Engineered dissipation to mitigate barren plateaus
arXiv (Cornell University)(2023)
Abstract
Variational quantum algorithms represent a powerful approach for solving optimization problems on noisy quantum computers, with a broad spectrum of potential applications ranging from chemistry to machine learning. However, their performances in practical implementations crucially depend on the effectiveness of quantum circuit training, which can be severely limited by phenomena such as barren plateaus. While, in general, dissipation is detrimental for quantum algorithms, and noise itself can actually induce barren plateaus, here we describe how the inclusion of properly engineered Markovian losses after each unitary quantum circuit layer can restore the trainability of quantum models. We identify the required form of the dissipation processes and establish that their optimization is efficient. We benchmark our proposal in both a synthetic and a practical quantum chemistry example, demonstrating its effectiveness and potential impact across different domains.
MoreTranslated text
Key words
barren plateaus,dissipation
AI Read Science
Must-Reading Tree
Example
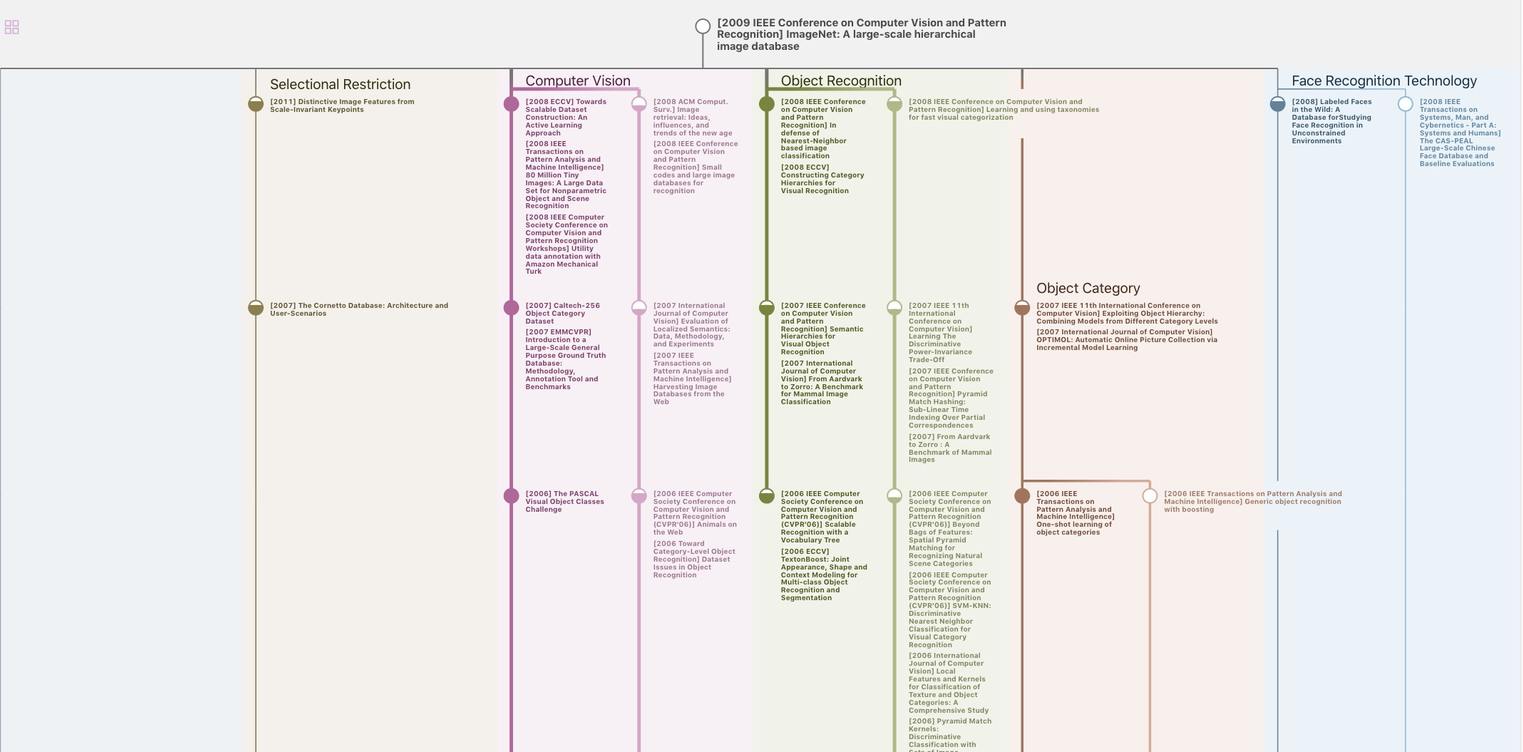
Generate MRT to find the research sequence of this paper
Chat Paper
Summary is being generated by the instructions you defined