Can Embeddings Analysis Explain Large Language Model Ranking?
PROCEEDINGS OF THE 32ND ACM INTERNATIONAL CONFERENCE ON INFORMATION AND KNOWLEDGE MANAGEMENT, CIKM 2023(2023)
摘要
Understanding the behavior of deep neural networks for Information Retrieval (IR) is crucial to improve trust in these effective models. Current popular approaches to diagnose the predictions made by deep neural networks are mainly based on: i) the adherence of the retrieval model to some axiomatic property of the IR system, ii) the generation of free-text explanations, or iii) feature importance attributions. In this work, we propose a novel approach that analyzes the changes of document and query embeddings in the latent space and that might explain the inner workings of IR large pre-trained language models. In particular, we focus on predicting query/document relevance, and we characterize the predictions by analyzing the topological arrangement of the embeddings in their latent space and their evolution while passing through the layers of the network. We show that there exists a link between the embedding adjustment and the predicted score, based on how tokens cluster in the embedding space. This novel approach, grounded in the query and document tokens interplay over the latent space, provides a new perspective on neural ranker explanation and a promising strategy for improving the efficiency of the models and Query Performance Prediction (QPP).
更多查看译文
关键词
Explainable Artificial Intelligence,Large Language Models,Embeddings Analysis,Text Ranking
AI 理解论文
溯源树
样例
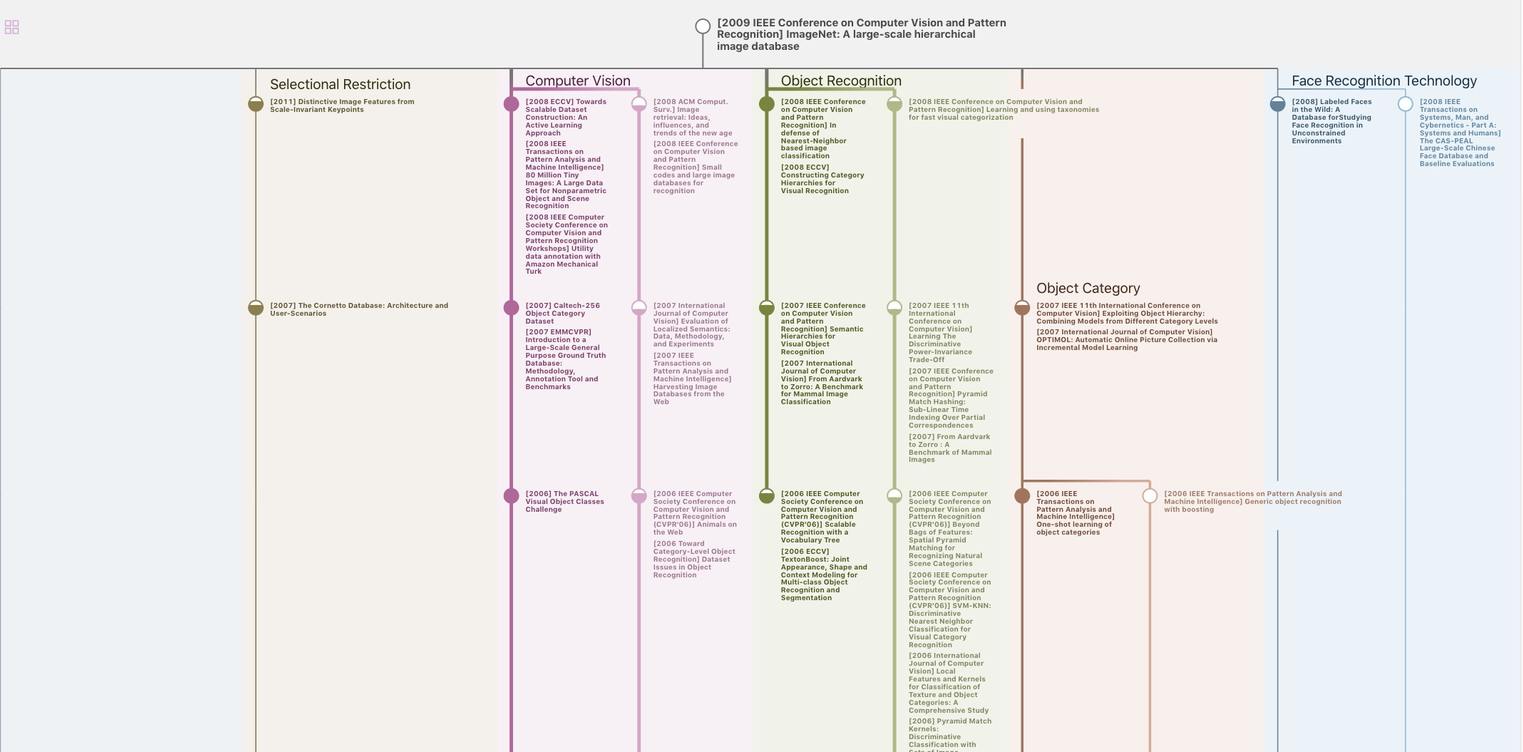
生成溯源树,研究论文发展脉络
Chat Paper
正在生成论文摘要