A Better Match for Drivers and Riders: Reinforcement Learning at Lyft
INFORMS JOURNAL ON APPLIED ANALYTICS(2024)
摘要
To better match drivers to riders in our ridesharing application, we revised Lyft's core matching algorithm. We use a novel online reinforcement learning approach that estimates the future earnings of drivers in real time, and we use this information to find more efficient matches. This change was the first documented implementation of a ridesharing matching algorithm that can learn and improve in real time. We evaluated the new approach during weeks of switchback experimentation in most Lyft markets and estimated how it benefited drivers, riders, and the platform. In particular, it enabled our drivers to serve millions of additional riders each year, leading to more than $30 million per year in incremental revenue. Lyft rolled out the algorithm globally in 2021.
更多查看译文
关键词
Edelman award,reinforcement learning,ridesharing,optimization,experimentation,transportation
AI 理解论文
溯源树
样例
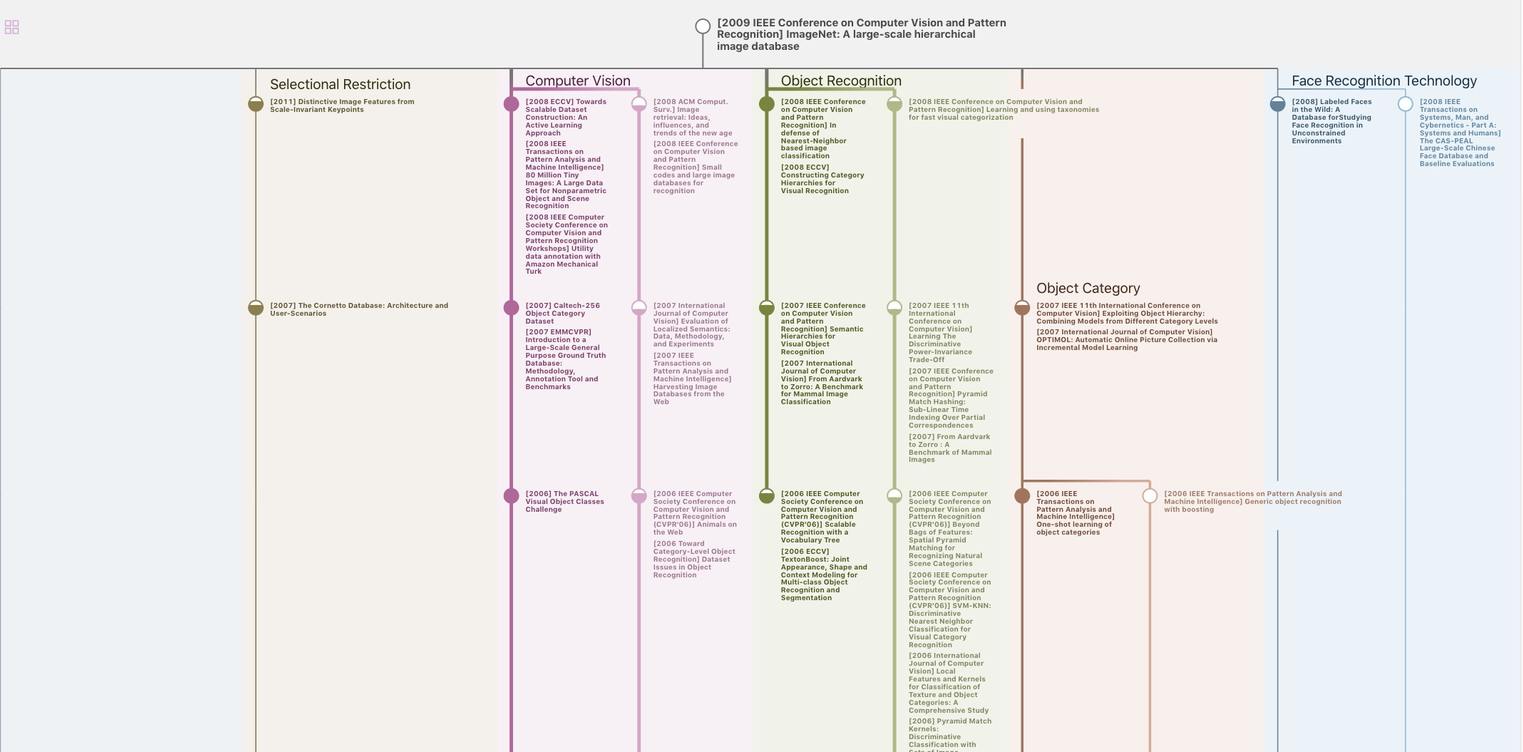
生成溯源树,研究论文发展脉络
Chat Paper
正在生成论文摘要