CoSP: co-selection pick for a global explainability of black box machine learning models
WORLD WIDE WEB-INTERNET AND WEB INFORMATION SYSTEMS(2023)
摘要
Recently, few methods for understanding machine learning model’s outputs have been developed. SHAP and LIME are two well-known examples of these methods. They provide individual explanations based on feature importance for each instance. While remarkable scores have been achieved for individual explanations, understanding the model’s decisions globally remains a complex task. Methods like LIME were extended to face this complexity by using individual explanations. In this approach, the problem was expressed as a submodular optimization problem. This algorithm is a bottom-up method aiming at providing a global explanation. It consists of picking a group of individual explanations which illustrate the global behavior of the model and avoid redundancy. In this paper, we propose CoSP (Co-Selection Pick) framework that allows a global explainability of any black-box model by selecting individual explanations based on a similarity preserving approach. Unlike submodular optimization, in our method the problem is considered as a co-selection task. This approach achieves a co-selection of instances and features over the explanations provided by any explainer. The proposed framework is more generic given that it is possible to make the co-selection either in supervised or unsupervised scenarios and also over explanations provided by any local explainer. Preliminary experimental results are made to validate our proposal.
更多查看译文
关键词
Machine learning models,Explicability,Local explanation and global aggregation
AI 理解论文
溯源树
样例
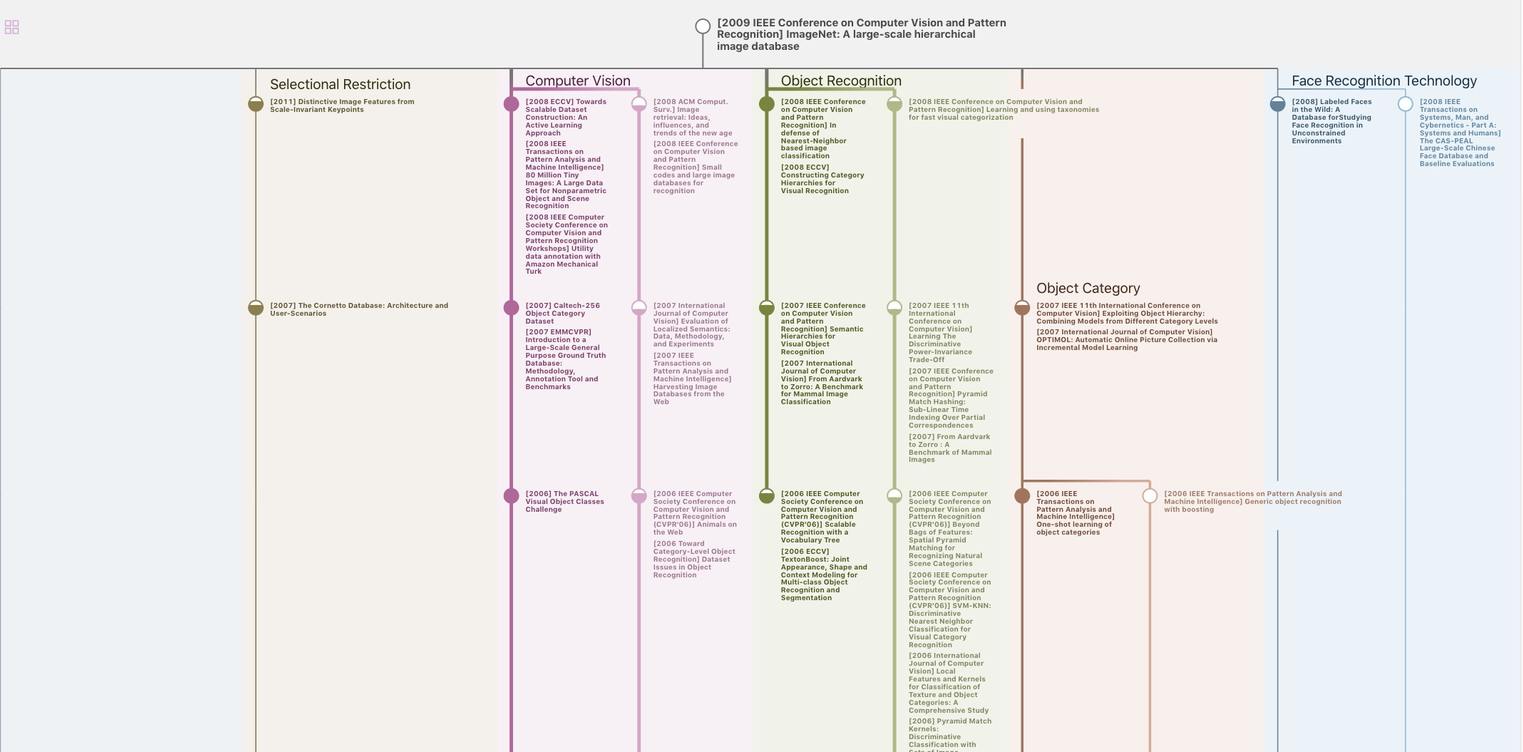
生成溯源树,研究论文发展脉络
Chat Paper
正在生成论文摘要