Improved K-mer Based Prediction of Protein-Protein Interactions With Chaos Game Representation, Deep Learning and Reduced Representation Bias
CoRR(2023)
摘要
Protein-protein interactions drive many biological processes, including the detection of phytopathogens by plants' R-Proteins and cell surface receptors. Many machine learning studies have attempted to predict protein-protein interactions but performance is highly dependent on training data; models have been shown to accurately predict interactions when the proteins involved are included in the training data, but achieve consistently poorer results when applied to previously unseen proteins. In addition, models that are trained using proteins that take part in multiple interactions can suffer from representation bias, where predictions are driven not by learned biological features but by learning of the structure of the interaction dataset. We present a method for extracting unique pairs from an interaction dataset, generating non-redundant paired data for unbiased machine learning. After applying the method to datasets containing _Arabidopsis thaliana_ and pathogen effector interations, we developed a convolutional neural network model capable of learning and predicting interactions from Chaos Game Representations of proteins' coding genes.
更多查看译文
关键词
chaos game representation,deep learning,k-mer,protein-protein
AI 理解论文
溯源树
样例
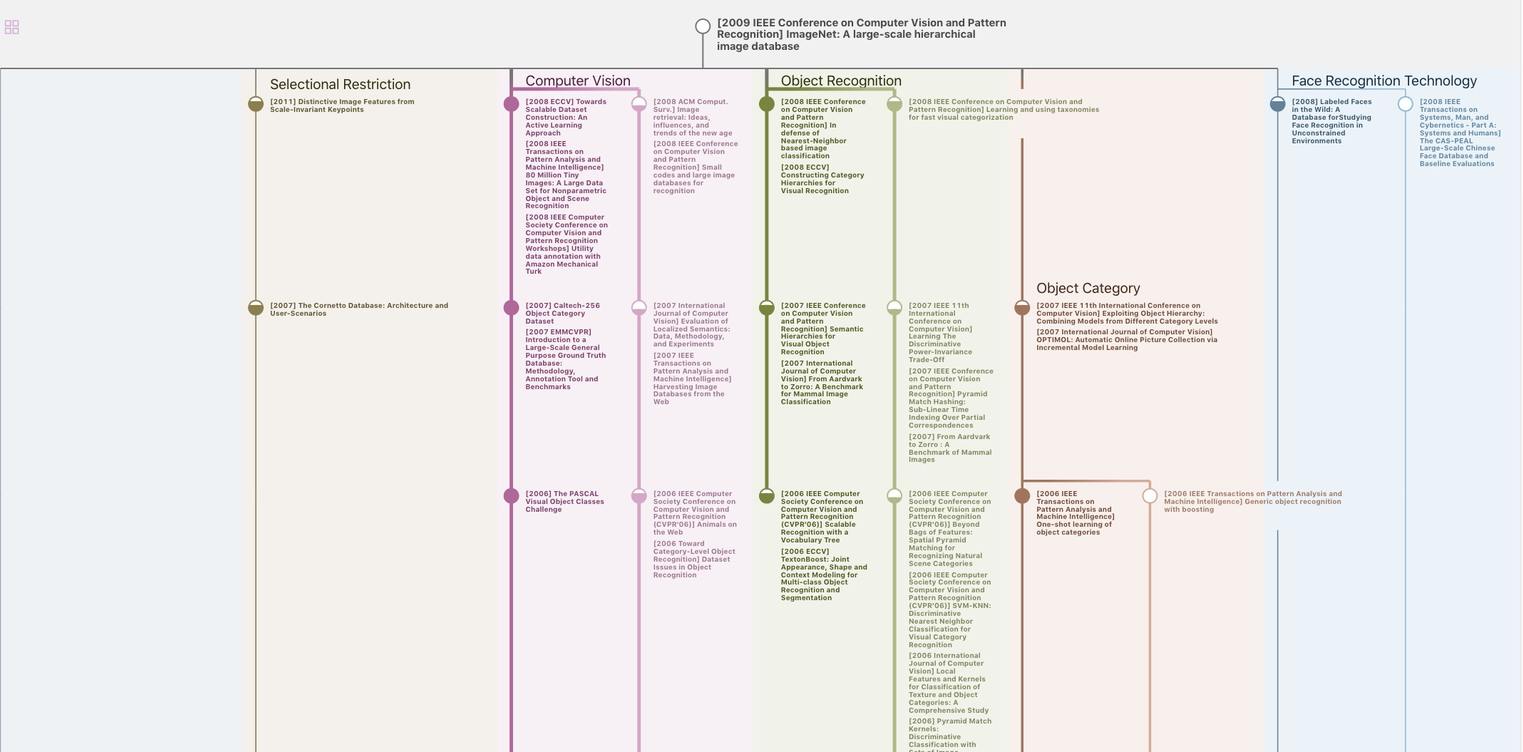
生成溯源树,研究论文发展脉络
Chat Paper
正在生成论文摘要