SCL-IKD: intermediate knowledge distillation via supervised contrastive representation learning
Applied Intelligence(2023)
Abstract
Knowledge distillation, which extracts dark knowledge from a deep teacher model to drive the learning of a shallow student model, is helpful in several tasks, including model compression and regularization. While previous research has focused on architecture-driven solutions for extracting information from the teacher models, these solutions are focused on a single task and fail to extract rich dark knowledge from large teacher networks in the presence of capacity gaps for broader applications. Hence, in this paper, we propose a supervised contrastive learning-based intermediate knowledge distillation (SCL-IKD) technique that is more effective in distilling knowledge from teacher networks to train a student model for classification tasks. SCL-IKD, unlike other approaches, is model agnostic and may be used in a variety of teacher-student cross-architectures. Investigations on several datasets reveal that SCL-IKD can achieve 3-4% better top-1 accuracy over several state-of-the-art baselines. Furthermore, compared to the baselines, SCL-IKD is found better to handle capacity gaps between teacher and student models and is significantly more robust to symmetric noisy labels and data availability.
MoreTranslated text
Key words
Knowledge distillation,Image classification,Supervised contrastive learning,Dark knowledge extraction,Deep learning
AI Read Science
Must-Reading Tree
Example
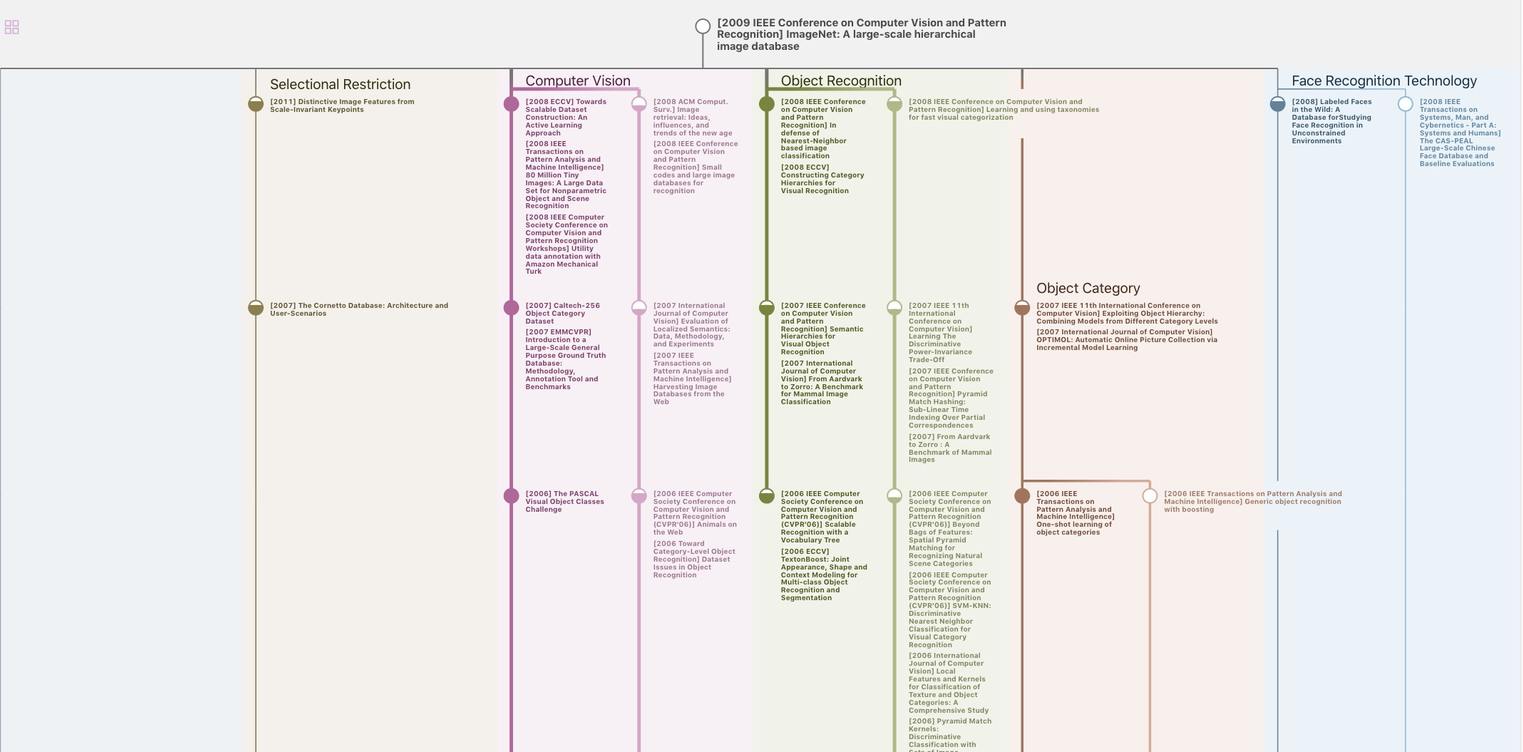
Generate MRT to find the research sequence of this paper
Chat Paper
Summary is being generated by the instructions you defined