Enhancing E-commerce Product Search through Reinforcement Learning-Powered Query Reformulation
PROCEEDINGS OF THE 32ND ACM INTERNATIONAL CONFERENCE ON INFORMATION AND KNOWLEDGE MANAGEMENT, CIKM 2023(2023)
摘要
Query reformulation (QR) is a widely used technique in web and product search. In QR, we map a poorly formed or low coverage user query to a few semantically similar queries that are rich in product coverage, thereby enabling effective targeted searches with less cognitive load on the user. Recent QR approaches based on generative language models are superior to informational retrieval-based methods but exhibit key limitations: (i) generated reformulations often have low lexical diversity and fail to retrieve a large set of relevant products of a wider variety, (ii) the training objective of generative models does not incorporate a our goal of improving product coverage. In this paper, we propose RLQR (Reinforcement Learning for Query Reformulations), for generating high quality diverse reformulations which aim to maximize the product coverage (number of distinct relevant products returned). We evaluate our approach against supervised generative models and strong RL-based methods. Our experiments demonstrate a 28.6% increase in product coverage compared to a standard generative model, outperforming SOTA benchmarks by a significant margin. We also conduct our experiments on an external Amazon shopping dataset and demonstrate increased product coverage over SOTA algorithms.
更多查看译文
关键词
Query Reformulation,Natural Language Generation,Reinforcement Learning,E-Commerce,Product Search
AI 理解论文
溯源树
样例
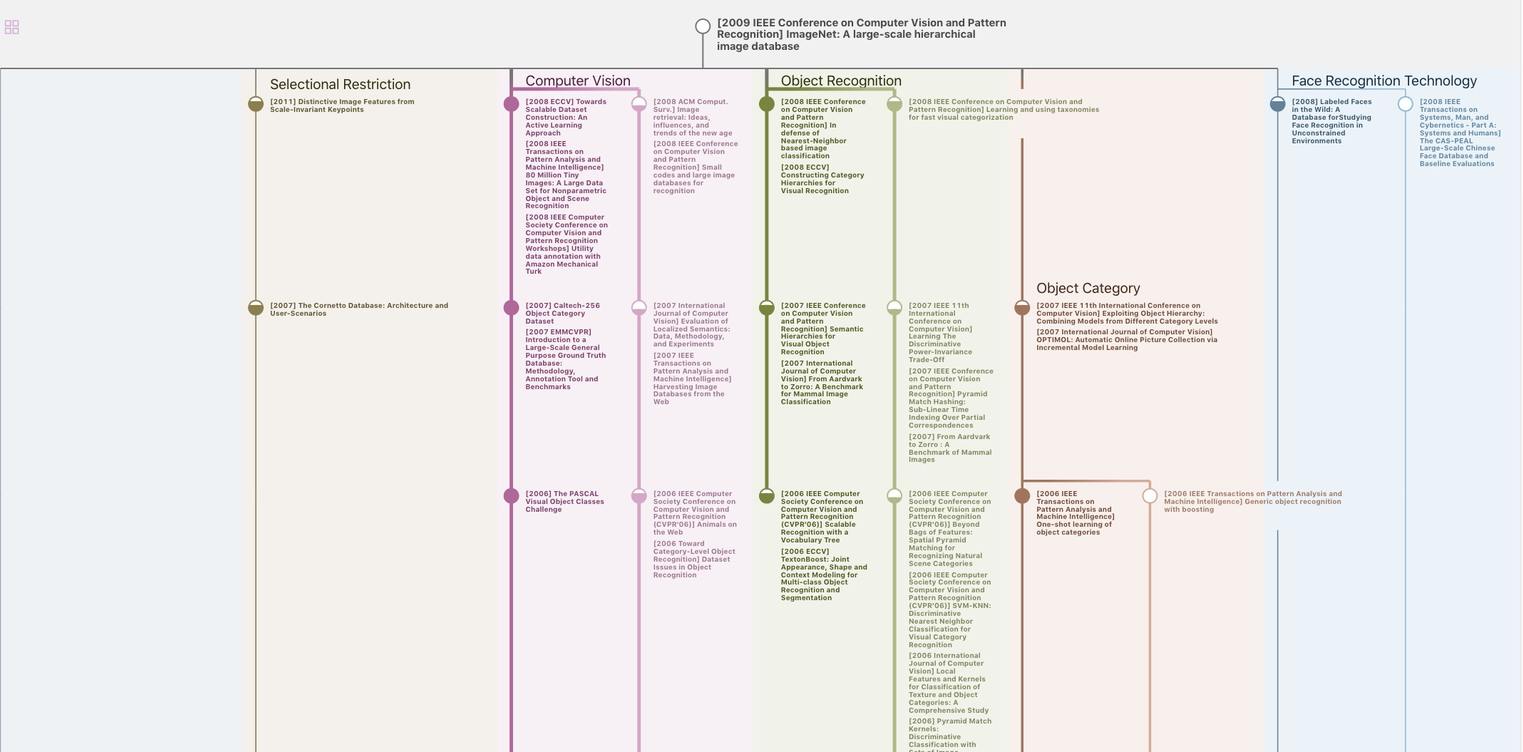
生成溯源树,研究论文发展脉络
Chat Paper
正在生成论文摘要