Stream-Based Active Learning with Adaptive Uncertainty and Diversity Thresholds
2023 IEEE 33rd International Workshop on Machine Learning for Signal Processing (MLSP)(2023)
Abstract
This paper proposes Stream-based Active Learning method using Adaptive uncertainty and Diversity Thresholds (SALAT), which aims to reduce the amount of data required for labeling in stream-based data settings while accounting for concept drift. The proposed method uses adaptive uncertainty and diversity thresholds, which are monitored by a sliding window of data selection history to ensure that the data selection is neither too conservative nor too aggressive. The effectiveness of SALAT is validated on the MNIST and FashionMNIST datasets, where it achieves similar performance to the baseline with fewer training samples. The experiments also confirm the hypothesis that a fixed threshold approach is deficient, and an adaptive threshold significantly reduces the data requirement to reach an adequate level of performance. The paper shows that SRLRT can handle concept drift effectively, making it applicable to various real-world applications such as text classification, image classification, and speech recognition.
MoreTranslated text
Key words
active learning,data selection,stream-based data,neural networks
AI Read Science
Must-Reading Tree
Example
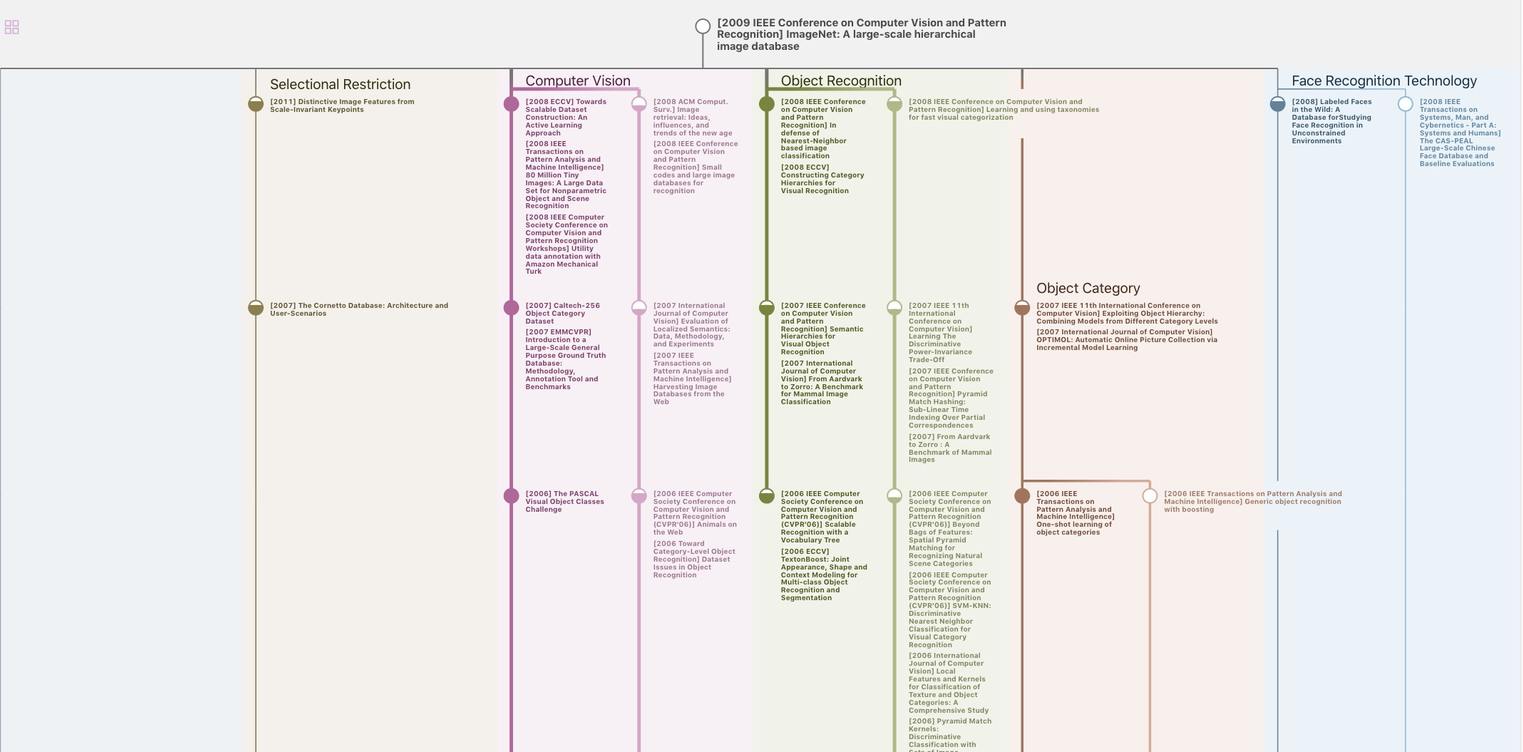
Generate MRT to find the research sequence of this paper
Chat Paper
Summary is being generated by the instructions you defined