A Self-Learning Resource-Efficient Re-Ranking Method for Clinical Trials Search
PROCEEDINGS OF THE 32ND ACM INTERNATIONAL CONFERENCE ON INFORMATION AND KNOWLEDGE MANAGEMENT, CIKM 2023(2023)
摘要
Complex search scenarios, such as those in biomedical settings, can be challenging. One such scenario is matching a patient's profile to relevant clinical trials. There are multiple criteria that should match for a document (clinical trial) to be considered relevant to a query (patient's profile represented with an admission note). While different neural ranking methods have been proposed for searching clinical trials, resource-efficient approaches to ranker training are less studied. A resource-efficient method uses training data in moderation. We propose a self-learning reranking method that achieves results comparable to those of more complicated, fully supervised, systems. Our experiments demonstrate our method's robustness and competitiveness compared to the state-of-the-art approaches in clinical trial search.
更多查看译文
关键词
Language Model,Clinical trials search,Medical information retrieval,Learning-to-rank,Evidence-based medicine
AI 理解论文
溯源树
样例
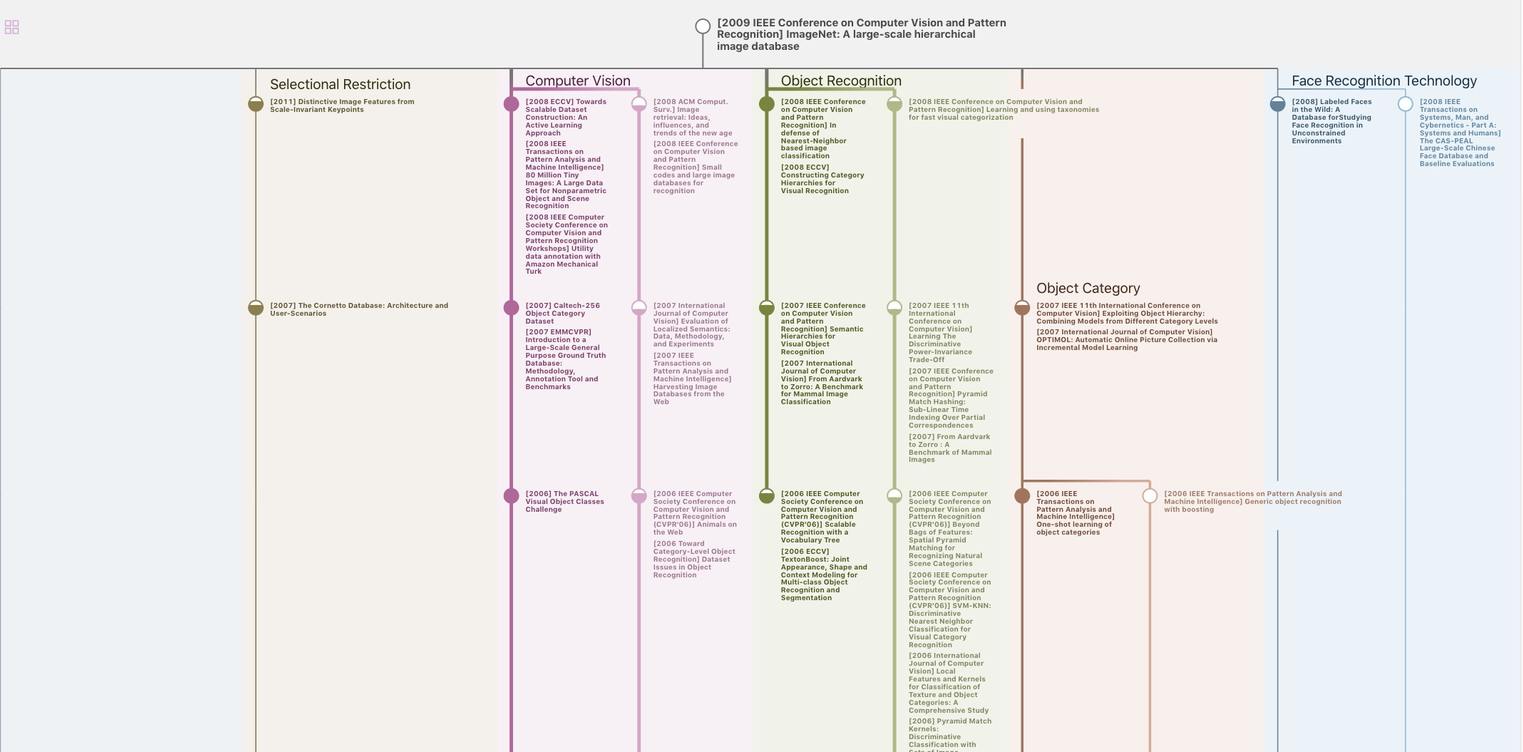
生成溯源树,研究论文发展脉络
Chat Paper
正在生成论文摘要