SeqGen: A Sequence Generator via User Side Information for Behavior Sparsity in Recommendation
PROCEEDINGS OF THE 32ND ACM INTERNATIONAL CONFERENCE ON INFORMATION AND KNOWLEDGE MANAGEMENT, CIKM 2023(2023)
摘要
In real-world industrial advertising systems, user behavior sparsity is a key issue that affects online recommendation performance. We observe that users with rich behaviors can obtain better recommendation results than those with sparse behaviors in a conversion-rate (CVR) prediction model. Inspired by this phenomenon, we propose a new method SeqGen, in an effort to exploit user side information to bridge the gap between rich and sparse behaviors. SeqGen is a learnable and pluggable module, which can be easily integrated into any CVR model and no longer requires two-stage training as in previous works. In particular, SeqGen learns a mapping relationship between the user side information and behavior sequences, only on the basis of the users with long behavior sequences. After that, SeqGen can generate rich sequence features for users with sparse behaviors based on their side information, so as to alleviate the issue of user behavior sparsity. The generated sequence features will then be fed into the classifier tower of an arbitrary CVR model together with the original sequence features. To the best of our knowledge, our approach constitutes the first attempt to exploit user side information for addressing the user behavior sparsity issue. We validate the effectiveness of SeqGen on the publicly available dataset MovieLens-1M, and our method receives an improvement of up to 0.5% in terms of the AUC score. More importantly, we successfully deploy SeqGen in the commercial advertising system Xlight of Alipay, which improves the grouped AUC of the CVR model by 0.6% and brings a boost of 0.49% in terms of the conversion rate on A/B testing.
更多查看译文
关键词
sequence generation,user side information,behavior sparsity,recommendation system
AI 理解论文
溯源树
样例
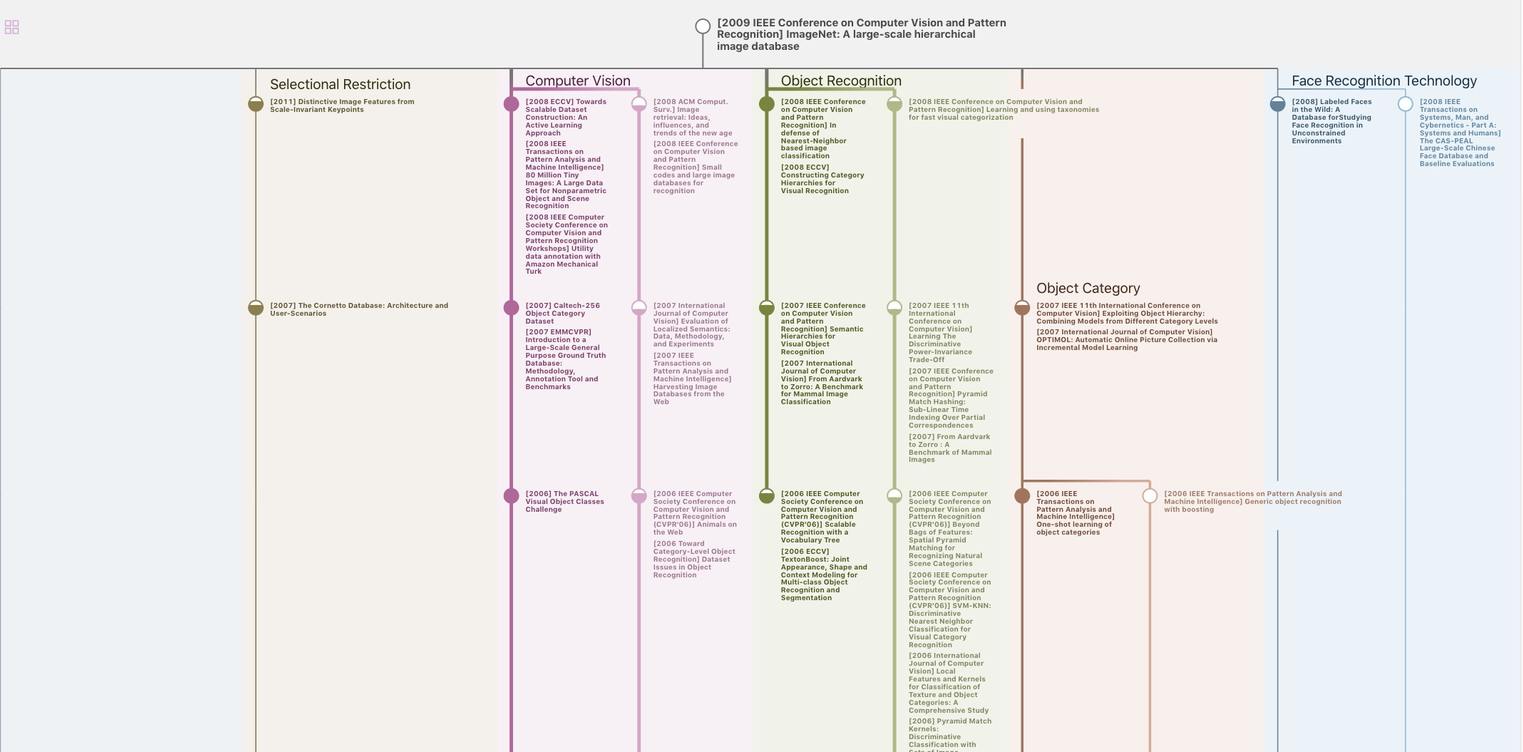
生成溯源树,研究论文发展脉络
Chat Paper
正在生成论文摘要