CAD-DA: Controllable Anomaly Detection after Domain Adaptation by Statistical Inference
CoRR(2023)
摘要
We propose a novel statistical method for testing the results of anomaly detection (AD) under domain adaptation (DA), which we call CAD-DA -- controllable AD under DA. The distinct advantage of the CAD-DA lies in its ability to control the probability of misidentifying anomalies under a pre-specified level $\alpha$ (e.g., 0.05). The challenge within this DA setting is the necessity to account for the influence of DA to ensure the validity of the inference results. Our solution to this challenge leverages the concept of conditional Selective Inference to handle the impact of DA. To our knowledge, this is the first work capable of conducting a valid statistical inference within the context of DA. We evaluate the performance of the CAD-DA method on both synthetic and real-world datasets.
更多查看译文
关键词
controllable anomaly detection,domain adaptation,anomaly detection
AI 理解论文
溯源树
样例
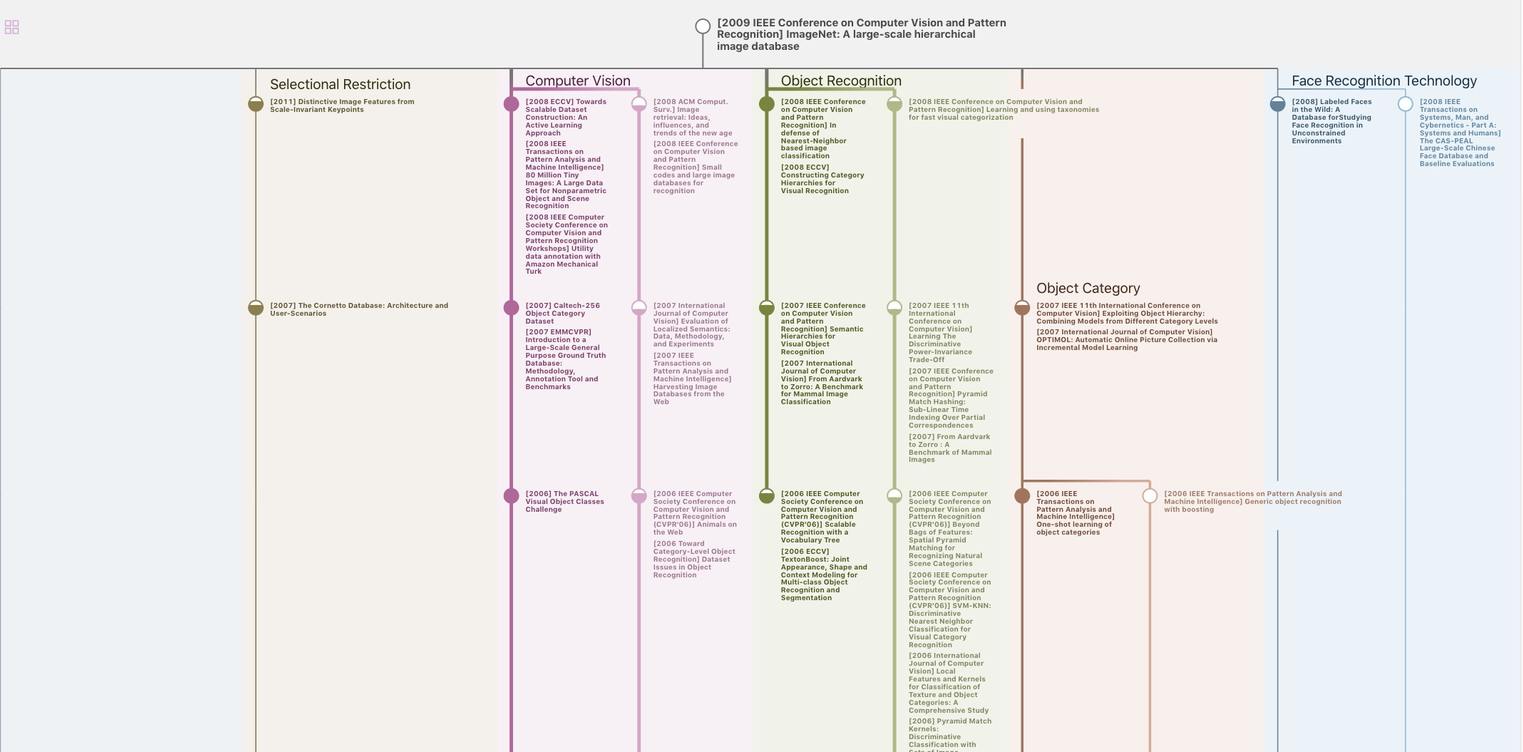
生成溯源树,研究论文发展脉络
Chat Paper
正在生成论文摘要