Epidemiology-aware Deep Learning for Infectious Disease Dynamics Prediction
PROCEEDINGS OF THE 32ND ACM INTERNATIONAL CONFERENCE ON INFORMATION AND KNOWLEDGE MANAGEMENT, CIKM 2023(2023)
摘要
Infectious disease risk prediction plays a vital role in disease control and prevention. Recent studies in machine learning have attempted to incorporate epidemiological knowledge into the learning process to enhance the accuracy and informativeness of prediction results for decision-making. However, these methods commonly involve single-patch mechanistic models, overlooking the disease spread across multiple locations caused by human mobility. Additionally, these methods often require extra information beyond the infection data, which is typically unavailable in reality. To address these issues, this paper proposes a novel epidemiology-aware deep learning framework that integrates a fundamental epidemic component, the next-generation matrix (NGM), into the deep architecture and objective function. This integration enables the inclusion of both mechanistic models and human mobility in the learning process to characterize within- and cross-location disease transmission. From this framework, two novel methods, Epi-CNNRNN-Res and Epi-Cola-GNN, are further developed to predict epidemics, with experimental results validating their effectiveness.
更多查看译文
关键词
Epidemiological constraints,Deep learning,Infectious disease dynamics prediction
AI 理解论文
溯源树
样例
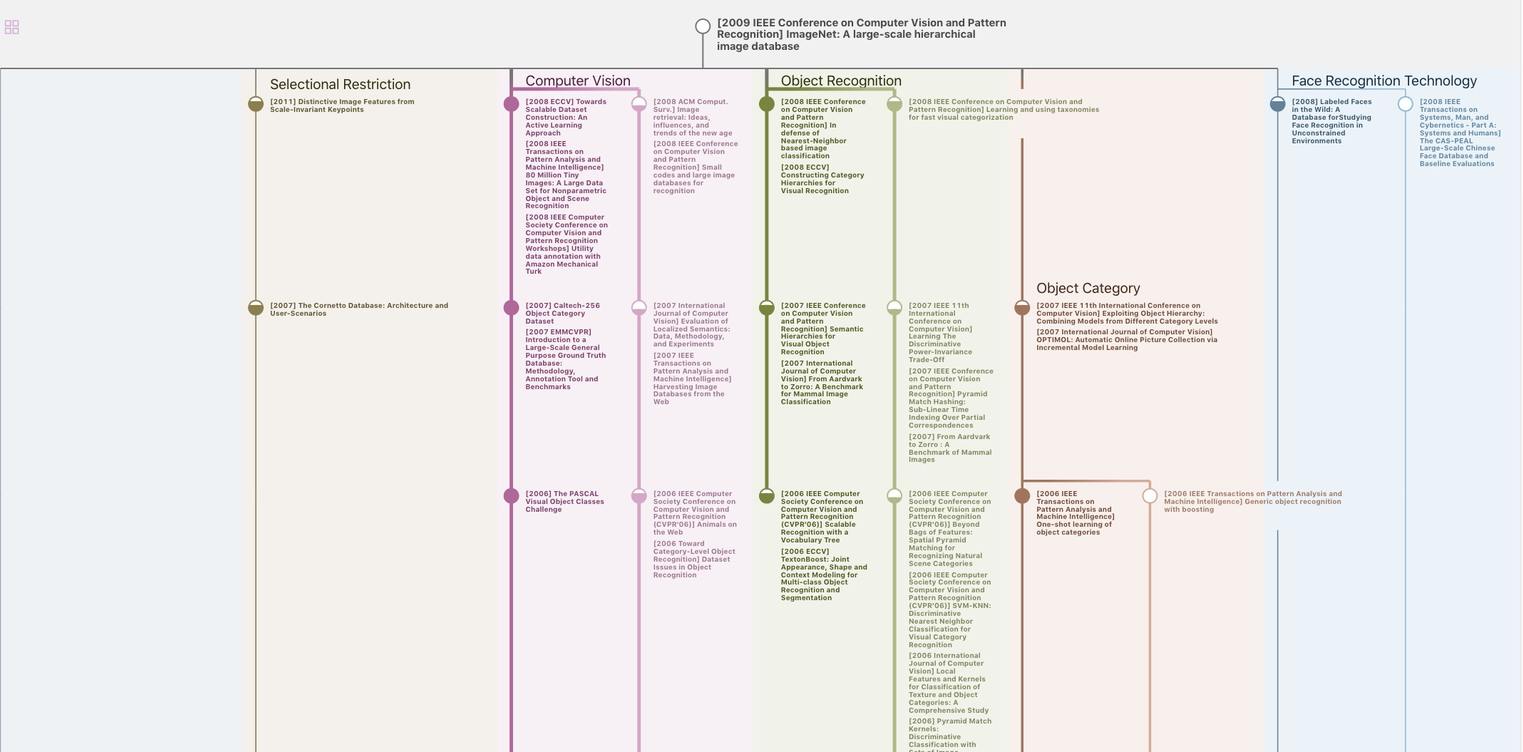
生成溯源树,研究论文发展脉络
Chat Paper
正在生成论文摘要