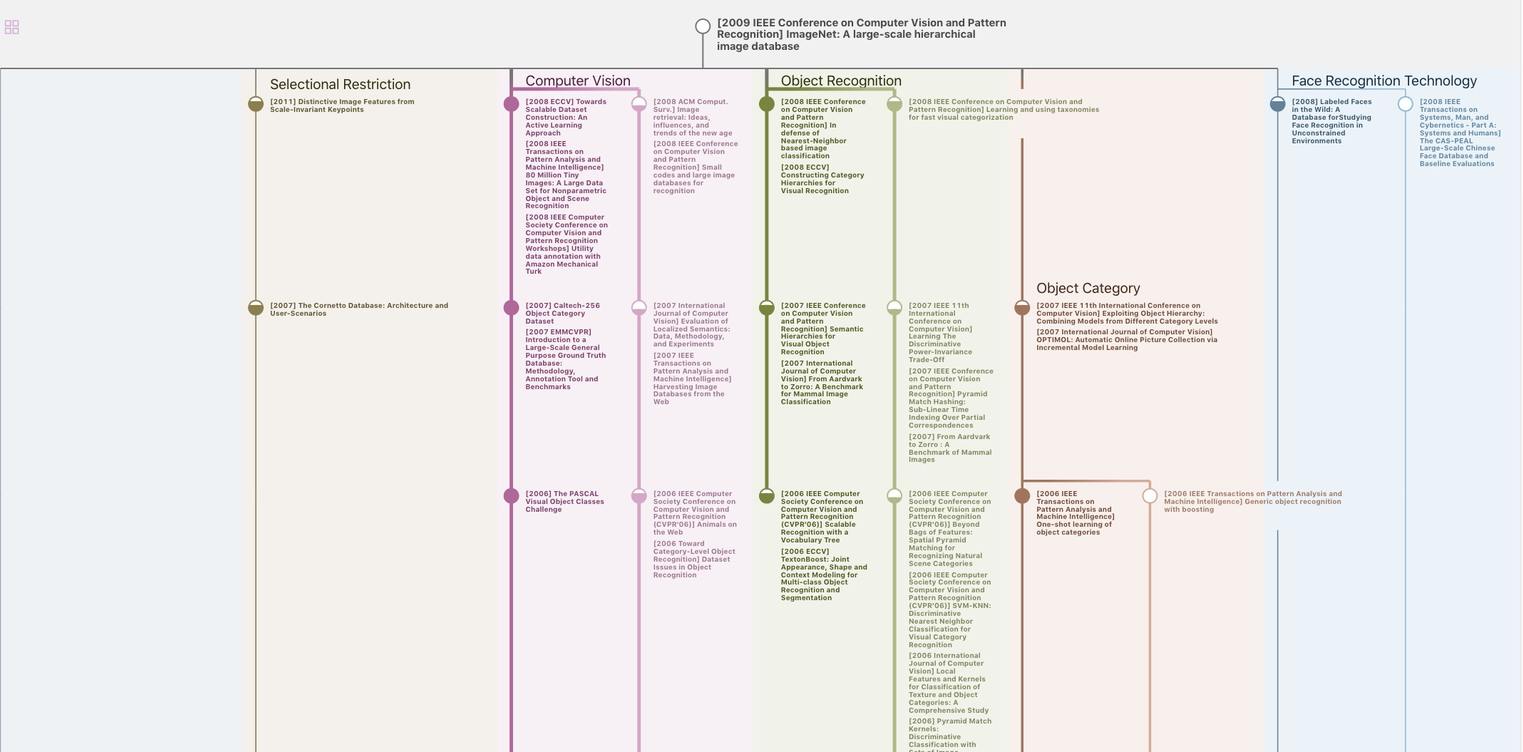
生成溯源树,研究论文发展脉络
Chat Paper
正在生成论文摘要