Rapidly-exploring random tree-based obstacle-aware mobile sink trajectory for data collection in wireless sensor networks
Journal of Ambient Intelligence and Humanized Computing(2024)
摘要
Wireless sensor networks (WSNs) have embraced mobile sink-based data collection for a decade due to its advantages in addressing sinkhole or energy hole problems, as well as collecting data from disjoint nodes. Several algorithms are introduced in the literature to address mobile sink-based algorithms for WSNs. However, they do not focus on the obstacles contained in WSNs. In this context, we propose RRTMT which performs efficient RP selection and obstacle-aware path planning for a mobile sink in WSNs with obstacles. A spectral clustering algorithm is used to identify the most effective RPs in which the hop count between the RPs and their children is minimal. Next, we determine the obstacle-aware path between the RPs using a rapidly-explored random tree (RRT) to adapt to the environment dynamically. We conduct the simulation through Python under different scenarios and various metrics. The proposed RRTMT outperforms the existing but related obstacle-sensitive mobile sink data collection algorithms by approximately 14-26
更多查看译文
关键词
Wireless sensor networks,Mobile sinks,Rapidly-exploring random tree,Spectral clustering
AI 理解论文
溯源树
样例
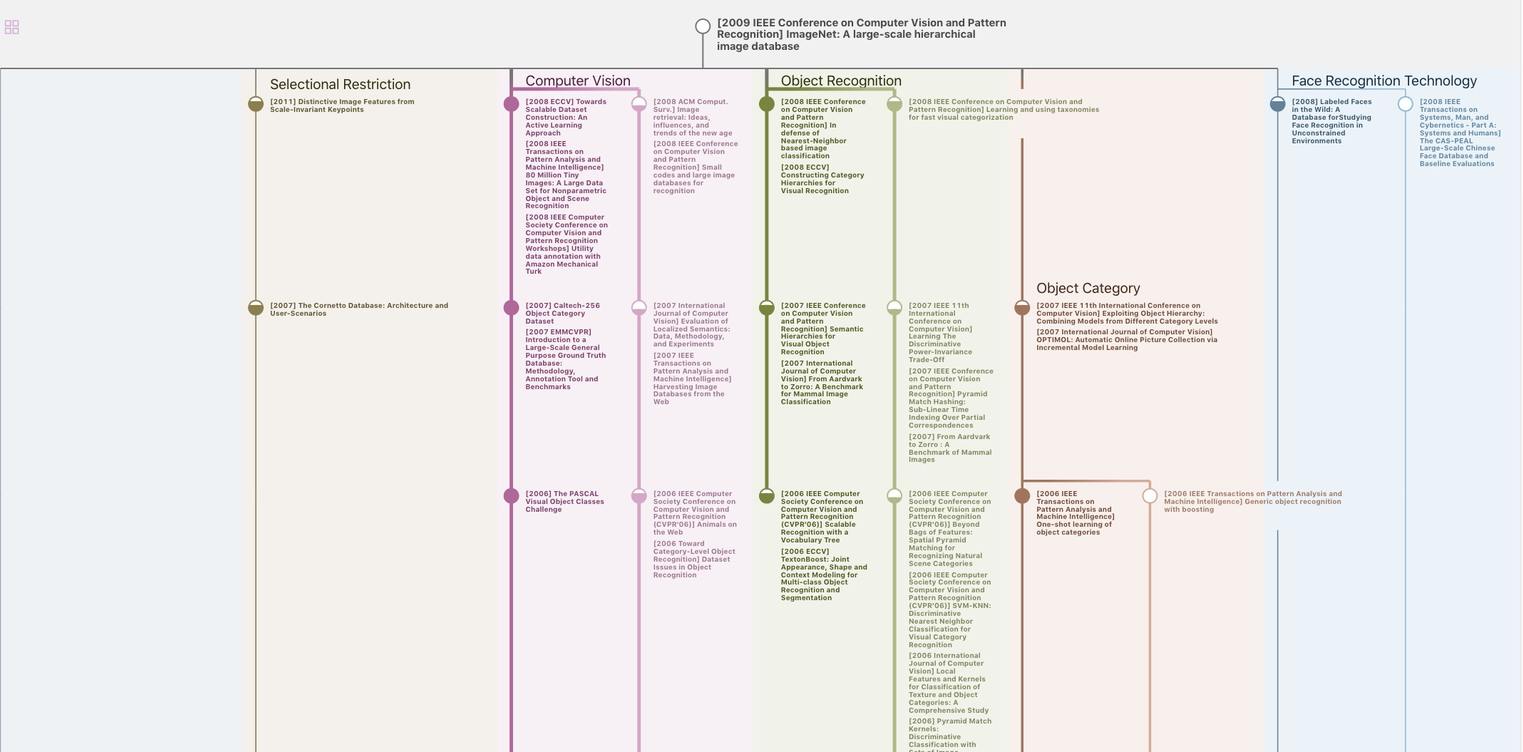
生成溯源树,研究论文发展脉络
Chat Paper
正在生成论文摘要