Investigating Natural and Artificial Dynamics in Graph Data Mining and Machine Learning
PROCEEDINGS OF THE 32ND ACM INTERNATIONAL CONFERENCE ON INFORMATION AND KNOWLEDGE MANAGEMENT, CIKM 2023(2023)
摘要
The complexity of relationships between entities is increasing in the era of big data, leading to a growing interest in graph (network) data, owing to its ability to encode intricate relational information. Graph data mining and machine learning methods extract informative node and graph representations to support broad applications, which have been proven effective for various high-impact tasks in the fields such as computer vision, natural language processing, and recommendation systems. Despite their effectiveness, graph data mining and machine learning methods face practical challenges in real-world scenarios. First, the input graphs may change over time, making it necessary to integrate time-evolving information to enhance representation capabilities. Second, the input graphs may contain unreliable, noisy, or sub-optimal information, requiring researchers and practitioners to intentionally modify the graph topology and node features to improve downstream performance. Facing these two phenomena, our research works focus on natural and artificial dynamics for benefiting graph data mining and machine learning. In this paper, we will briefly introduce our recent works in investigative natural and artificial dynamics and point out some under-explored research problems in the interaction of these dynamics.
更多查看译文
关键词
Graph Data Mining,Graph Machine Learning,Temporal Graphs,Graph Augmentation
AI 理解论文
溯源树
样例
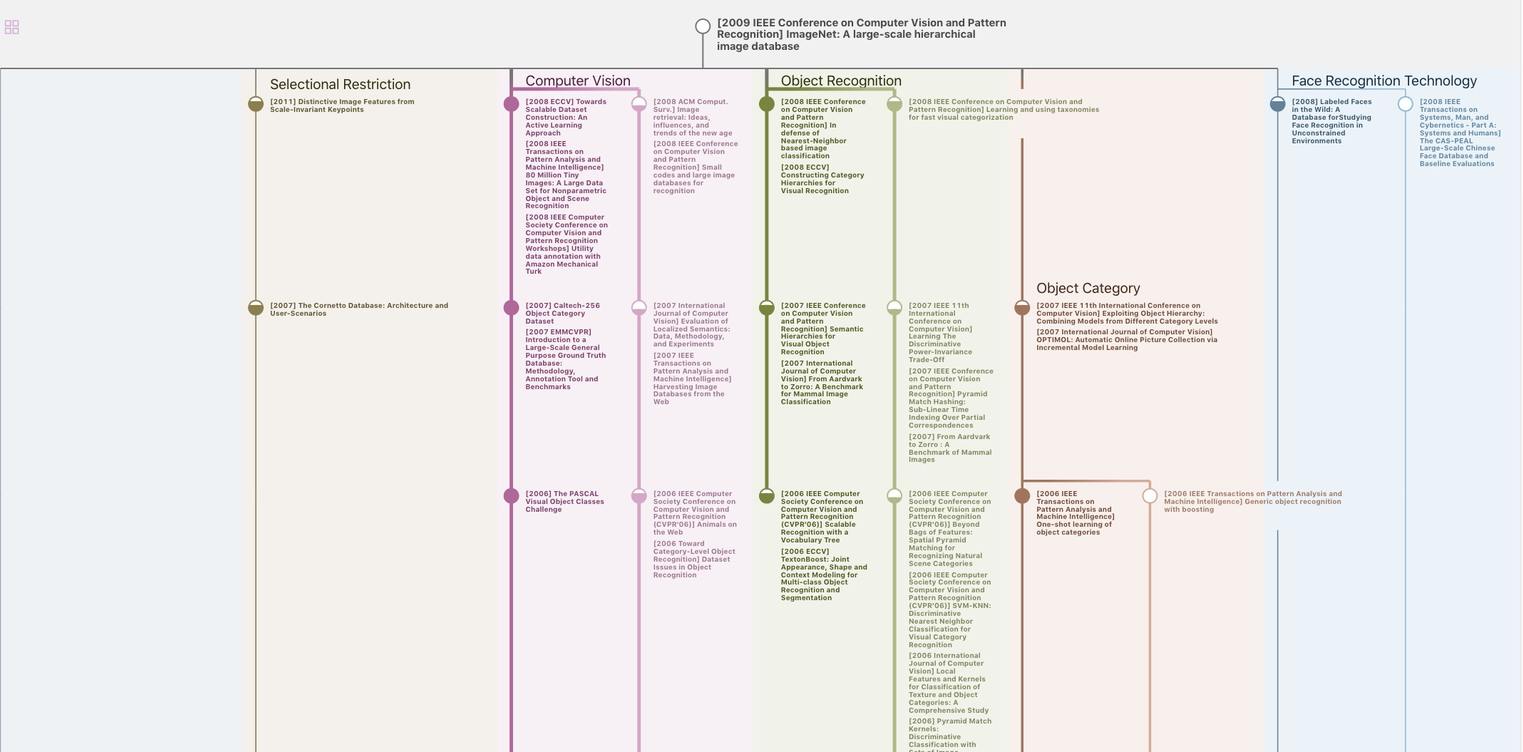
生成溯源树,研究论文发展脉络
Chat Paper
正在生成论文摘要