A Multi-strategy Improved Sparrow Search Algorithm and its Application
Neural Processing Letters(2023)
Abstract
In order to address the issues of slow convergence and susceptibility to falling into the local optimum trap of the original sparrow search algorithm, a novel multi-strategy improved sparrow search algorithm (MSSSA) is proposed. Firstly, an improved tent chaotic mapping is introduced to enhance the diversity and quality of the initial population distribution. Secondly, an adaptive adjustment strategy of population division is incorporated to balance the global search and local exploitation capabilities of algorithm. Furthermore, To improve the convergence performance, the sinusoidal function is applied to update the explorer and vigilant. Finally, an adaptive perturbation strategy is proposed to assist the algorithm in escaping local optimal solutions. To evaluate the effectiveness of the proposed improved strategy, 13 classical test functions and the CEC2017 test suite were selected to validate the performance of MSSSA. the Friedman test and Wilcoxon test results also verify the significance of the results, the effectiveness and convergence of the improved strategy. In addition, the improved algorithm was applied to predict the medium-term electricity load of the microgrid, and the parameters of the gated recurrent unit neural network were optimally predicted on two actual electricity load datasets. The experimental comparison further confirms the effectiveness and feasibility of the proposed improved algorithm in practical applications.
MoreTranslated text
Key words
algorithm,search,multi-strategy
AI Read Science
Must-Reading Tree
Example
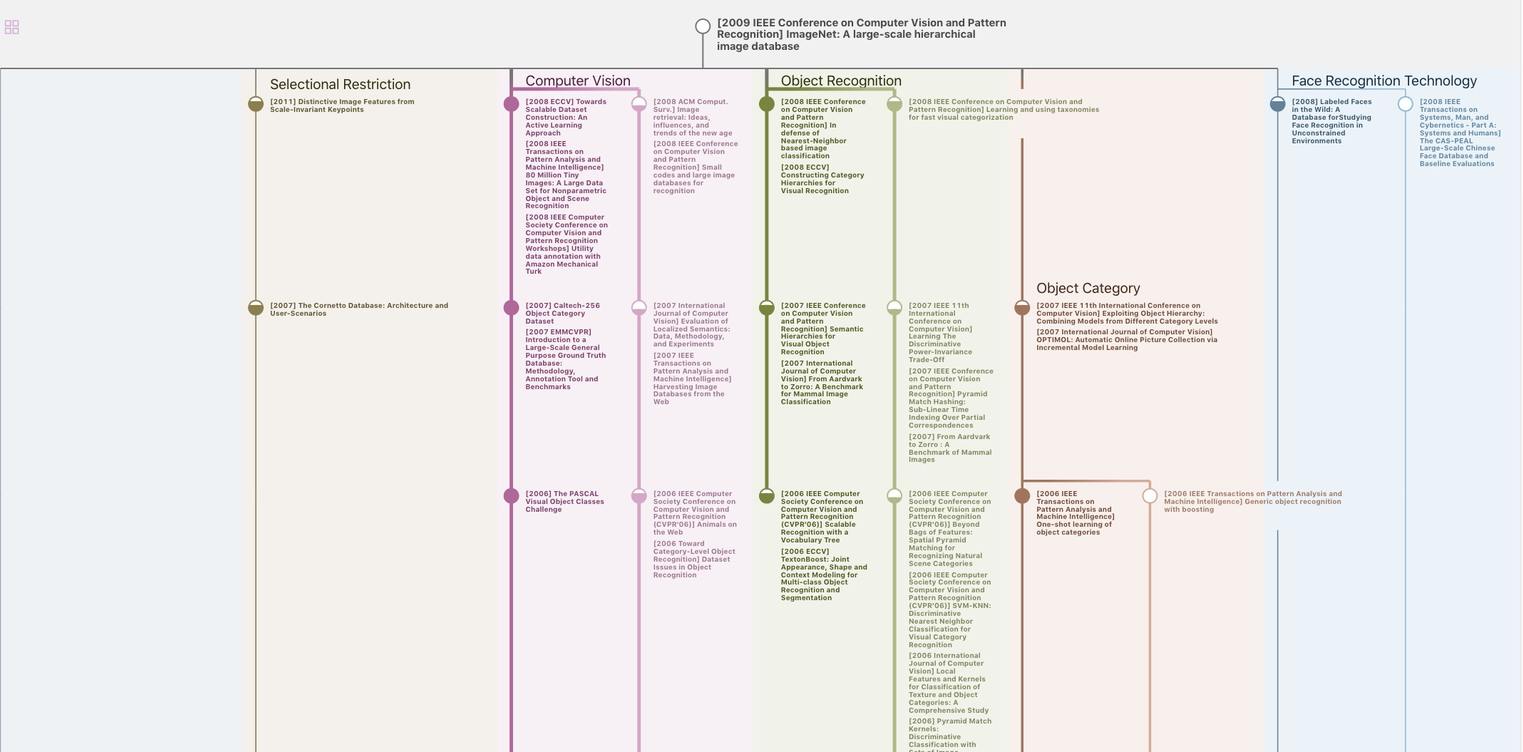
Generate MRT to find the research sequence of this paper
Chat Paper
Summary is being generated by the instructions you defined