Separating multiscale Battery dynamics and predicting multi-step ahead voltage simultaneously through a data-driven approach
2023 IEEE VEHICLE POWER AND PROPULSION CONFERENCE, VPPC(2023)
摘要
Accurate prediction of battery performance under various ageing conditions is necessary for reliable and stable battery operations. Due to complex battery degradation mechanisms, estimating the accurate ageing level and ageing-dependent battery dynamics is difficult. This work presents a health-aware battery model that is capable of separating fast dynamics from slowly varying states of degradation and state of charge (SOC). The method is based on a sequence to sequence learning-based encoder-decoder model, where the encoder infers the slowly varying states as the latent space variables in an unsupervised way, and the decoder provides health-aware multi-step ahead prediction conditioned on slowly varying states from the encoder. The proposed approach is verified on a Lithium-ion battery ageing dataset based on real driving profiles of electric vehicles.
更多查看译文
关键词
battery,multiscale dynamics,machine learning
AI 理解论文
溯源树
样例
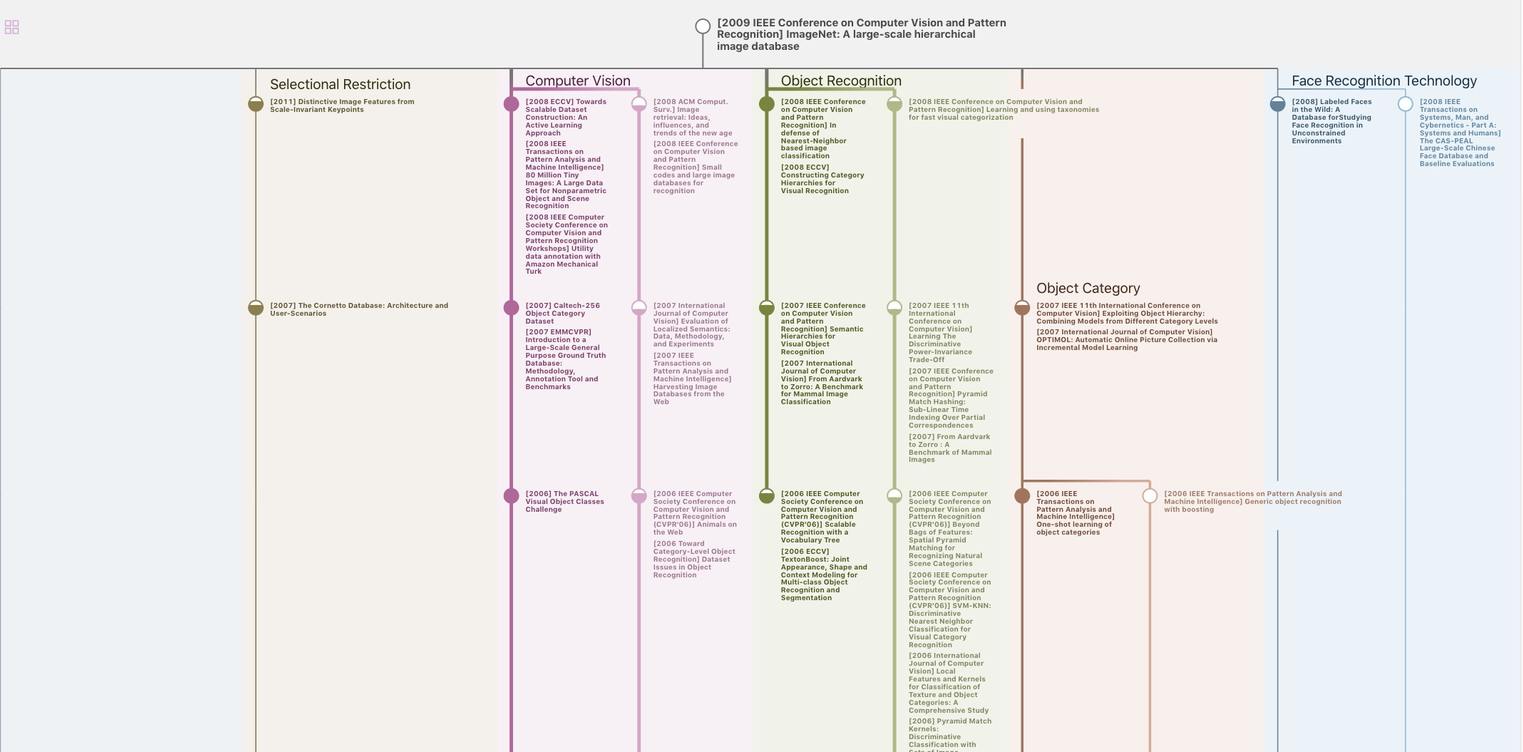
生成溯源树,研究论文发展脉络
Chat Paper
正在生成论文摘要