Can Language Models Laugh at YouTube Short-form Videos?
CoRR(2023)
摘要
As short-form funny videos on social networks are gaining popularity, it becomes demanding for AI models to understand them for better communication with humans. Unfortunately, previous video humor datasets target specific domains, such as speeches or sitcoms, and mostly focus on verbal cues. We curate a user-generated dataset of 10K multimodal funny videos from YouTube, called ExFunTube. Using a video filtering pipeline with GPT-3.5, we verify both verbal and visual elements contributing to humor. After filtering, we annotate each video with timestamps and text explanations for funny moments. Our ExFunTube is unique over existing datasets in that our videos cover a wide range of domains with various types of humor that necessitate a multimodal understanding of the content. Also, we develop a zero-shot video-to-text prompting to maximize video humor understanding of large language models (LLMs). With three different evaluation methods using automatic scores, rationale quality experiments, and human evaluations, we show that our prompting significantly improves LLMs' ability for humor explanation.
更多查看译文
关键词
language models laugh,language models,short-form
AI 理解论文
溯源树
样例
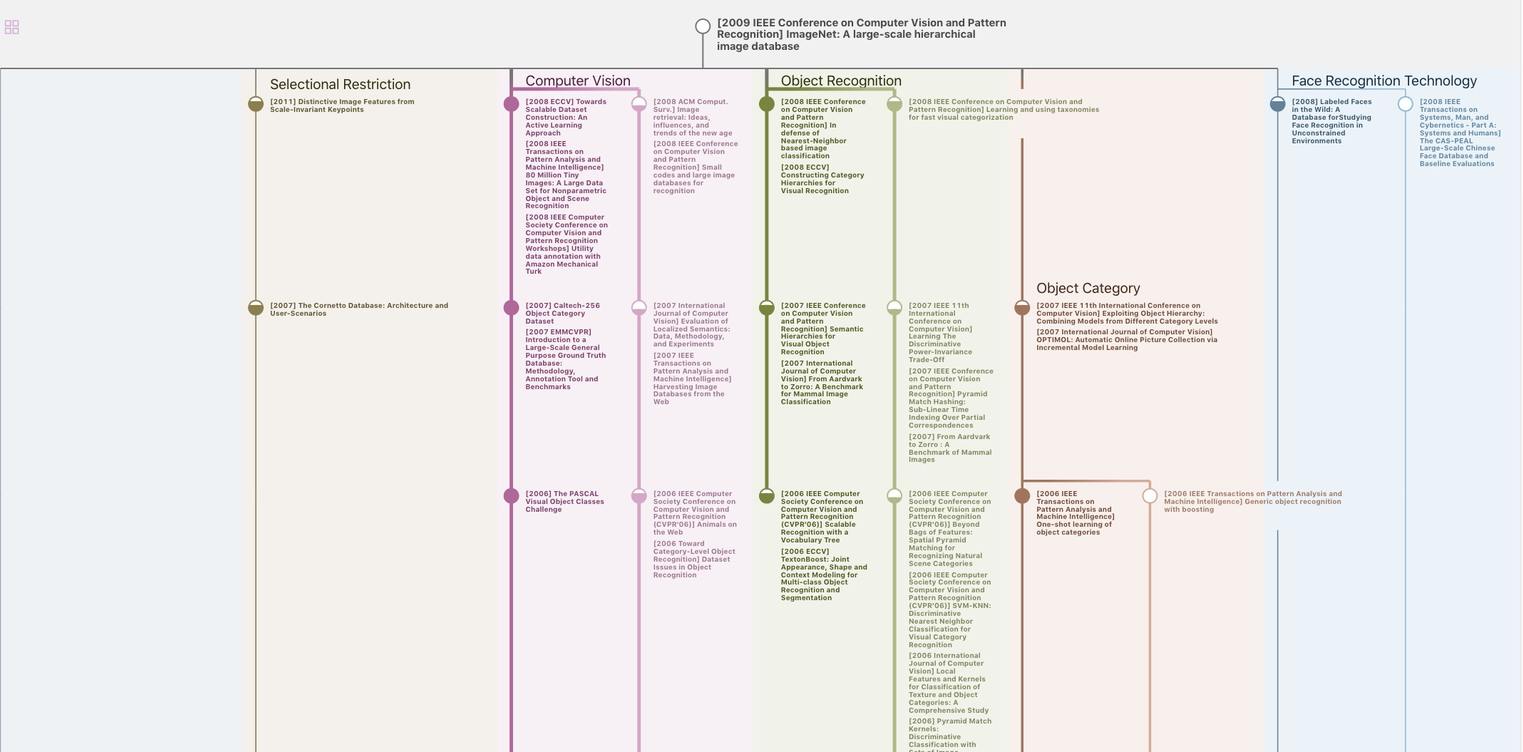
生成溯源树,研究论文发展脉络
Chat Paper
正在生成论文摘要