SpEL: Structured Prediction for Entity Linking.
CoRR(2023)
摘要
Entity linking is a prominent thread of research focused on structured data creation by linking spans of text to an ontology or knowledge source. We revisit the use of structured prediction for entity linking which classifies each individual input token as an entity, and aggregates the token predictions. Our system, called SpEL (Structured prediction for Entity Linking) is a state-of-the-art entity linking system that uses some new ideas to apply structured prediction to the task of entity linking including: two refined fine-tuning steps; a context sensitive prediction aggregation strategy; reduction of the size of the model's output vocabulary, and; we address a common problem in entity-linking systems where there is a training vs. inference tokenization mismatch. Our experiments show that we can outperform the state-of-the-art on the commonly used AIDA benchmark dataset for entity linking to Wikipedia. Our method is also very compute efficient in terms of number of parameters and speed of inference.
更多查看译文
关键词
structured prediction
AI 理解论文
溯源树
样例
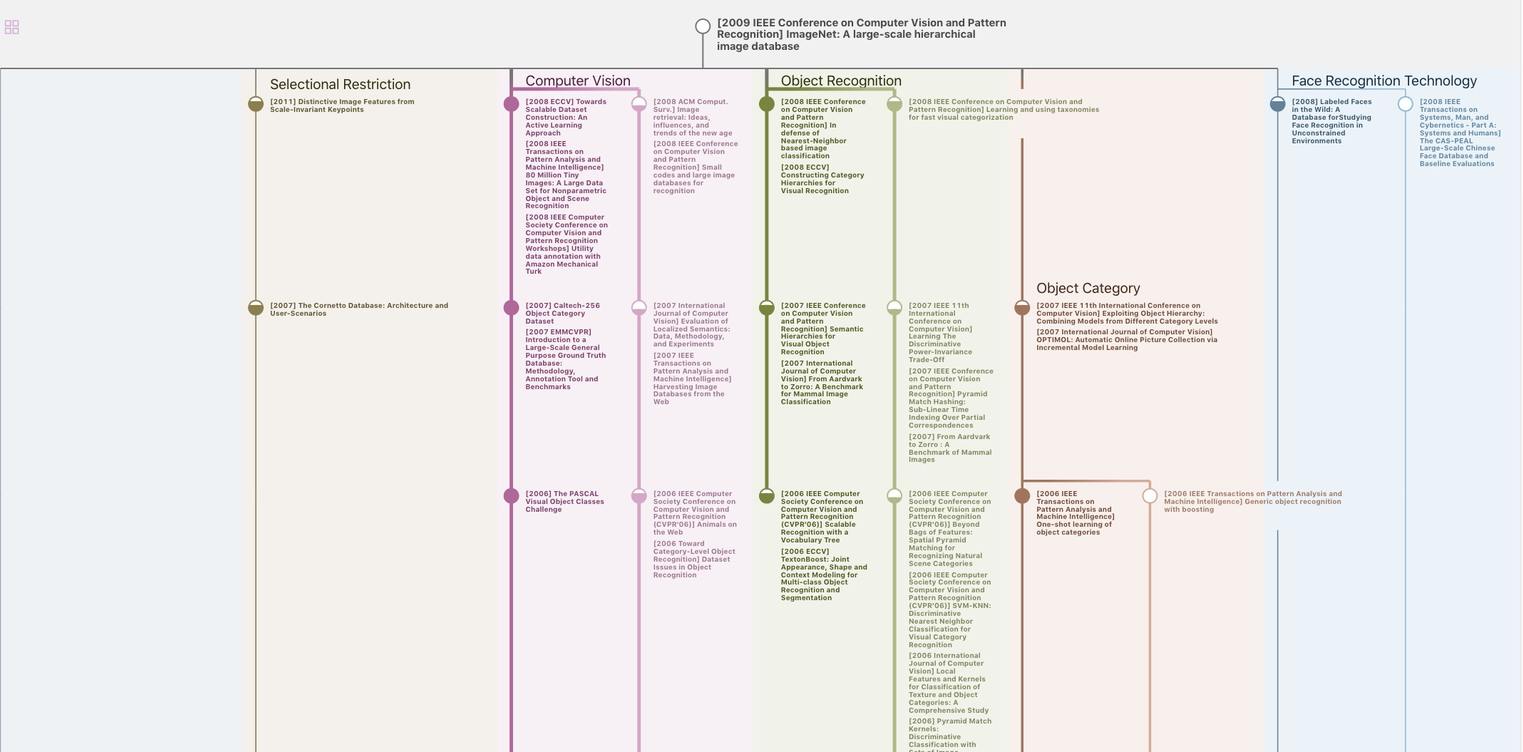
生成溯源树,研究论文发展脉络
Chat Paper
正在生成论文摘要