Forensic discrimination between traditional and compressive imaging by blurring kernel investigation
Multimedia Tools and Applications(2023)
摘要
Abstract Image forensics encompasses a set of scientific tests to investigation of a suspected event via intrinsic clues of imaging pipeline. Traditional image sensing at the Nyquist-Shannon rate as well as the new modality of compressive imaging below the rate are two main types of sensing in photography and imaging applications. Hence, for forensic investigators, it would importantly necessitate the ability to discriminate among images captured by them. However, due to the complex nonlinear nature of imaging processes, investigating imagers’ traces is a difficult task. To this intent, we first systematically model the imaging pipelines as an encoder-decoder pair. For exploring distinguishable traces, we mathematically simplify and linearize the pair for compressive imaging and two main forms of traditional image sensing with or without compression. Our theoretical analyses on the approximate linear models reveal blurring kernels of different imagers have discriminability. To validate it in real-world scenarios, we considered the whole imaging process as an inverse problem and estimated the blurring kernel based on a deconvolution approach, where the discriminability is also justified by information visualization. Then, we designed a pipeline classification system, where a deep convolutional neural network is trained by the estimated blurring kernels to be able to classify the three imaging systems. Our results in compressive imaging identification show an accuracy improvement about 3.7 % in comparison to the best result among compared methods. Implementation codes are available for research and development.
更多查看译文
关键词
Compressive sensing,Convolutional neural network,Data compression,Deconvolution,Image forensics,Imaging,Systems modeling
AI 理解论文
溯源树
样例
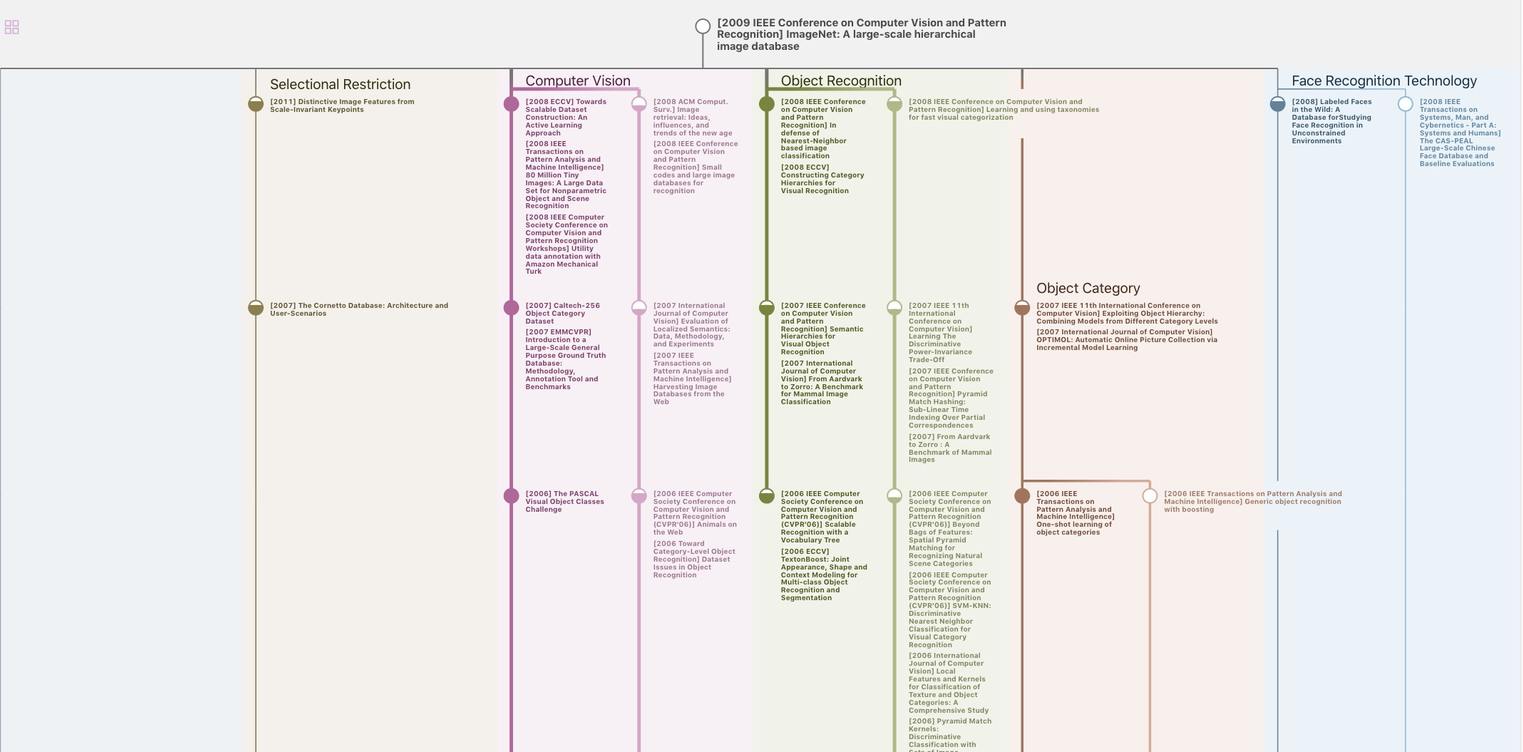
生成溯源树,研究论文发展脉络
Chat Paper
正在生成论文摘要